| Front page | | Contents | | Previous | | Next |
Prioritisation within The Integrated Product Policy
2 Methodology
2.1 Introduction
2.2 National accounting input-output tables (IO-tables)
2.3 Limitations of IO-tables for environmental analysis
2.4 Including unclassified imports
2.4.1 Tourism expenditures
2.5 Adding more environmental exchanges
2.5.1 Validating the completeness of the toxicity assessment
2.5.2 Data sources for the emissions included in the extended NAMEA
2.5.3 Emissions in the official Danish NAMEA
2.5.4 Internal work file from SSF-project (Pedersen 2003)
2.5.5 Internal work file from lcafood.dk (Dalgaard & Halberg 2004)
2.5.6 Point sources for N and P (Laursen et al. 2000)
2.5.7 Mass flow analyses
2.5.8 Ozone depleting substances (ODP)
2.5.9 Particles (PM10)
2.5.10 Pesticides
2.5.11 Land use (AIS)
2.5.12 Missing use stage emissions
2.6 Adjusting the level of aggregation of industries
2.7 Adjusting the level of aggregation of consumption
2.7.1 Accounting for investments and FISIM
2.7.2 Combining products in the use stage
2.8 Improving the modelling for imported products
2.9 Adjusting for market constraints
2.10 Impact assessment
2.10.1 Characterisation factors
2.10.2 New impact category: nature occupation
2.10.3 Normalisation
2.10.4 Weighting
2.10.5 Limitations of the impact assessment
2.11 Uncertainty analysis
2.11.1 Uncertainty due to aggregation in the Danish IO-table
2.11.2 Importance of aggregation level across national IO-tables
2.11.3 Geographical variation in inputs to industries
2.11.4 Aggregation vs. geographical uncertainty
2.11.5 Emission factor uncertainty for Danish emissions
2.11.6 Emission factor uncertainty for foreign emissions
2.12 Adjusting for differences in improvement options
2.13 Calculation routines and validation
2.1 Introduction
The main objective of the project was to establish and apply a method for prioritising product areas and product groups where Danish measures will give the largest improvement for the environment. The
method is based on environmentally extended Input-Output tables, also known as NAMEAs (National accounting matrices including environmental accounts). This chapter outlines the methodology applied,
and the critical methodological decisions made during the project.
2.2 National accounting input-output tables (IO-tables)
Input-output tables (or short: IO-tables) give an overview of the trade in national economies. The IO-tables are based on the reports on bought and sold products, which the national statistical bureau (in
Denmark: Statistics Denmark) receives from the individual industries. IO-tables report the monetary value of the products sold and bought within each sector of the economy.
For illustration of the principles, Table 2.1 shows a simplified IO-table for Denmark with 6 production industries. The full IO-table for Denmark has 130 industries and 107 different types of final use and is
published every year by Statistics Denmark.
Table 2.1: A simplified input-output table for Denmark, 1975 (Lihn Jørgensen, 1982). All figures in 1*109 DKK.
To :
Supplied from: |
Input to production industries |
Final Use |
To- tal |
A- gri- cul- tu- re |
In- dus- try |
Buil- ding |
Tra- de |
Pri- va- te
ser- vi- ces |
Pub- lic
ser- vi- ces |
Pri- va- te
con- sump- tion |
Pub- lic
con- sump- tion |
Gross- in- vest- ments |
Ex- ports |
Domestic prod. |
Agriculture |
3.0 |
15.7 |
0.4 |
0.0 |
0.1 |
0.2 |
2.1 |
- |
-0.6 |
3.1 |
24.0 |
Industry |
3.5 |
24.2 |
8.9 |
1.8 |
4.8 |
3.1 |
25.5 |
- |
4.5 |
41.4 |
117.7 |
Building |
0.4 |
0.8 |
0.0 |
0.8 |
4.3 |
1.9 |
- |
- |
26.9 |
- |
35.1 |
Trade |
1.5 |
3.9 |
1.8 |
0.4 |
1.7 |
1.0 |
20.2 |
- |
2.2 |
4.9 |
37.6 |
Private services |
1.2 |
5.7 |
3.4 |
4.0 |
8.5 |
7.0 |
40.1 |
- |
0.7 |
8.9 |
79.5 |
Public services |
0.0 |
0.2 |
0.0 |
0.1 |
0.3 |
0.1 |
2.1 |
53.2 |
- |
0.0 |
56.0 |
Import |
Agriculture |
0.3 |
6.4 |
0.0 |
0.0 |
0.1 |
0.1 |
0.9 |
- |
0.4 |
0.5 |
8.7 |
Industry |
2.8 |
18.8 |
4.0 |
0.7 |
2.6 |
1.9 |
10.7 |
- |
7.7 |
2.4 |
51.6 |
Building |
- |
- |
- |
- |
- |
- |
- |
- |
- |
- |
- |
Trade |
- |
- |
- |
- |
- |
- |
0.0 |
- |
0.0 |
0.0 |
0.0 |
Private services |
0.0 |
0.0 |
0.0 |
0.0 |
0.1 |
0.0 |
0.0 |
- |
0.0 |
0.0 |
0.1 |
Public services |
- |
- |
- |
- |
- |
- |
- |
- |
- |
- |
- |
Other import |
0.2 |
0.1 |
0.0 |
0.0 |
2.5 |
0.2 |
- 1.5 |
- |
0.1 |
5.2 |
6.8 |
Primary Factors |
Indirect taxes |
0.1 |
0.1 |
0.2 |
1.0 |
1.7 |
1.9 |
19.8 |
- |
3.2 |
- 1.5 |
26.5 |
Wages |
2.1 |
29.9 |
10.3 |
16.2 |
24.2 |
37.2 |
- |
- |
- |
- |
119.9 |
Other factorincome |
8.9 |
11.9 |
6.1 |
12.6 |
28.6 |
1.4 |
- |
- |
- |
- |
69.5 |
Total |
24.0 |
117.7 |
35.1 |
37.6 |
79.5 |
56.0 |
119.9 |
53.2 |
45.1 |
64.9 |
|
The rows show the total sales from each supply sector (divided in domestic production and import). The columns show the value of the input to each demand sector (the same domestic production sectors as
in the rows, plus some categories for final use). For example, Table 2.1 shows that Danish agriculture in 1975 sold goods worth 24 billion DKK in total. The majority of these goods were bought by Danish
industry, which purchased an amount worth 15.7 billion DKK. Of the remaining sales, 3.0 billion DKK were purchased by Danish colleagues within the agricultural sector, and products worth 3.1 billion
DKK were exported.
Since the production in one sector is based on inputs produced in all other sectors, and these again buy from all other sectors and so on, the IO-table can also be viewed as a very complete product life cycle
for all products in the national economy. This is illustrated in Figure 2.1.
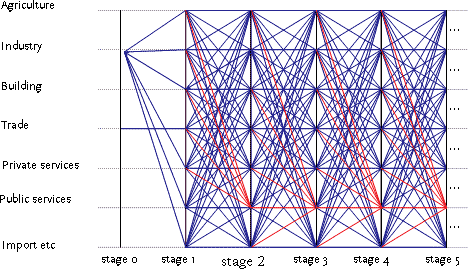
Figure 2.1: The direct demand for an industry product and the related, indirect demands for inputs from all industries. Figure based on Treloar (1998).
In Figure 2.1, starting from the purchase of a product worth 1 DKK from industry, the lines can be followed backwards (to the right in the figure) as it affects all other production sectors, which again affect
all other sectors, etc. Using IO-tables as a starting point for analysing interrelationships in an economy and the importance of different product groups is known as Input-Output Analysis (IOA).
When the IO-tables are supplemented with environmental data for each sector (resource consumption and emissions per DKK produced by each industry), the total environmental exchanges can be
calculated for those products from each sector that go into final use, i.e. the data that we need for prioritisation between product groups.
The name “Environmental IOA” is used when the IOA is applied to environmental issues, as in this project. As a “top-down” approach it allows a complete allocation of all activities to all products. IOA has
the advantage of being complete with regard to inclusion of all relevant activities related to a product. On the other hand, the IOA cannot deal with very specific questions, since it relies on a grouping of
activities in a limited number of industries. This makes it difficult to use for detailed studies, such as environmental product life cycle assessment (LCA), except for very homogenous industries. Also, the
necessary environmental statistics are not always available, which means that for some environmental exchanges, adequate information may be missing.
Instead, LCA has traditionally been performed as a “bottom-up” process analysis, based on linking the specific processes in a supply chain. A significant advantage of such process analysis is exactly its
capability for detail. However, a major problem in process-based LCA is the likelihood that important parts of the product systems are left out of the analysis, simply because it is a very difficult task to
follow the entire supply chain in detail (Lenzen 2001).
Combining process-based LCA and IOA in what has become known as “hybrid analysis” can yield a result that has the advantages of both methods (i.e. both detail and completeness).
For a project that is to prioritise among all product groups in an economy, the IOA approach is inherently better suited than LCA, but the results may still be improved by adding further detail via LCA-data
as suggested in Chapter 2.6.
IO-tables supplemented by environmental data are also known as NAMEAs (National accounting matrices including environmental accounts). NAMEAs can be produced when environmental data are
available for the same industries as in the IO-tables.
A Danish NAMEA is published annually by Statistics Denmark. The most recent NAMEA available, at the time of this project, was for the year 1999 (Danmarks Statistik 2003a), which was therefore
chosen as basis year for our further work.
2.3 Limitations of IO-tables for environmental analysis
To use IO-tables or NAMEAs as a basis for environmental analysis involves a number of limitations, some which are inherent to the methodology, and some have to do with data availability. Most of these
limitations may be overcome by adjusting and expanding the NAMEAs, as we have sought to do in this project.
In terms of data availability, the main limitation of the official Danish NAMEA is the coverage of environmental exchanges, which is limited to specific air emissions. We have added more environmental
exchanges, aiming for the same degree of completeness as in the normalisation reference for Denmark provided by the Danish LCA methodology EDIP (Hauschild & Wenzel 1998, Stranddorf et al. 2001).
This is described in detail in Chapter 2.5.
An important limitation is the assumption of homogeneity of the industries, i.e. that all products from an industry are assigned the same environmental impact per DKK. The higher the level of aggregation of
industries, and the more diverse the industry in question, the more erroneous this assumption will be. This is the main reason for attempting to subdivide such industries, as described in Chapter 2.6.
Some of the accounting conventions applied in the national accounts are also less appropriate for environmental IOA, and need therefore to be corrected, as described in Chapters 2.4, 2.6 and 2.7.
An important assumption of traditional IOA is that imported products are produced in the same way as the similar domestic products, although it is well-known that emission factors (e.g. CO2/DKK) can
vary significantly from country to country due to differences in geographic and administrative conditions, industries composition, applied technology, management systems and sizes of production units. For
example, in a traditional Input-Output analysis, the Danish textile industry's purchase of cotton will be treated as if the cotton was produced by Danish agriculture. The import assumption is especially
problematic in very open economies with large imports and exports, such as the Danish. A possible solution to the import assumption is to link the Danish IO-table to foreign IO-tables, thus obtaining a more
realistic picture. This solution is discussed in more detail in Chapter 2.8.
Further, using monetary IO-tables to represent physical flows of commodities between industries implies an assumption of proportionality of monetary and physical flows. For example, 100 DKK electricity
bought by the fertiliser industry is assumed to lead to equal amounts of electricity supplied as 100 DKK spent on electricity by travel agencies. However, electricity prices vary considerably amongst
industries, thus violating the proportionality assumption. The associated uncertainty can in principle be overcome by replacing the monetary entries in all basic IO-tables with entries in physical units.
However, such physical IO-tables are not produced on a regular basis in Denmark. Only for energy related air emissions, the NAMEA relates to physical flows of specific fuels based on the Danish energy
matrices, which are provided in both economic and physical units. In connection to the subdivision of industries, described in Chapter 2.6, we have sought to isolate physical product flows related to other
specific emissions, such as ozone depleting substances from refrigeration.
Finally, using retrospective IO-tables to answer prospective questions like the one we pose with this project: “What environmental measures will give the largest reductions in environmental impacts?”, may
lead to wrong results, since some of the processes included in the IO-table may not be able to change in response to market-based environmental measures. This problem can be overcome by adjusting the
input-output relations to reflect the actual prospective market reactions, as described in Chapter 2.9.
2.4 Including unclassified imports
In the Danish IO-table, a number of imported items are not distributed over the 130 industries, due to lack of information, and would therefore not enter into the analysis. We have therefore made our own
distribution of these imports:
- Unclassified transactions concerning oil activities in the North Sea (imports to industry 111000 Extraction of crude petroleum, natural gas etc. and 450003 Civil engineering) were distributed over the
foreign industries in proportion to the import already registered for the supplying industries to each of the two industries.
- Unclassified expenses of Danish ships abroad were distributed over foreign industries in proportion to the expenditure of the equivalent US-American industry (Water transport), except for fuel
bunkering, which was found specified in the Danish Supply-Use table.
- Tourism expenditure is a slightly more complicated issue, which we treat in the following sub-chapter. It should be noted that tourism revenues (the purchases made by foreigners visiting Denmark) are
included in the values for Danish consumption, thus resulting in a slight overestimate of Danish consumption. It would in principle be possible to deduct this consumption using the same data as for
tourism expenditure (purchases by Danes while travelling abroad). The size of tourism expenditure and tourism revenue is approximately the same, namely 26770 MDKK or 2.8% of the total Danish
consumption.
2.4.1 Tourism expenditures
In the IO-table, tourism expenditure is not classified by industry, in contrast to most other imports. The obvious reason for this is that the standard basis for the Danish IO-table does not provide any
information on what products are bought by Danes travelling abroad. In recent years there has been several initiatives to make up for this deficit by creating Tourism Satellite Accounts (TSA), notably the
convergence process of Eurostat, OECD and the World Tourism Organisation which led to a generally accepted framework in the context of the Statistical Commission of the United Nations (CEC et al.
2000). However, only few European countries have so far published such satellite accounts. Out of the countries that have a major share of Danish tourism, only Norway has published such an account
(Evensen 1999). Furthermore, even when such accounts were available, they would not distinguish between visitors from Denmark and other visitors. At the moment Statistics Denmark have no plans to
make a Danish TSA. Due to this lack of specific data (except for Norway), we have been forced to make some general assumptions on how the tourism expenditure is distributed over industries. These
general assumptions, shown in Table 2.2, have been derived in the following way:
- first, a distribution over the general groups “food, excl. restaurants” (8.5% for tourists and 1% for business travellers), “tobacco” (0.5% for tourists), “shopping” (12.5%), “accommodation” (industry
551000; 15% for tourists and 28% for business travellers), “restaurants” (industry 553000; 10% for tourists and 15% for business travellers), “motor vehicle driving” , “transport services” (35% for
tourists and 30% for business travellers), “tuition fees” (industry 803000; 3% of tourist expenditure), “conference fees” (industry 910000; 0.2% for tourists and 0.4% for business travellers), and
“recreation” (industry 920001; 6% for tourists and 2% for business travellers) is made, based on data from the available TSAs from Australia (ABS 2003), Canada (Delisle & Venne 2001), Norway
(Evensen 1999) and USA (Kass & Okubo 2000),
- “food” and “tobacco” is then distributed over the supplying industries (industries 11000, 50000, 150000 and 160000), in the same proportion as in the Danish private consumption,
- “shopping” is then distributed over the remaining industries producing small items typically consumed on the spot or as souvenirs in the same proportion as in the Danish imports,
- “motor vehicle driving” is split between “gasoline” (industry 230000; 6% for tourists and 8% for business travellers), “repair and maintenance of motor vehicles” (industry 502000; 0.5% for tourists
and 0.3% for business travellers) and “renting of vehicles” (industry 710000; 2.1%),
- “transport services” are split between railways (1%), other land transport (0.4 and 0.2%), taxis etc. (2 and 3%), water transport (5 and 1%), air transport (15 and 20%), and package tours (12 and
5%), the last figure indicating the percentage estimated for business travellers.
