| Front page | | Contents | | Previous | | Next |
Reducing Uncertainty in LCI
2 Identifying the most important uncertainties
2.1 Uncertainties in identifying the correct processes to include
2.2 Uncertainty from technological mismatch between desired and available data
2.3 Uncertainty inherent to the available data
In a life cycle assessment, the overall system studied is the difference between the product systems that substitute each other. Thus, the largest uncertainties are likely to be found in relation to the processes that contribute the most to the differences in environmental exchanges between the product systems. These important processes can be identified and ranked by subjecting the initial system model to an error analysis. The initial system model is based on readily available data and order-of-magnitude estimates. An error analysis identifies and ranks the relative contributions from each process in the model to one or more summary indicators for the environmental impact. A process may be important because it has a large product flow relative to the functional unit (i.e. makes up a large part of the product system) or because its environmental exchanges are large relative to the product flow. The fewer steps between a process and the process in which the reference flow occurs, the more important is an uncertainty on the product flow, since this uncertainty will affect all processes further up- or down-stream.
The uncertainty of a less important process is only relevant if it is so large that a worst-case estimate would shift the process from being less important to become more important, i.e. to contribute to a significant part of the total environmental exchanges.
For a specific process, the sources of uncertainty can be divided in three:
- Uncertain identification of the process as the one to be included in the product system. This may mean that completely different processes are to be included, and is thus a major source of uncertainty.
- Technological mismatch between the desired data and the available data. This may mean that data have to be extrapolated from data representing a different technology, with different environmental exchanges. The resulting uncertainty decreases with decreasing difference between the desired and the available data.
- Uncertainty in the available data as such. This is the least important of the three sources of uncertainty, since it involves only uncertainty within the process in question, and not across different processes.
These three sources of uncertainty are analysed in more detail in the following three sections.
2.1 Uncertainties in identifying the correct processes to include
The procedures for identifying the correct processes to include in the studied product systems are described in the guideline “Geographical, technological and temporal delimitation in LCA” (Weidema 2002a) and the report “Market information in life cycle assessments” (Weidema 2002b). These procedures rely on market data, in which the following uncertainties are of importance: Uncertainty re. the scale of change that may influence the boundary conditions of the market. Uncertainty re. what intermediate products may substitute each other in different market segments and geographical markets. Uncertainty re. the temporal and geographical boundaries of the actual market of an intermediate product. Uncertainty re. what technologies and processes are constrained in their ability to change their volume in response to a change in demand. Uncertainty re. market trends. Uncertainty re. the parameters that influence decisions on capacity adjustment, e.g. prices of different technologies and the effect of information on buying behaviour and investment decisions.
These uncertainties are all of major importance, since they may affect which processes are included and excluded from the analysed product systems. The importance increases in proportion to the possible variation in the technologies and processes that ma y be substituted, i.e.: Variation in the relevant technologies and processes between different possible markets. Variation in the relevant technologies and processes within the same market, especially the variation between the least and the most competitive technology/process. This means that the higher the variation in possible outcomes, the higher is the demand on the quality of the market data.
When relevant, several alternative scenarios should be included to reflect the limits of knowledge.
The mentioned major sources of uncertainty also apply to the handling of multi-functional systems, following the procedure described in Weidema (2001, 2002a, b). Additionally for this procedure, the following minor, technical sources of uncertainty may be considered, when relevant: Uncertainty of identifying the limiting parameter for a combined production. Uncertainty of identifying the split-off point and the point of displacement.
2.2 Uncertainty from technological mismatch between desired and available data
The possible mismatch between the desired data and the available data is illustrated in figure 1, where the boxes A, B and C illustrate available data, which are:
A. too specific