Table 2.2. Estimated distribution on industries of the expenditure of Danes travelling abroad (DK tourism imports)
|
Industry |
% of overall spending |
|
|
|
Business |
Tourist |
Notes |
11009 |
Agriculture |
0.04 |
0.32 |
1 |
11209 |
Horticulture, orchards etc. |
0.09 |
0.84 |
1 |
50000 |
Fishing |
0.00 |
0.03 |
1 |
151000 |
Production etc. of meat and meat products |
0.18 |
1.65 |
1 |
152000 |
Processing etc. of fish and fish products |
0.01 |
0.11 |
1 |
153000 |
Processing etc. of fruit and vegetables |
0.09 |
0.83 |
1 |
154000 |
Mfr. of vegetable and animal oils and fats |
0.01 |
0.05 |
1 |
155000 |
Mfr. of dairy products |
0.14 |
1.28 |
1 |
156009 |
Mfr. of starch, chocolate and sugar products |
0.14 |
1.30 |
1 |
158109 |
Mfr. of bread, cakes and biscuits |
0.06 |
0.58 |
1 |
158120 |
Bakers' shops |
0.07 |
0.64 |
1 |
158300 |
Manufacture of sugar |
0.00 |
0.02 |
1 |
159000 |
Mfr. of beverages |
0.12 |
1.04 |
1 |
160000 |
Manufacture of tobacco products |
0.00 |
0.50 |
2 |
170000 |
Mfr. of textiles and textile products |
0.00 |
3.27 |
3 |
180000 |
Mfr. of wearing apparel; dressing etc. of fur |
0.00 |
4.08 |
3 |
190000 |
Mfr. of leather and leather products |
0.00 |
1.11 |
3 |
221009 |
Publishing activities |
0.00 |
0.45 |
3 |
222009 |
Printing activities etc. |
0.00 |
0.90 |
3 |
230000 |
Refined petroleum products etc. (gasoline/diesel) |
7.50 |
6.00 |
4 |
261126 |
Mfr. of glass and ceramic goods etc. |
0.00 |
0.85 |
3 |
362060 |
Mfr. of toys, gold and silver articles etc. |
0.00 |
1.27 |
3 |
502000 |
Repair and maintenance of motor vehicles |
0.20 |
0.50 |
4 |
551009 |
Hotels etc. |
28.00 |
15.00 |
5 |
553009 |
Restaurants and other catering |
18.00 |
10.00 |
5 |
601000 |
Transport via railways |
1.00 |
1.00 |
6 |
602100 |
Other scheduled passenger land transport |
0.20 |
0.40 |
6 |
602223 |
Taxi operation and coach services |
3.00 |
2.00 |
6 |
610000 |
Transport by ship |
1.00 |
5.00 |
6 |
620000 |
Air transport |
28.00 |
15.00 |
6 |
631130 |
Travel agencies (package tours etc.) |
5.00 |
12.00 |
6 |
640000 |
Post and telecommunications |
0.64 |
0.64 |
3 |
710000 |
Renting of vehicles etc. |
4.10 |
2.10 |
4 |
803000 |
Higher education (tuition fees) |
0.00 |
3.00 |
7 |
910000 |
Membership organizations (conference fees etc.) |
0.40 |
0.20 |
7 |
920001 |
Recreational, cultural, sporting activities (market) |
2.00 |
6.00 |
6 |
|
100.00 |
100.00 |
|
1) The distribution is in proportion to the share in Danish private consumption, but much smaller (1/9) for business travellers.
2) Somewhat more than the share in Danish private consumption, as some carry-home is assumed. Business travellers' purchase is included as tourist expenditure, since employers do not refund this.
3) The distribution is in proportion to the share in Danish imports. Business travellers' shopping is included as tourist expenditure, since the employer does not refund such purchases, except for
telecommunication.
4) The relatively high figures are founded in an assumption that travels in Germany, Sweden and Norway are often by (own) car; business cars less likely to need repair.
5) Business travellers are expected to spend significantly more on accommodation and restaurants.
6) Business travellers use more taxi and air transport, while transport by ship and package tours are primarily for leisure.
7) The expenditure of students studying abroad is included under “tourists”. Some conference fees are also expected to be paid by students and other non-business travellers.
The emissions from tourism enter the calculation via the consumption of the listed items. The only exception is the emissions from fuel combustion from car driving, which are not included in the data for fuel
purchase. Therefore, we have separately added the emissions from car driving abroad, see Chapter 2.5.12.
2.5 Adding more environmental exchanges
To enable a more complete environmental assessment of product groups, we have extended the coverage of the official Danish NAMEA to more environmental exchanges.
The aim has been to include all exchanges that contribute significantly (more than 1.5%) to the normalisation reference for Denmark provided by the Danish LCA methodology EDIP. This normalisation
reference is an inventory of the total Danish emissions contributing to the EDIP impact categories, i.e. the impact categories used in LCA studies (see also Chapter 2.10). The original EDIP normalisation
reference for 1990 (Hauschild & Wenzel 1998) was updated to 1994 by Stranddorf et al. (2001). The latter was not published at the time of this project, but was available to us as a manuscript. Table 2.3
provides an overview of the substances contributing to the 1994 normalisation reference.
All the substances mentioned in Table 2.3 have been included in the expanded NAMEA, except for the following four substances:
- Benzene to water, as we found that the contribution via water is unlikely to be more important than the contribution via air, which is not included as separately important.
- Arsenic to soil (via sludge) as this stems mainly from old applications and not the currently used products. Furthermore, it would be very difficult to trace this contribution to specific products.
- PAH to water, as we have been unable to find any reliable Danish statistics for this. The value in Stranddorf et al. (2001) is an extrapolation from Dutch data. The emissions are mainly believed to
come from road runoff and occasional fuel spillage and fuel purchase could therefore be used as a key for distribution over industries. However, as no reliable source of data for the total value for
Denmark in 1999 is available, we have refrained from this. This implies a minor underestimation of road transport and products involving much road transport.
- Tetrachlorethylene use in Denmark has generally been phased out except for minor uses as a degreasing agent. The Danish supply-use table indicates use in the industry “Refuse collection and
sanitation”, but we have been unable to verify this with industry sources, and have therefore decided to ascribe this to a misallocation in the statistics.
Table 2.3. Substances contributing to the 1994 normalisation reference (from Stranddorf et al. 2001)
Percentages |
|
Impact categorya |
Exchange: |
to |
gw |
od |
ac |
ne |
po |
etwc |
etwa |
etsc |
hta |
htw |
hts |
CO2 |
air |
60 |
|
|
|
|
|
|
|
|
|
|
Methane |
air |
22 |
|
|
|
3 |
|
|
|
|
|
|
CO |
air |
5 |
|
|
|
|
|
|
|
5 |
|
|
SO2 |
air |
|
|
30 |
|
|
|
|
|
2 |
|
|
NOx |
air |
|
|
37 |
24 |
|
|
|
|
22 |
|
|
Ammonia |
air |
|
|
33 |
23 |
|
|
|
|
|
|
|
N2O |
air |
6 |
|
|
|
28 |
|
|
|
|
|
|
N-tot |
water |
|
|
|
34 |
|
|
|
|
|
|
|
NMVOC |
air |
|
|
|
|
70 |
|
|
|
14 |
|
|
Benzene |
water |
|
|
|
|
|
|
1b |
|
7b |
|
|
As |
air |
|
|
|
|
|
|
|
|
|
|
9 |
As |
soil |
|
|
|
|
|
|
|
|
|
|
8b |
Cd |
air |
|
|
|
|
|
1 |
|
|
1 |
|
|
Cd |
water |
|
|
|
|
|
3 |
3 |
|
|
|
|
Cu |
air |
|
|
|
|
|
1 |
|
|
|
|
|
Cu |
water |
|
|
|
|
|
14 |
14 |
|
|
|
|
Hg |
air |
|
|
|
|
|
1 |
|
|
|
90 |
75 |
Hg |
water |
|
|
|
|
|
|
|
|
|
9 |
|
Hg |
soil |
|
|
|
|
|
|
|
|
|
|
3 |
Pb |
air |
|
|
|
|
|
|
|
|
37 |
|
|
Tributyltinoxide |
water |
|
|
|
|
|
73 |
74 |
|
|
|
|
Zn |
air |
|
|
|
|
|
|
|
|
|
|
|
Zn |
water |
|
|
|
|
|
3 |
3 |
|
|
|
|
ODP1 |
air |
8 |
100 |
|
|
|
|
|
|
|
|
|
PAH |
air |
|
|
|
|
|
|
|
|
2 |
|
|
PAH |
water |
|
|
|
|
|
3b |
3b |
|
|
|
|
PM10 |
air |
|
|
|
|
|
|
|
|
9 |
|
|
Pesticides |
soil |
|
|
|
|
|
|
|
99 |
|
|
|
PO43- |
water |
|
|
|
19 |
|
|
|
|
|
|
|
Tetrachlorethylene |
air |
|
|
|
|
|
|
|
|
|
|
2b |
|
100 |
100 |
100 |
100 |
100 |
99 |
98 |
99 |
99 |
99 |
97 |
a) gw = global warming, od = ozone depletion, ac = acidification, ne = nutrient enrichment, po = photchemical ozone formation, etwc = ecotoxicity water chronic, etwa = cotoxicity water acute, etsc = cotoxicity soil chronic, hta = human toxicity air, htw = human toxicity water, hts = human toxicity soil
b) Not included in the expanded NAMEA. See text for explanations.
2.5.1 Validating the completeness of the toxicity assessment
The normalisation data for toxicity from EDIP (Hauschild & Wenzel 1998, Stranddorf et al. 2001) does not claim to cover all important toxic substances. To assess the completeness reached by this
approach, we used the toxic release data from the U.S. Toxic Release Inventory (TRI), which covers a larger number of substances (see Suh 2003b for an assessment of the TRI coverage). The TRI 1998
data was scored using the available EDIP characterisation factors (Olsen 2003) to see whether some substances would appear as important, additional to the substances covered by the EDIP normalisation
reference. We identified an error in the published EDIP ecotoxicity characterisation factors for Malathion, which has now been corrected.
This procedure does not in itself provide a complete validation, since:
- there are toxic substances not included in TRI, which may still be of importance, and
- there are substances covered by TRI for which no EDIP toxicity factors are available. The coverage of EDIP is approximately 37% (230 out of 630 TRI substances).
Nevertheless, with the current data availability, this procedure is found to be the most adequate way to validate the completeness of the EDIP normalisation data for use in this project.
The results of the EDIP scoring of the TRI 1998 data can be seen in Tables 2.4-2.9.
Table 2.4. Top 10 TRI 1998 emissions contributing to the total EDIP score for human toxicity via air.
Italics indicate substances not specifically included in the present study.
Chemical |
Initial emission
compartment |
EDIP EP(hta);
m³ |
% of total |
Accumulated % |
1,3-butadiene |
air |
3.10E+17 |
33% |
33.04% |
NO2 |
air |
1.88E+17 |
20% |
53.08% |
N-butyl alcohol |
air |
1.08E+17 |
12% |
64.58% |
Formaldehyde |
air |
7.63E+16 |
8% |
72.71% |
CO |
air |
7.57E+16 |
8% |
80.77% |
PM10 |
air |
5.80E+16 |
6% |
86.96% |
Benzene |
air |
3.50E+16 |
4% |
90.69% |
PAH's |
air |
3.35E+16 |
4% |
94.26% |
SO2 |
air |
2.21E+16 |
2% |
96.61% |
Pb |
air |
1.52E+16 |
2% |
98.23% |
Of the top 10 TRI substances contributing to more than 98% of the total EDIP score for human toxicity via air (Table 2.4), four substances are not included in the expanded NAMEA from this project.
These substances (1,3-butadiene [3], n-butyl alcohol [4], formaldehyde [5] and benzene) are all volatile organic compounds. In principle, they are (or should be) already covered in the NAMEA category
NMVOC.
However, their human toxicity potentials (and the photochemical ozone creation potential for 1,3-butadiene) are much larger per kg than for the average NMVOC according to the Danish normalisation
reference (Stranddorf et al. 2001). We would therefore recommend the separate inclusion of these substances in the Danish NAMEA. However, there are no adequate overall Danish statistics on emissions
of these substances. It would be possible to include n-butyl alcohol and formaldehyde in the Danish NAMEA by applying emission factors from the FIRE data system (EPA 2000) or more simply by using
emission factors derived from the U.S. TRI database for the equivalent industries. Specifically for 1,3-butadiene, emission factors can be found in EPA (1996). Due to time constraints, we have not used
these procedures to include the said substances in the NAMEA in the current project.
However, a closer analysis reveals that the major source of 1,3-Butadiene and formaldehyde is exhaust emissions from gasoline- and diesel-powered vehicles. In fact, Drivsholm et al. (2002) show that
1,3-Butadiene, formaldehyde and benzene account for 89% of the NMVOC impact potential for human toxicity via air from diesel trucks. The resulting EDIP characterisation factor for NMVOC's from
diesel is 1.12*107 m³/g (Drivsholm et al. 2002), which is three orders of magnitude larger than the characterisation factor applied for the average NMVOC in the Danish normalisation reference (Stranddorf
et al. 2001). Since 42 % of all NMVOC's come from transport (Drivsholm et al. 2002, Annex B), this points to the NMVOC characterisation factor applied for the normalisation reference being too low.
We therefore recommend that a revised characterisation factor be calculated for the normalisation reference, which would lead to a larger contribution of NMVOC's to the human toxicity potential via air,
compared to the 14% in the current EDIP normalisation reference (Stranddorf et al. 2001).
Table 2.5. Top 10 TRI 1998 emissions contributing to the total EDIP score for human toxicity via water
Chemical |
Initial emission
compartment |
EDIP EP(htw);
m³ |
% of total |
Accumulated % |
Mercury |
soil |
1.18E+13 |
91% |
91.17% |
Mercury |
air |
1.08E+12 |
8% |
99.56% |
Hexane |
air |
1.04E+10 |
0% |
99.65% |
Lead |
air |
8.05E+09 |
0% |
99.71% |
Benzene |
air |
7.88E+09 |
0% |
99.77% |
Zinc |
air |
7.48E+09 |
0% |
99.83% |
Mercury |
water |
6.61E+09 |
0% |
99.88% |
Thallium |
air |
3.23E+09 |
0% |
99.90% |
Antimony |
water |
2.09E+09 |
0% |
99.92% |
Thallium |
water |
1.92E+09 |
0% |
99.93% |
The EDIP score for human toxicity via water (Table 2.5), is dominated by mercury emissions. All other substances are dwarfed in comparison. Nevertheless, out of the top 10 TRI substances, we find again
two VOC's: hexane and benzene. Also three heavy metals (zinc, thallium and antimony), which are not included in the expanded NAMEA from this project, show up. Recently, the Danish EPA has
published an assessment of these “second rank” heavy metals (Kjølholt et al. 2003), so some data are available if it was judged desirable to include these in the NAMEA. In the present project this has not
been done.
Table 2.6. Top 10 TRI 1998 emissions contributing to the total EDIP score for human toxicity via soil
Chemical |
Initial emission
compartment |
EDIP EP(hts);
m³ |
% of total |
Accumulated % |
Arsenic |
soil |
4.5E+11 |
82% |
81.84% |
Benzene |
air |
5.05E+10 |
9% |
91.03% |
Antimony |
soil |
1.21E+10 |
2% |
93.22% |
Chromium |
soil |
9.84E+09 |
2% |
95.01% |
Mercury |
soil |
8.82E+09 |
2% |
96.61% |
Manganese |
soil |
5.02E+09 |
1% |
97.53% |
Vinyl chloride |
air |
1.6E+09 |
0% |
97.82% |
Chlorine |
air |
1.41E+09 |
0% |
98.07% |
Propionaldehyde |
air |
9.68E+08 |
0% |
98.25% |
Silver |
soil |
9.67E+08 |
0% |
98.43% |
The EDIP score for human toxicity via soil (Table 2.6) is dominated [6] by arsenic emissions to soil. In the Danish normalisation reference for 1994, arsenic in sludge amounted to 8% of the total, while
mercury and arsenic emissions to air were dominating (Mercury and arsenic to air comes in as no. 11 and 22 in the TRI 1998 emissions). For this reason, only these two air emissions were included. The
result is that only one of the top 10 TRI substances (Hg to soil) is included in the expanded NAMEA from this project. The difference in results is mainly due to the very different industry composition
between Denmark and USA (Denmark does not have any primary metal extraction and manufacture, nor any production of vinyl chloride and chlorine). However, it cannot be excluded that soil emissions
were underreported in the data used for the Danish normalisation reference. We would therefore recommend revisiting the decision not to include heavy metal emissions to soil in the Danish NAMEA (data is
to some extent available from mass flow analyses), although the most important improvement would be to include the mentioned emissions (heavy metals to soil, vinyl chloride and chlorine to air) in the data
used for imported products (e.g. based on the TRI data). The VOC's (here benzene and propionaldehyde) have already been mentioned above under the other two human toxicity scores, and the
recommendation is here again to include these as separate substances or to recalculate the average NMVOC effect factor applied in the normalisation.
Table 2.7. Top 10 TRI 1998 emissions contributing to the total EDIP score for ecotoxicity water, chronic
Chemical |
Initial emission
compartment |
EDIP EP(ewc);
m³ |
% of total |
Accumulated % |
N-hexane |
air |
4.52E+12 |
47% |
46.52% |
PAH |
water |
1.1E+12 |
11% |
57.80% |
Copper |
air |
9.53E+11 |
10% |
67.60% |
Hydrogen cyanide |
air |
7.5E+11 |
8% |
75.32% |
Mercury |
soil |
4.34E+11 |
4% |
79.78% |
Zinc |
air |
3.62E+11 |
4% |
83.50% |
Copper |
water |
3.22E+11 |
3% |
86.81% |
Toluene |
air |
1.78E+11 |
2% |
88.64% |
Formaldehyde |
air |
1.46E+11 |
2% |
90.14% |
Xylene |
air |
1.24E+11 |
1% |
91.42% |
Table 2.8. Top 10 TRI 1998 emissions contributing to the total EDIP score for ecotoxicity water, acute
Chemical |
Initial emission
compartment |
EDIP EP(ewa);
m³ |
% of total |
Accumulated % |
PAH |
water |
1.1E+11 |
63% |
63.31% |
Copper |
water |
3.22E+10 |
19% |
81.86% |
Formaldehyde |
water |
8.06E+09 |
5% |
86.52% |
Manganese |
water |
6.61E+09 |
4% |
90.33% |
Anthracene |
water |
2.63E+09 |
2% |
91.85% |
Malathion |
water |
2.31E+09 |
1% |
93.18% |
Zinc |
water |
1.87E+09 |
1% |
94.26% |
Antimony |
water |
1.24E+09 |
1% |
94.97% |
Lead |
water |
1.2E+09 |
1% |
95.67% |
Chromium |
water |
1.16E+09 |
1% |
96.34% |
Table 2.9. Top 10 TRI 1998 emissions contributing to the total EDIP score for ecotoxicity soil, chronic
Chemical |
Initial emission
compartment |
EDIP EP(esc);
m³ |
% of total |
Accumulated % |
Hydrogen cyanide |
air |
7.11E+12 |
68% |
68.44% |
Formaldehyde |
air |
1.24E+12 |
12% |
80.34% |
Dimethoate |
soil |
9.17E+11 |
9% |
89.17% |
Hydrogen cyanide |
soil |
3.87E+11 |
4% |
92.89% |
Atrazine |
soil |
1.5E+11 |
1% |
94.34% |
Ethylene |
air |
1.22E+11 |
1% |
95.51% |
N-hexane |
air |
7.58E+10 |
1% |
96.24% |
Chloroform |
air |
7.24E+10 |
1% |
96.93% |
Silver |
soil |
6.07E+10 |
1% |
97.52% |
Toluene |
air |
4.31E+10 |
0% |
97.93% |
The ecotoxicity scores in the expanded NAMEA are all dominated by TBTO, an antifouling agent that is underreported in the TRI data. Besides this, heavy metals are the main contributors to the Danish
ecotoxicity scores, with the exception of soil ecotoxicity, where pesticides play a dominating role [7].
Again, the VOC's (here hexane, toluene, formaldehyde and xylene) contribute to the EDIP score of the TRI emissions, especially to ecotoxicity water, chronic.
Other major contributions to ecotoxicity that were not initially included in the expanded NAMEA of this project are: PAH emissions to water and zinc and hydrogen cyanide to air. Zinc emissions to air are
readily available in the dataset provided by Pedersen (2003) and have therefore been added to the expanded NAMEA in this project. For the two other substances, it would be possible to apply emission
factors derived from the U.S. TRI database, but this has not been done in this project. Hydrogen cyanide is mainly emitted from biomass incineration, but emission factors are not readily available. PAH
emissions to water are mainly believed to come from road runoff and occasional fuel spillage, but no Danish statistics are available. Fuel purchase could be used as key for distribution over industries.
Denmark is the globally leading producing country for malathion, which implies that these emissions would be included if a complete life cycle perspective on Danish products were applied, i.e. including the
use stage of exported products. Of all malathion used, 2/3 is applied to cotton crops, implying that it is mainly part of the life cycle of textiles. We have therefore included this application specifically.
We have also assessed the importance of detergents, which are not recorded in the TRI data, but based on the data in Hauschild & Wenzel (1998) and Stranddorf et al. (2001) we have found that the
contribution to the overall ecotoxicity is insignificant.
In conclusion:
- All toxicity characterisation factors for unspecified VOC's are underestimated in the EDIP method applied in this project, especially affecting human toxicity via air and chronic water ecotoxicity.
- Emissions to soil (except Hg) are excluded from the expanded NAMEA, which gives a bias to the results for human toxicity via soil, which are dominated by mercury and arsenic emissions to air.
- PAH to water, which is not included in the expanded NAMEA of this project, is a significant contributor to water ecotoxicity.
The identified data gaps will affect the results of the current project in the following way:
- Land transport and products involving much land transport will be underestimated due to the low and missing toxicity scores for VOC's and PAH to water.
- Products with steel components and high electricity use (which are the two main contributors to human toxicity via soil, when using the current expanded NAMEA) will be overestimated with respect
to human toxicity via soil, while products with non-ferrous metal components will be underestimated, since the major sources to TRI human toxicity via soil are the non-ferrous metal producing
industries.
A summary of the above recommendations is to:
- Recalculate the EDIP characterisation factor for NMVOC for human toxicity via air, based on a speciation of the VOC's in major sources (or preferably to include the specific VOC's with their
specific characterisation factors).
- Include heavy metals to soil, vinyl chloride and chlorine to air in the data used for imported products, based on the TRI data, while considering also data sources for Danish emissions to soil.
- Include PAH emissions to water and hydrogen cyanide to air, based on the TRI data, while considering also other data sources.
2.5.2 Data sources for the emissions included in the extended NAMEA
Emissions were estimated using a variety of sources, shown in table 2.10. Some data were further processed, as explained in the following sub-chapters.
Table 2.10. List of emissions covered by the expanded NAMEA and their main sources.
Exchange |
Compartment |
Main data source |
CO2 |
air |
Official Danish NAMEA |
Methane |
air |
Official Danish NAMEA, Dalgaard & Halberg 2004 |
CO |
air |
Official Danish NAMEA |
SO2 |
air |
Official Danish NAMEA |
NOx |
air |
Official Danish NAMEA |
Ammonia |
air |
Official Danish NAMEA |
Land use |
non material |
AIS (Madsen 2003), Dalgaard & Halberg 2004 |
N2O |
air |
Official Danish NAMEA, Dalgaard & Halberg 2004 |
N-tot |
water |
Dalgaard & Halberg 2004 and Laursen et al. (2000) |
Non methane VOC |
air |
Official Danish NAMEA |
As |
air |
Pedersen 2003 |
Cd |
air |
Pedersen 2003 |
Cd |
water |
Substance flow analyses (Drivsholm et al. 2000) |
Cu |
air |
Pedersen 2003 |
Cu |
water |
Substance flow analyses (Lassen et al. 1996) |
Hg |
air |
Pedersen 2003 |
Hg |
water |
Substance flow analyses (Skårup al. 2003) |
Hg |
soil |
Substance flow analyses (Skårup al. 2003) |
Ni |
air |
Pedersen 2003 |
Pb |
air |
Pedersen 2003 |
Pb |
water |
Substance flow analyses (Lassen et al. 2003) |
Tributyltinoxide |
water |
Substance flow analyses (Lassen et al. 1997) |
Zn |
air |
Pedersen 2003 |
Zn |
water |
Substance flow analyses (Hansen 1995b) |
ODP |
air |
Poulsen 2001 |
PAH |
air |
Pedersen 2003 |
Particulates (PM10) |
air |
NERI 2003 |
Pesticides |
soil |
DEPA 2000 and Suh 2003a |
Phosphorous (PO43-) |
water |
Dalgaard & Halberg 2004 |
2.5.3 Emissions in the official Danish NAMEA
The official Danish NAMEA (Danmarks Statistik 2003a) covers both energy related emissions and non-energy related emissions of CO2, SO2, NOx, NH3, NMVOC, CO, CH4, and N2O.
The accounting conventions for emissions are not always the same as those typically applied in LCA, for example CO2 emissions are included from cement manufacture and from biomass combustion, even
though there is no credit included for CO2 uptake during concrete hardening and during plant growth. To avoid introducing alternative arbitrary conventions and unnecessary adjustments, we have generally
not adjusted the data, also because the effects of these conventions on the prioritisation are relatively small. However, when the data are used for other purposes than strategic prioritisation, these
conventions may lead to an unwanted bias, of which the user should be aware and take the appropriate measures to adjust for.
In one specific case we have adjusted the emissions reported in the official Danish NAMEA: Emission of NMVOC from Danish forestry has been deleted. These emissions (14190 Mg) were mainly due
to coniferous forests and gave forest products a very prominent position in the prioritisation. However, if the alternative to coniferous forest is a natural forest with a high degree of oak-trees, the NMVOC
emissions could be in the same order of magnitude (Fenhann 1999). Therefore, cultivation of forests has no net impact on the NMVOC emission.
In parallel, it could be argued that other area-occupying activities are in fact suppressing the emission of natural NMVOC (and other emissions from natural woodlands, which is the alternative climax
vegetation) and should therefore be assigned this effect as a negative emission. However, the knowledge on NMVOC emissions from agricultural plant growth is still sparse, which would make such
corrections highly speculative.
Most emissions in the official Danish NAMEA are allocated to the 130 industries of the IO-table or to final use. However, five sources of emissions are reported as undistributed:
- Undistributed emissions from production industries
- Distribution of fossil energy
- Wastewater treatment and dumps
- Nature
- Solvents
Most of the undistributed emissions from production industries are negligible and we have therefore not taken them into account. We have allocated the undistributed VOC-emissions to beer brewing, based
on Illerup et al. (2002).
For the undistributed emissions from distribution of fossil energy we have allocated the methane to manufacture and production of gas (industry 402000) and the non-methane VOC with 3/4 for fuel
distribution and 1/4 for gas distribution, based on rough estimates of the involved quantities of fuel and gas and the corresponding CORINAIR emission factors (EEA 2003).
For wastewater treatment and dumps, the undistributed emissions concern methane, which is a result of previous landfilling of biomass, an activity that no longer takes place. These emissions have therefore
been left out.
Emissions from nature are considered background emissions, and thus not included.
For the undistributed emissions of solvents, we have used the amounts reported in Illerup et al. (2002) for fat extraction and car maintenance. We have distributed the remaining amount on the basis of a
solvent mass flow analysis using the data from the Danish physical supply-use table (Danmarks Statistik 2003b). The mass flow analysis covers the commodities mentioned in Table 2.11. Solvents included in
imported products have also been accounted for, mainly in wood preservation agents.
Table 2.11. Solvents covered by mass flow analysis
Commodity no. |
Commodity name |
V290301 |
Chlormethane, chlorethane, etc. |
V290303 |
Dichlormethane, saturated |
V290305 |
Chloroform, saturated |
V290307 |
Trichlorethylene, unsaturated |
V290309 |
Tetrachlorethylen, unsaturated |
V291401 |
Acetone |
V291403 |
Butanone (ethylmethylketone) |
V271025 |
Turpentine, mineral |
V220700 |
Ethanol |
The total amount of these commodities is approx. 46 Gg. Subtracting 7 Gg used in pharmaceuticals (assumed to be metabolised or combusted), the remaining 39 Gg are traced either to final use (household
& public use), use in industry or incorporation in products from the industries “Mfr. of detergents and other chemical products” and “Mfr. of paints, printing ink and mastics”. The solvent-containing products
of these two industries are again traced either to final use (household & public use) or use in industry, applying the estimated emission factors given in Table 2.12 and taking into account that part of the
solvents (approx. 4.5 Gg) are exported (mainly in products from the paint industry). Since the amounts for fat extraction and car maintenance make up 1 and 4.5 Gg, respectively, the total amount accounted
for in this way is 40 Gg, which is close to the 38.538 Gg undistributed emissions reported in the emission matrices of Statistics Denmark (Danmarks Statistik 2003a). Some of the industrially applied solvents
are collected and combusted and may account for the difference. In view of the uncertainty on the data, we have chosen to distribute the entire 40 Gg on the industries and final use. In fact, the estimated
emission factors in Table 2.12 are lower than many of the default values recommended for national emission inventories, which implies that the total value of 40 Gg for Denmark may be underestimated. The
distribution resulting from our mass flow analysis is shown in Table 2.13.
Table 2.12. Approximate average emission factors (solvent contents) for product groups. Own unverified estimates.
Commodity
no. |
Commodity name |
Emission
factor
(solvent
content) |
V320800 |
Paint & laquer, non-waterbased |
15.0% |
V320900 |
Paint & laquer, waterbased |
1.0% |
V321000 |
Laquer & paint of drying oils, glue |
5.0% |
V321400 |
Putty etc. |
2.0% |
V321501 |
Printing inks |
10.0% |
V321503 |
Writing inks etc. |
10.0% |
V330101 |
Citrus oils |
30.0% |
V330103 |
Concentrated etheric oils |
30.0% |
V330200 |
Mixtures of odorants |
3.0% |
V330300 |
Perfumes |
6.0% |
V330500 |
Hair care agents |
0.3% |
V330701 |
Perfumes, cosmetics, toiletries |
3.0% |
V330703 |
Odorants |
3.0% |
V340203 |
Prepared cleaning agents |
0.3% |
V340400 |
Synthetic wax |
3.0% |
V340501 |
Shoe polish |
3.0% |
V340503 |
Polish for wood and automobiles |
6.0% |
V340507 |
Polish for metals |
6.0% |
V350600 |
Prepared glues |
3.0% |
V381400 |
Solvents and dilutants |
10.0% |
V382000 |
Antifreeze preparations |
3.0% |
Table 2.13. Resulting distribution of solvent emissions.
Group
code |
Group name |
NMVOC
from solvent
use (Mg) |
11009 |
Agriculture |
59 |
11209 |
Horticulture, orchards etc. |
3 |
14002 |
Public landscape gardeners (stadsgartnere) |
17 |
151000 |
Production etc. of meat and meat products |
1 |
153000 |
Processing etc. of fruit and vegetables |
6 |
154000 |
Mfr. of vegetable and animal oils and fats |
1021 |
155000 |
Mfr. of dairy products |
11 |
156009 |
Mfr. of starch, chocolate and sugar products |
159 |
158109 |
Mfr. of bread, cakes and biscuits |
11 |
158120 |
Bakers' shops |
1 |
159000 |
Mfr. of beverages |
9 |
160000 |
Manufacture of tobacco products |
10 |
170000 |
Mfr. of textiles and textile products |
25 |
190000 |
Mfr. of leather and leather products |
24 |
200000 |
Mfr. of wood and wood products |
1176 |
210000 |
Mfr. of pulp, paper and paper products |
385 |
221200 |
Publishing of newspapers |
6 |
221309 |
Publishing activities, excluding newspapers |
332 |
222009 |
Printing activities etc. |
765 |
230000 |
Mfr. of refined petroleum products etc. |
26 |
241209 |
Mfr. of dyes, pigments and organic basic chemicals |
60 |
241500 |
Manufacture of fertilisers etc. |
547 |
241617 |
Mfr. of plastics and syntethic rubber |
1444 |
242000 |
Manufacture of pesticides and other agro-chemical products |
16 |
243000 |
Mfr. of paints, printing ink and mastics |
136 |
244000 |
Mfr. of pharmaceuticals etc. |
89 |
245070 |
Mfr. of detergents and other chemical products |
99 |
251122 |
Mfr. of rubber products and plastic packing goods etc. |
918 |
252300 |
Mfr. of builders' ware of plastic |
82 |
252400 |
Manufacture of other plastic products n.e.c. |
138 |
261126 |
Mfr. of glass and ceramic goods etc. |
332 |
266080 |
Mfr. of concrete, cement, asphalt and rockwool products |
370 |
271000 |
Mfr. of basic ferrous metals |
5 |
272030 |
First processing of iron and steel |
410 |
274000 |
Mfr. of basic non-ferrous metals |
122 |
275000 |
Casting of metal products |
84 |
281009 |
Mfr. of construct. materials of metal etc. |
1056 |
286009 |
Mfr. of hand tools, metal packaging etc. |
955 |
291000 |
Mfr. af marine engines, compressors etc. |
149 |
292000 |
Mfr. of other general purpose machinery |
391 |
293000 |
Mfr. of agricultural and forestry machinery |
246 |
294009 |
Mfr. of machinery for industries etc. |
137 |
297000 |
Mfr. of domestic appliances n.e.c. |
168 |
300000 |
Mfr. of office machinery and computers |
13 |
310000 |
Mfr. of other electrical machinery and apparatus |
654 |
320000 |
Mfr. of radio and communicat. equipm. etc. |
155 |
330000 |
Mfr. of medical and optical instrum. etc. |
14 |
340000 |
Manufacture of motor vehicles etc. |
534 |
351000 |
Building and repairing of ships and boats |
410 |
352050 |
Mfr. of transport equipment excl. ships, motor vehicles etc. |
34 |
361000 |
Mfr. of furniture |
745 |
362060 |
Mfr. of toys, gold and silver articles etc. |
49 |
450001 |
Construction of new buildings |
3261 |
450002 |
Repair and maintenance of buildings |
2620 |
450003 |
Civil engineering |
299 |
450004 |
Construction materials |
7239 |
501009 |
Sale of motor vehicles, motorcycles etc. |
45 |
502000 |
Repair and maintenance of motor vehicles |
4880 |
505000 |
Service stations |
8 |
521090 |
Retail trade of food etc. |
33 |
524490 |
Other retail sale, repair work |
16 |
551009 |
Hotels etc. |
3 |
553009 |
Restaurants and other catering |
12 |
601000 |
Transport via railways |
278 |
602100 |
Other scheduled passenger land transport |
96 |
620000 |
Air transport |
17 |
631130 |
Cargo handling, harbours etc.; travel agencies |
1 |
651000 |
Monetary intermediation |
21 |
652000 |
Financial intermediation n.e.c. |
6 |
670000 |
Activities auxiliary to finan. intermediat. |
4 |
710000 |
Renting of machinery and equipment etc. |
1 |
730002 |
Research and development (other non-market) |
113 |
747000 |
Industrial cleaning |
171 |
748009 |
Other business activities |
75 |
751100 |
General (overall) public service activities |
33 |
751209 |
Public administration for education, health & social care |
116 |
751300 |
Regulation of and contribution to more efficient business operation |
265 |
752002 |
Defence, police and lawcourts |
142 |
801000 |
Primary education |
302 |
802000 |
Secondary education |
164 |
803000 |
Higher education |
103 |
804002 |
Adult and other education (other non-market) |
84 |
851100 |
Hospital activities |
1631 |
851209 |
Medical, dental, veterinary activities etc. |
2 |
853109 |
Social institutions etc. for children |
40 |
853209 |
Social institutions etc. for adults |
458 |
900010 |
Sewage removal and disposal |
20 |
900020 |
Refuse collection and sanitation |
300 |
900030 |
Refuse dumps and refuse disposal plants |
14 |
920001 |
Recreational, cultural, sporting activities (market) |
23 |
920002 |
Recreational, cultural, sporting activities (other non-market) |
293 |
930009 |
Service activities n.e.c |
83 |
4300 |
Maintenance and repair of the dwelling |
165 |
5610 |
Non-durable household goods |
1418 |
7210 |
Maintenance and repairs of motor vehicles |
345 |
9300 |
Other recreational items and equipment |
30 |
9530 |
Stationery and drawing materials etc. |
5 |
9912 |
Appliances, articles and products for personal care |
275 |
Sum: |
|
40119 |
2.5.4 Internal work file from SSF-project (Pedersen 2003)
Courtesy of the project “Miljøvurdering af danskernes forbrugsmønster” financed by Statens Samfundsvidenskabelige Forskningsråd (SSF) we have applied data from their internal work file (Pedersen
2003) providing estimates of the emissions of polyaromatic hydrocarbons and heavy metals to air for each of the 130 industries for the year 1998. We scaled the data to year 1999 by taking into account for
each industry the differences in production volumes between the two years.
2.5.5 Internal work file from lcafood.dk (Dalgaard & Halberg 2004)
Courtesy of the project “Livscyklusvurdering af basislevnedsmidler” we have applied data from their internal work file (Dalgaard & Halberg 2004) providing data on methane and nitrogen emissions and
phosphorous balances for Danish agriculture, split on 29 farm types. The nitrogen emissions and phosphorous balances are calculated on the basis of a detailed farm model described at www.lcafood.dk.
The total phosphorous emission for Denmark is estimated from an average value of 0.5 kg PO43- per hectare, and this total amount is then distributed over the 29 farm types in proportion to their
phosphorous surplus.
2.5.6 Point sources for N and P (Laursen et al. 2000)
Point sources of N and P are provided by Laursen et al. (2000), which has separate chapters on aquaculture and a detailed annex listing names of individual industrial plants, allowing identification and
complete allocation to the 130 industries (incl. waste water treatment facilities). The emissions from households in agricultural areas are allocated to four household activities according to Wrisberg et al.
(2001) (see Table 2.14).
Table 2.14. Sources of N and P in household waste water
(based on Wrisberg et al 2000)
Household activity |
N |
P |
Toilet flush |
86% |
80% |
Dishwashing |
7% |
8% |
Clothes wash |
6% |
10% |
Personal hygiene |
1% |
2% |
|
100% |
100% |
2.5.7 Mass flow analyses
In the late 1990'es, the Danish Environmental Protection Agency commissioned a series of mass-flow analysis of heavy metals in the Danish production and consumption. These publications have been used
to estimate emissions of heavy metals to water.
In general, we have disregarded emissions that are not due to current activities, but are caused by releases from older stock, such as emissions of mercury from thermometers in private use (since mercury
thermometers are no longer sold for private use).
Emissions of cadmium to water come mainly from anodic protection rods. We have distributed this emission on marine activities (oil extraction, fishery, transport by ship and harbours) with 15% to fishery
and ships (0.05 Mg to each) and the rest to oil extraction and harbours (0.25 Mg to each) based on Drivsholm et al. (2000, p. 63). Other sources of emission of cadmium to water are sewage treatment,
primarily from corrosion of galvanised products, such as road signs and roof gutters. Drivsholm et al. (2000) do not specify what products are galvanised. This could in principle be traced via the use of zinc
in the supply-use table, but has not been done in this project.
Emissions of mercury in wastewater are not included under sewage treatment, but allocated to the industries causing the emission, which are medical activities (dentists), extraction of crude petroleum,
manufacture of refined petroleum products and hospital thermometers (Skårup et al. 2003). Emission from thermometers in private use has been excluded as well as emissions from laboratory equipment,
since the emissions reported by Skårup et al. (2003) are mainly caused by old equipment, not current products.
Emission of zinc to water comes from road transport as well as sewage treatment, mainly from corrosion of galvanised products. Galvanised products could in principle be traced via the use of zinc in the
supply-use table, but this has not been done in this project. The total emission to water has been estimated based on Stranddorf et al (2001). The distribution of this onto industries is based on Hansen
(1995b).
Emissions of lead to wastewater come mainly from run-off from lead on roofs (Lassen et al. 2003). Other sources includes paint on historical buildings and from sandblasting on steel bridges, transportation,
extraction of crude oil, paint used in fishery and wear on brakes. Emissions of lead from private and industrial fishing gear is not included, as well as emissions from cables left at the sea floor, since the
availability of metallic lead to biota is very low (Strandorff et al. 2001, p. 176).
Copper emissions to water are mainly due to antifouling paints used on ships (Lassen et al 1996). This emission is distributed based on the maintenance costs reported in the supply-use table (Danmarks
Statistik 2003b) with 80% to shipping, 10% to defence (the navy) and 10% to fishery. Another important source is fishing gear, which is distributed with 40% to fishery and 60% to private use (Recreation),
also based on the supply-use table. Other sources of emission of copper to water are ink, printed circuit boards, copper sulphate from surface treatment of communication equipment, sandblasting of ships,
buildings (run-off from cupper roofs as well as copper pipes), sewage removal and transportation. We have not included emissions from copperware due to the low amount (0.1-1 Mg).
Emissions of tributyltinoxide (TBTO) are reported by Lassen et al. (1997). Danish ships cause a total of 10.2-13.3 Mg TBTO emissions. In the normalisation reference by Stranddorf et al. (2001) only
emissions to Danish waters are included, while we include all emissions caused by Danish ships, in accordance with the principles of national accounting. The emissions have been split 80% on water
transport, 10% on defence (navy) and 10% on fishery, based on maintenance costs reported in the supply-use table (Danmarks Statistik 2003b).
2.5.8 Ozone depleting substances (ODP)
More than 95% of the ozone depletion potential in Denmark in 1999 was caused by HCFC's (Poulsen 2001). HCFC's are applied in insulation foam and as cooling agents in refrigeration systems, mainly for
industrial use. Emissions have not been determined, but emission potentials based on consumption statistics are applied instead. The distribution of industrial refrigeration and freezing systems have been
analysed by using the supply-use table (Danmarks Statistik 2003b). To improve the allocation, a separate “Industrial cooling equipment” industry was separated out from the general industry “Other general
purpose machinery”, and all HCFC use allocated to this new industry. In this way, the use/emission is attributed to the industries buying industrial refrigeration and freezing systems and thus to their products.
2.5.9 Particles (PM10)
The original data from NERI (2003) for year 2000 (year 1999 data not available) are distributed on five groups:
- Road transport
- Other mobile sources and machinery
- Combustion in manufacturing industry
- Non-industrial combustion plants
- Combustion in energy and transformation industry (electricity production)
We have further distributed the emissions data for road transport and other mobile sources based on the 1999 consumption of diesel in all industries and for private cars, and the consumption of fuel-oil in the
shipping industry (industry code 610000).
We further distributed the emissions from combustion in manufacturing industry and in non-industrial combustion plants based on the remaining 1999 energy consumption in GJ (excluding gas, due to the
negligible emission factor for particles from gas combustion) for all industries and for the public sector and private consumption, respectively.
2.5.10 Pesticides
For pesticides it is not possible to find a reliable estimate of the emission to the environment. Instead pesticides are measured as the amount of active ingredient applied. An estimate of this is found in
statistics of the Danish Environmental Protection Agency (DEPA 2000), who monitors the consumption of pesticides in agriculture, horticulture and other uses, and publishes the results on a yearly basis. For
uses outside agriculture, it was possible to distribute the amount of herbicides and insecticides on industries by using information in the supply/use-table (Danmarks Statistik 2003b). For insecticides, we
excluded 2 Mg classified under "Unspecified commodities" in the supply/use-table.
For fungicides, no information was available about the distribution outside agriculture. By default, this amount was therefore distributed between horticulture (industry 11209) and agricultural services
(industry 14000) in same proportions as for herbicides.
2.5.11 Land use (AIS)
Land use is measured as an area occupied in a certain time (m²yr).
For agriculture, the data from lcafood.dk are used (Dalgaard & Halberg 2004).
For areas outside agriculture, we applied data from AIS 100 – Arealanvendelses-kortet, produced at the National Environmental Research Institute (Madsen 2003), derived from satellite photos, the Danish
Address and Road-database and different surveys. One of these is the so-called TOP10-data, which for 1999 only covered 3 regions in Denmark (Ringkøbing Amt, Frederiksborg and Bornholms Amt),
and thus underestimate the land use for activities, which are not common in these regions, such as recreational areas and horticulture. For later years, TOP10-data cover Denmark entirely.
Road area was allocated to the main road transport activities based on their vehicle fuel consumption (76% private cars, 15% freight transport by road, 7% wholesale trade, 2% taxi and coach services).
2.5.12 Missing use stage emissions
Use stage emissions are generally included in the sources mentioned in the previous sub-chapters. However, a few additions or corrections have been made:
The emissions from combustion of vehicle fuels for final use are generally allocated to private car driving. However, a part of the emissions are due to combustion in lawn-mowers and other motor-tools for
gardening. The main emissions for these tools were estimated from Bak et al. (2003), while the minor emissions were estimated from the vehicle emissions using the assumption that gardening machines have
the same percentage of other emissions as they do of CO2-emissions (1%). The resulting emissions were then subtracted from the vehicle fuel emissions before these were distributed over the different
purposes of private car driving (see Chapter 2.7) and instead allocated to "Tools and equipment for house and garden." Land use is not attributed to gardening machines, nor has the fuel input (i.e.
precombustion emissions) been allocated to the final use group "Tools and equipment for house and garden."
We have added emissions from fuel use to "Car driving for holiday abroad," based on the emissions from Danish private car driving (same emissions per DKK spent on petroleum products, using buyer's
prices, i.e. incl. product taxes and VAT). This estimate is reasonable, since the buyer's price of petrol varies only slightly between the countries most obvious for the Danes' car-holidays (Vejdirektoratet
2004).
We investigated the data from the Dutch prioritisation project (Goedkoop et al. 2003) for sources of emissions that were not covered by the data from Statistics Denmark and NERI. The following four
items were added to the Danish NAMEA:
- Wear on vehicle tyres and belts in private cars
- Fireworks
- Combustion of tobacco
- Combustion of candles
using the emission factors given in table 2.15. The data for private cars were extrapolated to other road traffic using the same data as for land use (see Chapter 2.5.11).
Data on the number of km driven in Denmark was found in Danmarks Statistik (2002), whereas data on amount of fireworks, tobacco and candles used in Denmark was found in the supply-use tables.
The contributions are small compared to the overall emissions, except for Cu to water, where fireworks contribute a total of 72 Mg (compared to a Danish total emission of 170 t). This value is not
confirmed from other sources and is highly uncertain. In proportion to the total Danish emissions, wear on vehicle tyres and belts contributes mostly to Zn emissions to water (20 t out of a Danish total
emission of 123 t), tobacco combustion contributes mostly to particulate emissions (0.7% of the Danish total), and candle combustion contributes most to CO emissions (1.8% of the Danish total).
Table 2.15. Emission factors for minor sources (based on Goedkoop et al. 2003)
|
Wear on
vehicle tyres
and belts |
Fireworks |
Tobacco
combustion |
Candle
combustion |
kg/vehicle-
km |
kg/DKK
(buyers
price) |
kg/kg
tobacco |
kg/kg
candle |
CO2 |
air |
|
3.10E-03 |
1.33E-01 |
3.1E+00 |
Methane |
air |
|
1.40E-04 |
|
|
CO |
air |
|
2.17E-03 |
5.18E-02 |
4.0E-01 |
SO2 |
air |
|
1.43E-04 |
|
5.0E-05 |
NOx |
air |
|
3.15E-05 |
2.30E-04 |
|
Ammonia |
air |
|
|
3.05E-05 |
|
Ni |
air |
|
|
4.75E-06 |
|
N2O |
air |
|
1.41E-04 |
|
|
NMVOC |
air |
|
6.56E-03 |
1.50E-03 |
1.0E-02 |
As |
air |
8.1E-11 |
|
|
|
Cd |
air |
2.4E-11 |
|
2.90E-09 |
|
Cd |
water |
1.22E-10 |
|
|
|
Cu |
air |
5.08E-09 |
4.21E-05 |
1.00E-07 |
|
Cu |
water |
1.74E-08 |
1.84E-04 |
|
|
Zn |
air |
5.52E-08 |
|
1.43E-06 |
8.2E-06 |
Zn |
water |
4.12E-07 |
|
|
|
Pb |
air |
1.19E-09 |
|
2.20E-07 |
3.1E-06 |
Pb |
water |
6.28E-09 |
|
|
|
Fluoranthene |
air |
|
|
2.01E-07 |
|
PAH (as benzo(a)pyrene) |
air |
|
|
4.05E-08 |
|
Particulates (PM10) |
air |
1.9E-05 |
8.00E-04 |
5.00E-02 |
5.0E-07 |
2.6 Adjusting the level of aggregation of industries
The classification of the Danish production is described in Plovsing and Dalgaard (1997). This follows the EU statistical classification of activities and products (NACE, CPA and PRODCOM), which is fully
harmonised with the similar international ISIC and CPC classifications (i.e. an aggregation of categories in one system matches a category in the other system).
We have made the following modifications in order to target regulatory meaningful product groups:
- Industries 403000 (Steam and hot water) and 401000 (Electricity) were aggregated, since the original allocation by Statistics Denmark was not based on causal mechanisms.
- Agriculture and horticulture were disaggregated into 28 farm types based on the supply-use table (Danmarks Statistik 2003b) and economic statistics for the 28 farm types from Dalgaard & Halberg
(2004).
- The meat industry was disaggregated into three industries for the three main outputs (Pork, Beef and Chicken meat), based on data from the supply-use table (Danmarks Statistik 2003b) on input of
live pigs, cattle and chicken, respectively. Internal turnover of meat-products (semifabricata), i.e. from "Production etc. of meat and meat products, DK" to the same industry are distributed under the
assumption that only pork-products are used as input in the pork-production and so on. The main purpose of this disaggregation is to provide a direct link between the demand for a specific type of
meat and the supply of the matching animals from agriculture.
- Manufacture of starch, chocolate and sugar products (industry code 156009) was disaggregated into nine industries (Dog and cat food, Other animal feeds, Chocolate and cocoa products, Candy
and other sugar products, Flavoring extracts and flavoring syrups, Roasted coffee, Other food preparations, Flour, and Oatflakes), based on the supply-use table (Danmarks Statistik 2003b). By
comparing the output of flour with the necessary grain input to produce this flour, it was established that all grain input to the original aggregated industry would be required for the new “Flour” industry
(but no other edible inputs). Similar considerations were made with regard to the inputs of all other commodities and when a clear relationship between an input and one or more specific industries
could be established, the input was allocated to this or these industries (e.g. all fish to Other animal feeds, no edible inputs to oatflakes but oats, no edible inputs to roasted coffee but coffee). When
more than one industry could be identified (e.g. all meat to Dog and cat food, Other animal feeds, and other food preparations), the allocation was made on basis of their relative production value. All
non-edible inputs, except toys, were allocated evenly according to production value. Toys were allocated to “Chocolate and cocoa products” and “Candy and other sugar products”. The output from
the original aggregated industry was disaggregated to the new industries according to commodity data from the supply-use table (Danmarks Statistik 2003b). Emissions were disaggregated according
to production value except for N-tot and NMVOC where the original data source allowed a better specification.
- To isolate the commodity “Køle- og fryseudstyr ian.", which was identified as solely responsible for the emissions of ozone-depleting substances, this commodity was split out from “Manufacture of
other general purpose machinery” (industry code 292000), both for domestic and imported products, resulting in the new industry: “Manufacture of industrial cooling equipment”. Inputs to the new
industry are the same as for the original industry (relative to production value). The same applies to emissions, except for ODP, where all emissions are allocated to the new industry. For imported
industrial cooling equipment, the same emission factor per DKK is applied for ODP as for domestic produce.
2.7 Adjusting the level of aggregation of consumption
The classification of the Danish consumption is described in Plovsing & Dalgaard (1997).
We aggregated some final uses for which the distinction does not have any environmental relevance:
- Two types of out-patient services by medical doctors and dentists (market and non-market government consumption)
- “Recreational and cultural services” and “Recreational, Cultural and religious affairs and services”
- “Education” and “Education and Research Affairs and services”
2.7.1 Accounting for investments and FISIM
The Danish input-output tables (Plovsing & Dalgaard 1997) follow the traditional convention of including investments in capital goods as a final use, rather than as an intermediate consumption. For
input-output analysis, this implies that the investments are not linked as an input to the other items of final use, as is typical in the practice of life cycle assessment. To include the investments as intermediate
consumption, the investment of each industry (recorded under final use) must be redistributed to the industries supplying the investment goods. We have performed this correction, based on the investment
matrices of Statistics Denmark (Danmarks Statistik 2003c), which provide investment data aggregated on 57 supplying industries. We further disaggregated this to the level of the 130 industries of the IO
table, based on an internal work file from statistics Denmark. In this process, negative investments were regarded as changes in stock and thus eliminated. An exception to the described procedure is
“agricultural breeding stock” and “net acquisition of valuables”, which are not capital goods in the traditional sense, but should rather be seen as items of changes in stock, i.e. as a net storage of value in the
same industry that supplies the investment good.
The Danish input-output tables (Plovsing & Dalgaard 1997) also follow the traditional convention of including financial intermediation services indirectly measured (FISIM), i.e. bankers' net interest, as a final
use rather than as an intermediate product of the financial industry. For input-output analysis, this implies that this income of the financial industry is not linked to the other items of final use, as is typical in the
practice of life cycle assessment. To include financial intermediation services as an intermediate consumption, we have redistributed the FISIM expenditure (recorded under final use) to the financial industries
supplying the loans. This redistribution is done in proportion to the non-FISIM spending of each industry and private consumers (group 9950 “Financial services n.e.c.”) on each of the financial industries
651000 and 652000.
2.7.2 Combining products in the use stage
We rearranged some of the groups of final consumption in order to obtain results that resemble LCA results, i.e. groups that better reflect the functions of the different products in their combined use in the
households:
- We disaggregated major household appliances and repair of major household appliances into new groups of household activities (Storage of food, Cooking, Dishwashing, Clothes washing, Personal
hygiene, Toilet flush, Cleaning, TV computer etc., Lighting, Heating, and Other household activities with energy-use), based on data from the supply-use tables (Danmarks Statistik 2003b). Private
consumption of water, electricity, gas, liquid fuel, hot water, as well as the associated emissions, was distributed over the new household activities, based on Dall et al. (2002). The items of
"Appliances, articles and products for personal care” were allocated to the new activity Personal hygiene, and the commodity “Toilet paper” to the new activity Toilet flush.
- We have regrouped “Purchase of vehicles”, “Repair and maintenance of motorvehicles”, and “Fuels and lubricants” (with the use stage emissions), according to purpose of trip (work, shopping, leisure
activities), based on data from the Danish investigation on transport habits (“Transportvane-undersøgelsen”) as reported in Bach et al. (2001, Figure 1.4.6). A further "Car driving for holiday abroad"
was disaggregated from "Consumption of residents in the ROW" (tourist expenditures abroad) covering the items Refined petroleum products etc. (gasoline/diesel), Repair and maintenance of motor
vehicles, and Renting of vehicles etc. (see Chapter 2.4.1).
- We have split out "Detergents, prepared for use" and "Candles" from the original "Non-durable household goods," based on data from the supply-use tables (Danmarks Statistik 2003b).
- We have split out toys (still including minor amounts of jewellery), pet food and veterinarian services, Christmas trees, tools and equipment for recreation, household textiles, and fireworks from the
original "Other recreational items and equipment," based on data from the supply-use tables (Danmarks Statistik 2003b).
2.8 Improving the modelling for imported products
In traditional Input-Output Analysis, the standard procedure is to apply to the foreign industries the same emission factors (environmental exchange per DKK) as for the corresponding Danish industry.
This assumption was applied in an initial analysis, and showed that the imports to Denmark resulted in an average environmental impact of a size approximately 1/3 of the environmental impact from the
Danish production and use stages. As Denmark has very little raw material extraction and primary processing, it is to be expected that applying Danish emission factors to foreign production will result in an
underestimation of the actual environmental impact. This expectation was confirmed in a later analysis, where emission factors from USA was used for the foreign industries. This resulted in an average
environmental impact of a similar size as the environmental impact from the Danish production and use stages, i.e. three times the original result. Obviously, this value varies from impact category to impact
category, and the above values should be seen only as rough average indications.
The simplest improvement that can be made is to apply emission factors that are more representative for the foreign industries. Such emission factors can be obtained from foreign NAMEAs or other
sources. For this purpose, we obtained NAMEAs for the following countries and country groups:
- Six European countries (Germany, Sweden, the Netherlands, United Kingdom, France and Norway)
- USA (Suh 2003)
- Europe, Non-Europe OECD, and Non-OECD, in the form of aggregated GTAP-based 30-industry NAMEAs (Goedkoop et al. 2003, Nijdam & Wilting 2003).
Due to the limited time available to us, we first analysed the NAMEAs to see which set of emission factors would be most appropriate to apply as a default for all foreign industries.
In the process of comparing emission factors between the different NAMEAs, we discovered some very large emission factors in some of the GTAP-based NAMEAs, which were found to originate in a
mis-allocation of the original emissions data from the EDGAR emissions database. For this reason, we decided to refrain from using the GTAP-based NAMEAs. Another reason for not applying the
GTAP-based data was our investigation into the causes of uncertainty (see also Chapter 2.11), which show that a high level of industry-aggregation, as in the GTAP-based NAMEAs, implies a larger
uncertainty than transferring less aggregated data between countries, i.e. using data from a “wrong” country.
The NAMEAs from the six European countries are at very different level of aggregation (between 50 and 200 industries) and including very different numbers of emissions (generally less than in the Danish
NAMEA, and restricted to air emissions). For this reason, it was decided to use the US-American table (Suh 2003) as a starting point for developing a default for all foreign industries. Contributing to this
decision was the relatively low level of aggregation of the US table (493 industries), the high number of emissions available (more than in the Danish NAMEA) and the relatively high completeness of the
US-American economy in terms of industries covered (due to the size of the country, practically all kind of industries are found within the country).
Since 70% of the products imported to Denmark come from other European countries, we proceeded to analyse the US NAMEA with the aim of identifying the necessary adjustments to make the data
suitable as a proxy also for European industries exporting to Denmark. As a starting point, we compared the emission factors from the US NAMEA (as provided by Suh 2003) to the emission factors from
the closest corresponding Danish industries, using the conversion factor 7.25 between DKK99 and USD98, assuming a default 4% annual reduction in emission factors.
In general, we found the original US data to provide a reasonable proxy for imports to Denmark, while in some instances we found it necessary to make adjustments to the US data. The adjustments made
were:
- For CO2, the emission factor was 0 for the US 820000 general government industry. We applied here the Danish emission factor for DK 752000 Provision of services to the community.
- For CH4, the emission factor for dairy farms was found unrealistically low and the Danish emission factor applied instead.
- For SO2, low sulphur content of US fuels result in lower emission factors than what is appropriate for European conditions. We therefore calculated the SO2 emission factor from the US CO2 data
and the SO2/CO2 relationship of the closest corresponding Danish industry (with the exception of the US data for paper mills, petroleum refining, cement manufacture, primary metals and some
chemicals industries where Denmark do not have much corresponding industry, and where US SO2 data therefore appear more appropriate).
- For NOx and N2O for agriculture and transport industries we similarly applied the Danish NOx/CO2 and N2O/CO2 relationships, since the US data were found lacking for these specific industries.
- For NH4, US emission factors for agriculture are not differentiated among livestock and crop types, so here we applied the Danish emission factors as the more relevant.
- For N-tot, we used US data for Nitrate compounds, except for agriculture, fishery, fish processing, air transport and sanitary services (refuse dumps), where we found the US data lacking.
- For most heavy metals to air (Ni, Hg, Cd, Pb), the Danish emission factors are mainly fuel related, while the US emission factors appear to be more process-specific. We have therefore used the US
Ni factors for coal and the steel and machine industries (US codes 70000, 260806 and 370101 to 641200), US Hg factors for non-ferrous metal ores, coal, refuse dumps and inorganic and
agricultural chemicals, US Cd factors for scrap, refuse treatment and aluminium castings and similarly US Pb factors for a number of industries. The remaining data has been extrapolated from CO2
use, using heavy-metal/CO2-ratios from the closest corresponding Danish industry. Cu and Zn emissions have not been included for foreign products.
- For PM10, we have used Danish coefficients when US coefficients were 0 or close to 0, as we have assumed that such values are the result of differences in US reporting requirements.
- For heavy metals to water and soil, we have used the US data unedited, except for Hg from dentists, hospitals, and oil extraction and refining, Cu from printing, Cd and Cu from fishing, transport and
wholesale trade, Pb from fishing, and TBTO from ship and boat building, where we found the US values unreasonably low.
- For pesticides, we have used the overall pesticide emission factor for Europe from Goedkoop et al. (2003), the Danish proportions for distribution over industries and farm types, and a percentage
split among herbicides, fungicides and insecticides of 39/58/3, based on a rough estimate from the FAO pesticide statistics.
- For land use and phosphate emissions, Danish coefficients have been applied.
Ideally, our corrections should be verified by national emission experts, but failing this, we believe the above procedure is better than no correction. Ideally, we should have used emission factors from as
many countries as possible, to make the comparisons (and corrections) as valid as possible. Clearly, the use of the Danish emission factors alone is clearly a minimum procedure due to the lack of time for a
more elaborate procedure.
The resulting “US NAMEA with Europe-adjusted emission factors” was then used to calculate the “cradle-to-wholesale” emissions per product from each industry (see Chapter 2.13 for a description of the
standard LCA calculation routines). The resulting 493 products were then aggregated to the industry levels of the Danish NAMEA. These aggregated default “cradle-to-wholesale” emissions for products
imported to Denmark are included in the database from the project (see chapter 7).
When linking Danish imports to the US data, the purchase values less import tax were used.
Although country-specific emission data were not found to be available in sufficient detail, it would have been preferable to further detail the treatment of imported products by linking the most important
foreign IO-tables directly to the Danish, and adjust the default emission factors with country-specific emission factors when available. This would have allowed more detailed modelling, e.g. of improvement
options, market sensitivities etc.
However, the linking of IO-tables required more resources than available to this project, notably because of the different levels of aggregation between countries and different dividing lines between
industries.
2.9 Adjusting for market constraints
In standard IO-analysis, all the links between industries are assumed to contribute proportionally to the result, for example the environmental impact of milk will appear as a combination of the impacts from
the chain from dairy back to agriculture, fertiliser industry etc. However, such a calculation does not take into account that it is not all industries that can influence their entire supply chain in this way. For
example, because of the quotas on milk production, a change in the output of milk from the dairies will not be able to influence the amount of milk produced in agriculture, and therefore not the environmental
impacts from agriculture either. An increased output of milk will instead be met by decreasing the output of milk powder and butter. The only way the environmental impact of dairy farms can be influenced is
by placing explicit demands on the technology applied on the farms. This can be a direct regulation or it can be a consumer demand for BAT milk (e.g. milk from ecological farming).
To reflect this in the expanded NAMEA, i.e. to reflect the way each industry reacts on changes in supply and demand, we analysed all industries systematically for long-term production constraints, i.e.
constraints that influence investment decisions, like the one mentioned for dairy farms. This means that for each industry, we have investigated:
- Are there any regulatory or political constraints that determine the production output, so that this output cannot change in response to a change in demand?
- Does the industry have any co-products, the output of which cannot change in response to a change in demand, since it is determined by the demand for a determining product?
- Are there any long-term constraints in availability of raw materials, waste treatment capacity, or other necessary production factors?
As a result of our analysis, we identified the following main areas where constraints play a significant role:
- Agriculture, fishery, and the food industry, where some products are limited by quotas or similar regulatory arrangements and where there are a number of dependent by-products, for which the output
cannot change in response to a change in demand, notably animal hides, meat from milking cows, and fodder by-products of the food industry, where a change in demand in practice will lead to a
change in output of the least-cost unconstrained fodders, typically soy for protein (with oil as a by-product) and grain for carbohydrates.
- The vegetable oil industry, where soy oil is a dependent by-product, for which the output cannot change in response to a change in demand.
- Electricity generation, where some sources of power (wind power, hydropower, nuclear power) are constrained in some regions and where a change in demand for the by-product heat in most
situations does not lead to a change in production volume.
- The recycling industry, which is ultimately constrained by the supply of scrap materials.
- Industries in decline, such as the European ammonia and chlorine industry, where there is a constraint on building of new production plants, so that a change in demand will affect the least-profitable
production units, typically with the highest emission factors.
In most other industries, changes in demand will affect the modern plants, typically with low emission factors (Weidema 2003). This implies that using average emission factors will lead to a systematic
overestimation of the impact of a change in demand. However, since this overestimation will affect all industries, it should not affect the overall ranking of product groups, and we have therefore not adjusted
the data for this general overestimation. However, for a truly market-based database, we recommended to supplement the database with specific modern processes in the cases where there is a significant
difference in technology and emissions between the average and the modern plants. Since we have not implemented this recommendation in the expanded NAMEA, we call our version market-adjusted
rather than market-based.
The market adjustments that we have implemented for the ranking take into account the most important of the production constraints in the above bullet-points; see details below.
For each constrained supplying industry, we first identified the alternative most sensitive supply route (or in the case of input constraints, the most sensitive alternative consumption or treatment route)
according to the procedure of Weidema (2003).
We then created a separate copy of the expanded NAMEA, named “market-adjusted model.” In this version of the NAMEA, the following adjustments were made, for the most important constrained
industries:
- The industry is divided in a constrained and a non-constrained part.
- The constrained supplies are transferred to the alternative non-constrained industry.
- The constrained outputs are added as separate products in new final consumption group, typically named “industry name (constrained supplies)”. Since a constrained production is still relevant for
non-market-based environmental measures, this new product takes part in the prioritisation in the same way as any other product.
- The additional supply from the non-constrained supplying industry is matched by an identical reduction in the entry for that industry in a new column “Constraints adjustments”. In this way, the total
production volume and thus the total emissions of all industries are kept constant, while making the model sensitive to life cycle simulations.
More specifically, the following describes in detail the market adjustments made. The documentation for the described market conditions is provided in Weidema (2003) unless otherwise stated:
- Since milk from Danish agriculture is constrained by quotas, the input from Danish agriculture to dairy industry as well as the resulting “surplus” output of dried milk and butter is moved to a new group
of final consumption: "Dairy products (constrained), DK". Instead, input of milk to the dairy industry comes from a new industry: "Milk rerouted from dried milk and butter production, DK"
- Since Danish cattle production is constrained (Nielsen et al. 2003b, page “Living cattle ex farm”), the input of live cattle from agriculture to the meat industry is moved to a new group of final
consumption “Live cattle (constrained), DK”. Instead, input to beef production comes 50% in the form of live pigs and 50% in the form of “Meat animals, ROW” (Nielsen et al. 2003b, page “Living
cattle ex farm”).
- Since Danish sugar production is constrained (Walter-Jørgensen et al. 2001), the entire Danish sugar industry is renamed into a final consumption group “Sugar (constrained), DK”. Instead, the input
of sugar to other industries and to final consumption comes in the form of “Sugar, ROW”.
- Since only specific farm types deliver the live pigs and chicken that result from marginal demand changes (Jensen & Andersen 2003), the output from other farm types is moved to new groups of final
consumption “Live pigs (constrained), DK” and “Chicken (constrained), DK”. Instead, input to meat industry and final consumption (incl. export) come from the farm types that change output in
response to marginal demand changes (Jensen & Andersen 2003).
- Similar adjustments are made for internal turnover of seeds and grains within agriculture, input of barley to the beverage industry, input of wheat to fish processing industry, input of rape seed to oil
industry and paint manufacture, input of grain to flour industry, input of oat to oatflakes industry, input of straw to electricity and construction materials, as well as export of grain, resulting in a new
group of final consumption “Seeds and grains (constrained), DK”. For each of these products, the farm type with the largest production is assumed also to be the one that change output in response to
marginal demand changes. The same adjustment is also made for input of fur to dressing.
- The supply of constrained co-products from the farm types that change specific outputs in response to marginal changes in demand, are moved from these farm types to the farm types that have these
co-products as unconstrained output. This procedure, also known as “system expansion” (see Weidema 2003), results in a number of farm types which each have only one product as output.
- Since the supply of fodder by-products from the food industry (animal fat, meat meal etc., beet tops and molasses, bran and other grain milling by-products, and residues from starch production) and
the supply of industrial fish for fodder are constrained, the input of these products to agriculture and aquaculture is moved to a new group of final consumption “Feed products (constrained), DK”.
Instead, this input of fodder comes in the form of soy protein (for the protein-rich by-products) or feed grains (for the carbohydrate-rich by-products), both imported.
- Since the supply of animal hides is determined by the production of animals for milk or meat, the production of hides is split out from the meat industries, and assigned no emissions. The inputs and
emissions to the meat industry are thereby distributed solely on the meat products.
- Since most sources of electricity and heat are constrained by political decisions and emission restrictions, the input is moved to a new group of final consumption “Electricity and district heat
(constrained)”. Instead, electricity comes from the unconstrained supply based on natural gas (applying a fuel efficiency of 45% and the 1999 emission factors for Danish large natural gas fired power
plants – SNAP code 010102 - from NERI 2000) and heat is calculated as coming from 75% emission-free co-product from electricity production and 25% biomass-based dedicated district heat
plants, using a fuel efficiency of 65% and the 1999 emission factors for Danish district heat plants – SNAP code 010202 - from NERI (2000) for straw, except for SO2 and NOx where the lower
emission factor for wood was used, since the off-gasses are assumed to be cleaned to this level. The electricity consumption associated with heat distribution is calculated as 2.1 Wh/MJ (VK 2001).
All of the above adjustments have been made under the simplified assumption of equal monetary value of the constrained and the unconstrained products, i.e. the total production value of each industry is
kept constant. A more precise calculation could be made by using data on the physical amounts of unconstrained products that replace the constrained products, but comparing the effort required by this
approach as well as the additional assumptions that would thereby be introduced, we have judged our simplified approach to be preferable for the purposes of this project.
Furthermore, the following adjustment was introduced into the database from the project, see Chapter 7, but was not included in the version applied for the prioritisation, since it was estimated that this
would not influence the prioritisation:
- Since the recycling industry is ultimately constrained by the supply of scrap materials, the scrap output from the recycling industry is moved to the industry supplying the equivalent virgin material, while
the recycling industry is maintained as a service supplying industry to the scrap supplying industries. In this way, emissions of the supplying industries are no longer assigned to scrap as a commodity,
but rather the opposite: the emissions of the recycling industries are assigned to the scrap supplying industries. In return, the new recycling processes provide emission credits to the supplying industries
equal to the value of the supplied scrap, which is assumed to reflect the amount of primary material that is replaced by the supplied scrap. The adjustment reduces the turnover of the supplying
industries by the original value of the traded scrap, which implies that their emission intensities increase. This is a reflection of the adjusted situation where emissions are no longer assigned to scrap as a
commodity.
For some of the identified constraints, no adjustments were made, since it was estimated that this would not influence the prioritisation. This applies to:
- Fish and fish products: Although fish supplies are constrained by quotas, fish do not contribute significantly as an input to other products (with the exception of fish for fodder, which was treated
above) and therefore the entire fish and fish product industry, as already shown in the prioritisation, can therefore simply be regarded as constrained (Nielsen et al. 2003b, page “Wild fish”).
- Vegetable oils: Although soy oil is constrained by its determining co-product (soy protein), forming an integrated relationship with rape as the marginal source of edible oil (Weidema 1999), we have
not separated out soy and rape from the vegetable oil industry. A comparison to the database of Nielsen et al. (2003b) shows that the soy-rape cycle may indeed be of importance, but also very
dependent on the assumptions made regarding the specific emissions. In the database from the project (see Chapter 7), the soy-rape data from Nielsen et al. (2003b) are provided for the purposes of
sensitivity analyses.
- Ammonia: Although the ammonia production in Europe is generally constrained by a declining demand, we have not found the available process data (e.g. the ones used by Nielsen et al. 2003b) to
have an adequate completeness to make them preferable to the average NAMEA data. In the database from the project (see Chapter 7), the fertiliser data from Nielsen et al. (2003b) are provided
for the purposes of sensitivity analyses.
- Chlorine: Although the chlorine production in Europe is generally constrained by a declining demand, we have not found the foreign NAMEAs adequately detailed to allow an identification of what
industries use chlorine as input. In Denmark, chlorine is nearly exclusively used in the pharmaceutical and detergent industries, so to apply the Danish NAMEA for this purpose would introduce an
unwanted bias in the analysis.
2.10 Impact assessment
Different possible approaches to impact assessment was discussed with the project reference group. Based on these discussions, the group chose to apply the Danish EDIP-method for the external
environment (Wenzel et al. 1997), supplemented with an impact category for nature occupation (see Chapter 2.10.2) and an equal weighting (see section 2.11.4) of the resulting 8 impact categories:
- Global warming
- Ozone depletion
- Acidification
- Nutrient enrichment
- Photochemical ozone formation
- Ecotoxicity
- Human toxicity
- Nature occupation
In the course of the project, some EDIP characterisation and normalisation factors were updated, see sub-chapters 2.10.1, 2.10.3 and Chapter 3.
2.10.1 Characterisation factors
In general, the EDIP characterisation factors from Wenzel et al. (1997) have been applied. For photochemical ozone formation, the values for high background concentration of NOx have been applied to
ensure consistency with the SimaPro version of the EDIP method.
We have added characterisation factors for particulates, tributyltinoxide and unspecified herbicides, fungicides and insecticides, based on Stranddorf et al. (2001). To provide a consistent treatment of PAHs
from domestic and foreign industries, they have all been aggregated to benzo(a)pyrene-equivalents, based on MOE (1997) - and CEPA (1993) for fluoranthene. In general, all toxicity factors have been
updated with the most recent information from Olsen (2003).
The applied characterisation factors are site-generic, i.e. they do not take into account that the same amount of emission may have different impacts depending on the location of the emission. Particularly for
transport by ship this may lead to an unreasonably high impact for acidification, nutrient enrichment and human toxicity, since a large share of the emissions take place at high sea and therefore never reach
neither the sensitive ecosystems nor humans. Rather than applying specific characterisation factors for emissions from shipping, we have simply roughly simulated a site-dependent impact assessment by
reducing the emissions of SO2, NOx, ammonia, VOC, PAH and particulates from “Transport by ship” to 25% of those originally reported in the extended NAMEA. In this context, it is also worth noting
that due to the specific atmospheric conditions in marine areas, the marine NOx emissions are highly likely to have a larger effect on global warming than NOx emitted over land, but more research is needed
to quantify these influences (Skjølsvik et al. 2000), and we have therefore not made any corrections for this.
2.10.2 New impact category: nature occupation
Land use is specified in two categories:
- Land use (100% occupied), covering crop production, housing and infrastructure, where the natural vegetation is generally assumed to have been suppressed completely,
- Land use (33% occupied), covering cultivated forests and permanent grassland,
with the unit m²yr.
The characterisation factors applied are:
- Land use (100% occupied): 3.4 PAFm²yr/m²yr.
- Cultivated forest and permanent grassland: 0.33 PAFm²yr/m²yr.
PAF is an abbreviation of Potentially Affected Fraction, i.e. the number of native species that are assumed to be negatively affected by the occupation.
The maintenance of housing, infrastructure and crop production during one year affects 100% of the species during this year. In addition, these types of land use typically occupy arable land, and thereby
contribute to the general expansion of land under human use and thus to the annual global deforestation. The gross deforested area of natural forests is 0.15 E12 m²/year (FAO 2001). Assuming an average
relaxation time of 540 years (Weidema & Lindeijer 2001) and 50% average depression of the natural ecosystem during this time, the resulting nature occupation is 40.5 E12 m²/year. This is 2.4 times the 17
E12 m² currently used globally for human settlements and arable and permanent crops. Thus, to take deforestation into account, every area of arable land currently used is calculated as resulting in an
occupation of 3.4 times this area, i.e. 3.4 PAFm²yr/m²yr.
For cultivated forest and permanent grassland, it is estimated that 1/3 of the native species are sensitive to the activities (Weidema & Lindeijer 2001), resulting in the characterisation factor 0.33
PAFm²yr/m²yr.
The above is a simplification of the impact assessment for physical impacts of land use developed for the EDIP method by Weidema & Lindeijer (2001). In this method, detailed calculations were made to
take into account that ecosystems differ in species richness, vulnerability and inherent scarcity. Since these factors of ecosystem quality can only differ between 0 and 1, it turned out that in practice the impact
assessment is dominated by the occupied area of ecosystem, and only to a lesser extent influenced by the specific qualities of the ecosystems occupied. This is reinforced by the fact that most human activities
take place in areas that have a high ecosystem quality, and therefore do not differ so much in this respect. Together, these arguments lead us to apply the above simplification, which focus mainly on the area
occupied, and does not distinguish between the ecosystem qualities of the occupied areas.
Also, the above simplification focuses on biodiversity impacts, since this is regarded as the most important physical impact of land use, compared to the impacts on substance and energy cycles and natural
productivity, which was also covered by the method developed by Weidema & Lindeijer (2001).
2.10.3 Normalisation
The EDIP normalisation references of Wenzel et al. (1997) relate to the environmental impacts from Danish production and final use in 1990 (Global production and final use for the impact categories global
warming and ozone depletion). These references were updated to 1994 by Stranddorf et al. (2001). However, since our project applies emission data from 1999, Danish normalisation references for 1999
is an incidental by-product of our work (see Chapter 3).
However, since our assessment covers also emissions abroad caused by Danish production and consumption, it would give our assessment an unintended bias if we applied the Danish normalisation
reference. This would be particularly noticeable for impact categories where Danish impacts are relatively low (such as ozone depletion and toxicity), since the foreign emissions would make these impact
categories appear much more important than the other impact categories when measured in units of Denmark-equivalents or person-equivalents. This bias could be corrected for in the weighting, but we
found it more satisfactory to apply a normalisation reference that reflects the object of our study, i.e. the total environmental impact caused by Danish production and consumption. Thus, the normalisation
reference applied in this study is: The total environmental impact caused by Danish production and consumption in year 1999, see Table 2.16. Additional to the impacts from Danish production and
final use, this includes impacts caused abroad by production of products imported to Danish industries and final use, but excludes impacts caused by re-exported products, as well as impacts caused abroad
by consumption of products produced in Denmark.
To make the normalisation value more meaningful, it has been divided by the Danish population in 1999 (5313577 inhabitants), resulting in a value expressed per person. Thus, this value - in
person-equivalents - express the total environmental impact caused by the production and consumption of an average Dane in 1999.
The normalisation reference in Table 2.16 is affected by the reduction of the emissions of SO2, NOx, ammonia, VOC, PAH and particulates to take into account site-specific aspects of “Transport by ship”
as described in Chapter 2.10.1. This affects the values for acidification, nutrient enrichment, photochemical ozone and human toxicity air. Without this reduction, the values for these impact categories would
have been 1.64E+06 Mg SO2-eqv. (36% larger), 3.49E+06 Mg NO3--eqv. (14% larger), 2.46E+05 Mg C2H4-eqv. (2% larger) and 2.71E+16 m³ air (14% larger), respectively.
Table 2.16. Total environmental impact caused by Danish production and consumption in year 1999
Impact category |
Abbre-
viation |
Unit |
Normalisation
reference |
One person-
equivalent |
Global warming |
gw |
Mg CO2-eqv. |
1.83E+08 |
3.44E+01 |
Ozone depletion |
od |
Mg CFC-11-eqv. |
2.04E+02 |
3.84E-05 |
Acidification |
ac |
Mg SO2-eqv. |
1.05E+06 |
1.98E-01 |
Nutrient enrichment |
ne |
Mg NO3--eqv. |
2.99E+06 |
5.63E-01 |
Phtotchemical ozone formation (high NOx) |
po |
Mg C2H4-eqv. |
2.41E+05 |
4.64E-02 |
Ecotoxicity water chronic |
etwc |
m³ water |
1.93E+13 |
3.63E+06 |
Ecotoxicity water acute |
etwa |
m³ water |
1.90E+12 |
3.57E+05 |
Ecotoxicity soil chronic |
etsc |
m³ soil |
4.84E+12 |
9.11E+05 |
Human toxicity air |
hta |
m³ air |
2.34E+16 |
4.40E+09 |
Human toxicity water |
htw |
m³ water |
3.47E+12 |
6.54E+05 |
Human toxicity soil |
hts |
m³ soil |
2.72E+09 |
5.13E+02 |
Nature occupation |
biodiv |
PAFm²yr |
1.87E+11 |
3.52E+04 |
2.10.4 Weighting
The three categories of ecotoxicity (water chronic, water acute, soil chronic) are aggregated into one, based on their normalised values. The same is done for the three categories of human toxicity (air, water,
soil). This implies that each of the three sub-categories receives equal weight within the overall categories of ecotoxicity and human toxicity.
In most cases, results are presented per impact category, but for some purposes, e.g. the general presentation in Chapter 1.1, and for selecting the top 10 most important product groups in Chapters 1.2 to
1.4, the eight impact categories have been weighted equally based on their normalised totals. This implies that each impact category contributes with 1/8 to the total environmental impact of Danish
production and consumption as reported in these chapters.
2.10.5 Limitations of the impact assessment
The eight impact categories do not cover all environmental problems. Notably, the following issues are not covered:
- Species dispersal
- Poverty-related health issues
- Accidents
- Occupational health
- Noise
Species dispersal is mainly related to transport vectors and imports of biological materials. This implies that the exclusion of this impact category mainly leads to an underestimation of the importance of
transport products and products that involve much transport, and especially imported biological products. However, even with the current impact assessment method, transport and biological products
receive much attention. Thus, the exclusion is not likely to affect the prioritisation significantly.
Poverty-related health issues are mainly affected by trade and investment in countries with a low average income. Thus, the exclusion of this impact category mainly leads to an underestimation of the
positive effects of importing products from such countries.
Accidents (besides accidents at the workplace) are mainly related to traffic and to a lesser extent to specific household activities. As mentioned above, transport already receives much attention in the
prioritisation, even without the inclusion of this impact category.
Occupational health (including accidents at the workplace) could be included in the assessment by applying the method developed by Schmidt et al. (2004). The possible result of this has not been
estimated.
Also noise is mainly related to road traffic, and thus reinforces the focus on transport activities.
Besides the exclusions, the main limitation of the impact assessment method lies in the equal weighting of the normalised impact categories, which does not reflect their true importance, e.g. in terms of number
of affected individuals or ecosystems.
2.11 Uncertainty analysis
Uncertainty in using IO-tables for environmental analysis can be broadly understood as that arising from the models themselves, and that arising from the data used in the models. The analysis here
concentrates on data uncertainties (empirical uncertainty), which can be quantified in a probabilistic uncertainty analysis.
Our uncertainty analysis estimates the uncertainty of the main data inputs into the NAMEA-model, and propagates these to the output using a probabilistic simulation analysis. The uncertainties on the output
results are presented as coefficients of variance (CVs) as well as illustrative plots with confidence intervals (see Chapter 1).
Based on the research by Lenzen (2001), we identify the main sources of empirical uncertainty in the Danish economic input-output data to come mainly from three sources:
- Degree of aggregation (aggregation / allocation error)
- Geographical coverage (imports assumption)
- Age of the data
We thus find that these sources of uncertainty, together with uncertainty on the emission factors, will dominate the overall uncertainty, and that our analysis can reasonably be limited to these sources.
The first two sources are considered in detail, as there may often be a choice between using geographically relevant data at a low degree of detail (few industries), or using a table from another country,
available at a higher level of detail (many industries), see Chapters 2.8 and 2.11.4. In either case, there will be a certain amount of unavoidable uncertainty associated with using an IO-table constructed from
economic data a few years prior to the particular year of study (especially for rapidly changing industries). This temporal uncertainty has not been quantified.
Coefficients of variation (CVs) are estimated for the IO-data and the emissions data, with separate estimates for the domestic and foreign production. Our analysis does not include uncertainty for the final
use data and emissions, nor for the characterisation factors for the impact assessment.
The coefficients of variation estimates for the IO-data were made from two levels of aggregation analyses. The first of these (Chapter 2.11.1) looked at the variation within the Danish IO-table arising from
the aggregating the data in the supply-use tables (Danmarks Statistik 2003b) into the level of the 130 industries in the standard IO-table (Danmarks Statistik 2003a), and the second looked at various levels
of aggregation in different national IO-tables (Chapter 2.11.2). Geographical uncertainty was also analysed by comparing different national IO-tables at the same level of aggregation (Chapter 2.11.3).
Coefficients of variation were also estimated for the emission data. The CV estimates for the industry emission data are based on the values published with the Danish emission data, while CVs for those
emission types for which uncertainty data is not published are based on known variations in the processes underlying the emissions (see Chapter 2.11.5). For foreign emissions, these data were supplemented
by an analysis of the geographical variation between the air emission data of various countries (Chapter 2.11.6).
2.11.1 Uncertainty due to aggregation in the Danish IO-table
The most detailed transaction records complied by Statistics Denmark consists of a total of 51014 inputs and outputs classified by 7-digit commodity numbers (Danmarks Statistik 2003b). From these
detailed transaction records, we constructed 130×1,878 matrices for Make and Use. These two matrices were used to derive the most detailed commodity-by-commodity input-output matrix for Denmark
based on the industry-technology model (see Suh 2003b). The resulting matrix has the dimension of 1,878×1,878. The detailed square matrix was further aggregated into a 1,878×130 matrix (A’) based on
the standard classification system used for the Danish input-output table. The matrix shows the structure of inputs, represented by the most detailed commodity categories, to commodities, represented by the
standard Danish IO categories. In principle, aggregation of the A’ matrix into a 130×130 matrix would result in the standard IO-table (A), as produced by Statistics Denmark (Danmarks Statistik 2003a).
The aggregation error that we estimate is that arising when using the aggregated input coefficients of those commodities that belong to the same standard Danish IO category (elements in A) instead of the
most detailed, commodity-specific input-coefficients (elements in A’). The probability that a commodity in the detailed classification is chosen over the others in the same standard IO category is assumed to
correspond to the size of the input. Thus, the matrix of Coefficients of Variation (CVs) is derived from the elements in each column in the A’ matrix that are used for the aggregation into the A matrix.
The CV estimates derived from the above analysis were used directly as an estimate of the uncertainty of the Danish industry matrix.
2.11.2 Importance of aggregation level across national IO-tables
National IO-tables are published at very different levels of detail, as can be seen by the following list of tables considered in this analysis. The number of directly-comparable commodity groups in each table
is given in brackets (the numbers in brackets are slightly less than the total categories published for each country, as a few categories are not comparable across the tables):
- USA (492)
- Norway (176)
- UK (138)
- Denmark (129)
- Netherlands (106)
- Germany (71)
- Sweden (55)
- France (40)
The aggregation structure of the 30x30 GTAP IO-tables used by Nijdam & Wilting (2003) and Goedkoop et al. (2003) is also considered in our analysis, but the data were not used in the quantitative
analysis that follows.
To analyse the degree of aggregation across the tables, we organised the industries / commodity groups considered in each table according to the CPA 2002 (Statistical Classification of Products according
to Activity). This is a fairly straightforward procedure for the European tables, but creates some difficulties for the USA table which uses a different classification system, the Standard Industrial Classification
System or SIC. Once classified according to a common system, the different levels of aggregation in the different tables can be analysed (i.e. the number of commodity groups considered by each table at the
different classification levels).
Twenty-three "top-level" groups of industries are defined, where the "top-level" index is taken from the CPA nomenclature, and corresponds to where at least one of the tables listed above is constructed at
that level of aggregation, e.g. at the CPA level "DA - Food Products, Beverages and Tobacco", the USA table is broken down into 50 different commodities, whilst the UK is broken down into 13 and
Germany 3. However the French table has just one entry, so this is taken as a "top-level classification". 2nd and 3rd level classifications are also considered where possible, e.g. at the CPA level 16,
"Tobacco products", the USA table has 4 entries, and all other tables 1 (except the French and GTAP tables, which only have entries at the higher aggregation level).
Even at very high levels of aggregation, the different classification system used for the USA table means that certain industries are left of the analysis. The French table also has a few commodity groups with
unusual combinations of the CPA classifications, which also results in them falling outside the "top-level" classifications (i.e. being excluded from the analysis).
We then calculated the degree of variation (expressed as a coefficient of variance; CV) between the use commodities for each IO-table at the various aggregation levels. Figure 2.2 gives the results of this
analysis for "Pulp, paper products and publishing". For comparison, the variation within the Danish supply categories is also given (plotted on the y-axis).
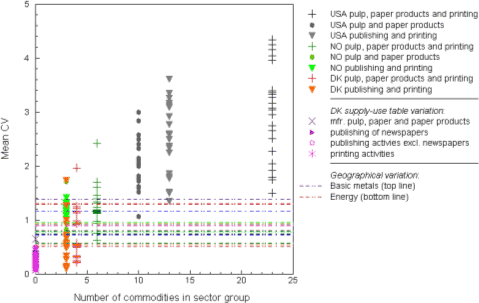
Figure 2.2. Coefficient of variance (CV) within industry groups as a function of the aggregation level (calculated by aggregating the commodities from the detailed tables from USA, Norway and Denmark to the levels of aggregation found in less-detailed tables), with the geographical variation superimposed as vertical lines showing the range in CV found for inputs to pulp, paper products and publishing between the tables, showing highest variation for Basic metals and lowest variation for Energy inputs.
Figure 2.2 clearly shows the higher level of variation as more commodities are aggregated. This effect is most visible with the USA table, as it has the largest number of commodity groups, however the trend
is also clear for the other tables where data allows.
2.11.3 Geographical variation in inputs to industries
The degree of variation between the tables was also assessed to give some indication of geographical variation. Although geographical differences are thought to be the primary driver for the variation
between the tables, the analysis in fact captures all sources of difference between the tables. The latest version of the IO-table is used for each country, so there are differences in the age of the data, and
perhaps even more importantly, there may be underlying differences in the methodology used to construct the different tables.
The analysis is also limited to comparing those commodities that are directly available at an identical level of detail for each table, i.e. Norway, Denmark, Germany and the Netherlands can be compared
according to "Beverages" (CPA 15.9), but not the USA or UK, which consider a lower aggregation level (e.g. "Bottled and canned soft drinks"), nor Sweden or France, which consider only a higher
aggregation (e.g. "Food products and beverages"). Variations are only calculated for those commodities considered by at least 3 tables, which limits the analysis to a consideration of only 77 commodities.
To be comparable with the previous analysis, the 77 commodities are then aggregated to the 23 "top-level" groups considered in the aggregation analysis (i.e. although the CV is calculated for each possible
commodity, the average of these CVs is calculated for the higher-level groupings). Variation of inputs within "Pulp, paper products and publishing" is shown in Figure 2.2 (CV of each industry group given by
a vertical line, with the industry showing the highest and lowest geographical variation given in the legend).
2.11.4 Aggregation vs. geographical uncertainty
Figure 2.2 clearly shows that geographical variation between the IO-tables is often considerably lower than the variation due to aggregation of industries / commodities. This same trend was found for almost
all of the 23 industry-groups analysed. The analysis shows that where 10 commodities (and in some cases considerably fewer) are aggregated, these have higher variation between them than what is found
across the tables (at that aggregation level). Thus, from a data uncertainty perspective, it appears preferable to use a table with a low level of aggregation rather than using more geographically relevant data at
a high level of aggregation.
This supported our decision to model the products imported to Denmark on the basis of the detailed USA table, rather than using the more specific, but less detailed IO tables acquired for European and
non-OECD countries (see Chapter 2.8).
This interesting finding also means that when using a table with a high degree of detail but in a different geographical context, e.g. using the USA table to represent the rest of the world (ROW), the
geographical variation values can be used as a rough estimate of the uncertainty arising from using this data in a different context. The geographical variations calculated above are thus used as an estimate of
the uncertainty of the modified USA IO-table applied to model the products imported to Denmark (see Chapter 2.8).
In both the domestic and foreign IO tables, the row average CV is used to estimate the uncertainty in those few instances where lack of data results in a "blank" field. This approximation is made because
empty fields are invariably interpreted as zero, which is not meaningful in this context.
2.11.5 Emission factor uncertainty for Danish emissions
Uncertainty estimates are available in various reports on the Danish national emission inventory (see Nielsen & Illerup 2003; Illerup et al. 2003a and b). The uncertainty estimates are derived using the
methodology of Pulles & van Aardenne (2001), and are expressed as half of the 95% confidence interval divided by the total (interpreted to be the mean). The uncertainty estimates combine the uncertainty
of the data source (national statistics) and the uncertainty of the emission factor. The former is estimated by Illerup et al. (2003) as contributing only little to the overall uncertainty (2% for most emissions),
whilst the latter range considerably (as high as 1000% for ammonia and heavy metals). The level at which the uncertainty estimates are available depends on the emission type, for example for carbon
dioxide, the estimates are for each fuel type, whilst for many emissions it is at the aggregated industry level (i.e. transport and stationary consumption, which is sometimes further disaggregated into energy and
transformation industry, non-industrial combustion and manufacturing industry). Estimates for fugitive emissions and those from various agricultural processes are also available for certain emission types (e.g.
methane and ammonia).
Using information on the breakdown of fuel types consumed by the various industries, the approximate relative contribution of transport and stationary consumption activities to the emission can be estimated,
and an overall CV calculated for each industry from the available uncertainty estimates (or from the contribution of each fuel type, in the case of carbon dioxide emissions).
This simple method does not take into account the fact that much of the uncertainty is correlated, i.e. arising from a common source, with its distorting effect on the output uncertainty sample (when calculated
using a probabilistic simulation analysis). However, this effect can be mitigated by basing the results on an analysis on the normalised difference between the industries, which removes the distorting effect of
common elements (provided they are calculated from the same uncertainty sample). For simplicity and consistency, the CV estimates are once again calculated at the level of 23 industries.
Uncertainty estimates for emissions to water and soil are specified in considerably less detail than emissions to air. We have either based these on expert knowledge, where a single estimate is used across all
industries (e.g. nitrogen emissions to water are estimated at 10%, representing 2 standard deviations), or calculated them from known ranges for the particular emission (e.g. the 4-86 kg range given for
mercury emissions to water from the extraction of crude and natural gas is assumed to cover the 95% confidence range).
We did not include uncertainty estimates for the land use data, and consequently uncertainty has not been estimated for the impact category “Nature occupation”. The land use data for Denmark is expected
to have very low uncertainty. However, no information was available to guide an estimate of using the Danish data as proxy for foreign land use (which is expected to imply a larger higher uncertainty).
2.11.6 Emission factor uncertainty for foreign emissions
The CVs for the foreign emissions (i.e. those associated with imports) are derived from the geographical variation between the NAMEA air emission inventories (Pasquier 2001). Sufficiently consistent air
emission data and economic data are available for the following countries to allow for an analysis of the degree of variation between the emission inventories (data for 1999, unless otherwise indicated):
- Denmark
- Greece
- Spain
- Finland (2000)
- Sweden
- United Kingdom
- Luxembourg (1998)
- Portugal (1997)
- The Netherlands
- Germany
- Norway
- Austria (1997)
- Belgium
To allow for an equal analysis of emissions per industry, we normalised the emissions to the total output for the industry in 1999 US dollars.
CVs for the air emission data are predominantly calculated using only the data from the first six countries listed above, as recommended in Pasquier (2001), which cautions that the data from all available
countries cannot reliably be compared because of methodological and data collection differences. However, CVs are only calculated where three or more data points are available, so in a few cases (e.g. for
particulates) the data for all countries other than Belgium are used (Belgium is excluded because of highly anomalous results). This restriction also means that the CVs are calculated at fairly high industry
aggregation levels, since for many of the countries, the emission data is only available at a relatively high level of industry aggregation. For consistency, in most cases the CVs are calculated at the same level
of aggregation as the economic data (23 industry groups), and this CV used to estimate the uncertainty of the emission for all industries in the group.
When the geographical variation data gave lower uncertainty estimates than that estimated for the Danish data, the Danish CV value was used for both domestic and import emissions. In these cases, the
overall uncertainty is assumed to be dominated by the very high uncertainty of the method used to calculate or estimate the emissions (e.g. as found for emissions of heavy metals). The same applies to
emissions to water and soil where a single estimate based on expert knowledge is used across all industries.
2.12 Adjusting for differences in improvement options
The standard NAMEA expresses the magnitude of the environmental exchanges from each industry and product group, not the possible change in these exchanges (e.g. improvement potential). The product
groups that have the largest environmental exchanges may not necessarily also be the ones with the largest improvement potential.
We therefore investigated different data sources on improvement potentials (including BREFs from EU, BAT documents for the Nordic Council of Ministers, BATNEEC guidance documents from Ireland,
and Cleaner Technology projects of the Danish EPA) to identify possible differences that could affect the prioritisation (see also Chapter 1.7). To limit the analysis, we addressed only the product groups
with the largest environmental impacts (Top 20).
The rationale behind this approach is the assumption that unless specific circumstances apply, all industries (product groups) have a relatively similar potential for improvements, which implies that the
industries / product groups with large impacts also have equivalently large improvement potentials based on the general results of increased efficiency and incremental technology improvements characteristic
of the market economy. Limited improvement potentials would especially be expected for in the case of mature, and well-established technologies, on the last, flat part of the learning curve, if at the same
time competition from new technologies were constrained – either by the lack of economic incentives or by physical, cultural or political limitations.
Limited improvement potential can also be identified by relatively small differences between best available, modern and average technologies.
We found large improvement potentials for all the priority product groups, generally falling within the following categories:
- Substitution of chemicals, e.g. antifouling (TBT and copper), pesticides, solvents and heavy metals,
- Substitution of energy sources from fossil fuels to renewable energy,
- Substitution of raw materials, e.g. new protein sources for animal feed, new materials instead of metals,
- Recycling and biological extraction of metals and containment of mining effluents.
Phylipsen et al. (2002) also conclude that “traditional” material technologies, such as more efficient material production, material-efficient product design and material recycling are still important options to
reduce the environmental impact in each of the impact categories of most of the materials they investigated.
For detailed results on improvement options for the top-10 product groups, see Chapter 1.7.
We have not found any evidence that any of the priority product groups should be particularly subject to limitations in their improvement potential relative to other product groups. The differences appear to
have more to do with the focus that have been placed on different industries or environmental problems. Some industrial industries and product groups have been subject of cleaner production projects,
development of BAT notes etc., so technological options have been identified, but in general only applied to a limited extent. In other areas, international agreements are already in force or being negotiated,
i.e. both needs and options have been identified, but policy measures and instruments still lack full implementation.
Experiences from the cleaner technology programmes especially in Denmark, the Netherlands and Germany (Remmen 2003) show that public funding do promote improvements by higher efficiency or
substitution of substances, materials or processes, but within the regime of the options of the technological development in general. As these funding programmes can only support a very limited number of
industries, general improvements will require industry-wide incentives, e.g. use of legislative restrictions or economic incentives, such as taxes.
In general it can be concluded that a focus on improvement options does not change the overall ranking of product groups compared to the focus on environmental impact applied for the current
prioritisation. We have therefore not found any reason to quantify the improvement potentials further.
2.13 Calculation routines and validation
To arrive from the expanded NAMEA to the results presented in Chapter 1, the environmental impact intensity (i.e. the “life cycle” or “embodied” environmental impacts per monetary value of products) of
each industry is first calculated by multiplying the emissions or impact matrix with the inverted production matrix. This provides the exact solution to the iterative calculation suggested by figure 2.1, and is
equivalent to the standard calculation routine for LCA and IO-analysis, as described by Heijungs & Suh (2002).
The environmental impact intensity of each industry is then multiplied by the monetary requirements from each final consumption group (product group or household activity) and by the export value of each
industry, resulting in the total environmental impact from each product group, household activity or export item.
The calculation routine was performed by exporting the expanded NAMEA from the Excel work book in which it was stored into three different software, namely MatLab (www.mathworks.com), SimaPro
(www.pre.nl) and Analytica (www.lumina.com) and performing the above calculation in parallel in the three software. The calculation was validated by ensuring that the results were the same across the three
software. Calculations of coefficients of variance for the results were done exclusively in Analytica, using a probabilistic simulation with Median Latin Hypercube sampling of the input distributions. The very
large number of inputs into the uncertainty model forced us to restrict the simulation to a sample size of 200. This relatively small sample size is deemed sufficient for this application (i.e. it gives reproducible
CV results), but the results should not be interpreted beyond two significant figures.
Footnotes
[3] 1,3-Butadiene is a product of incomplete combustion and mainly found in exhaust emissions from gasoline- and diesel-powered vehicles, non-transportation fuel combustion, biomass combustion
(CMEH 2000, EPA 1996) and cigarette smoke. It is also an industrial chemical produced in petroleum processing and mainly used in the production of synthetic rubber, but is also found in smaller amounts
in plastics and fuel. Exposure may therefore occur from polluted air and water near chemical, plastic or rubber facilities and from ingestion of foods that are contaminated from plastic or rubber containers,
although workplace exposure is more important (USDLSHT 2002). Industries with a high potential for exposure include rubber and latex production, petroleum refining, secondary lead smelting (due to the
plastic separators and rubber casings), agricultural fungicides, and production of raw material for nylon (USDHHSPHS 2002).
[4] N-butyl alcohol (also known as 1-butanol) is used as a solvent, dehydrating substance, adjuvant for agricultural chemicals, in hydraulic fluids and as an intermediate in chemical and pharmaceutical
manufacturing. It is used in many different industries, including manufacture of detergents, dyes, lacquers, fats, resins, waxes, gums, rubber, fabrics, ore floatation agents, and safety glass.
[5] Formaldehyde is emitted to air from fuel combustion, mainly in vehicles, and biomass combustion (including cigarettes), from on-site industrial uses, and as an off-gas from products in which it is used,
notably particleboards, fabric and paper coating, insulation foams and as a preservative in some paints, coatings, and cosmetics (Environment Canada 2001).
[6] In fact, NOx dominated the original top10 due to a high characterisation factor for human toxicity soil. This is regarded as an artefact due to a very simplified fate modelling (Hauschild & Olsen 2004) and
this characterisation factor has therefore been omitted both from the top10 and from the version of the EDIP method used in this project.
[7] The very simplified way pesticides are treated in the Danish normalisation data implies that pesticides are not assigned any characterisation factor for water ecotoxicity (see Stranddorf et al. 2001).
| Front page | | Contents | | Previous | | Next | | Top |
Version 1.0 February 2005, © Danish Environmental Protection Agency
|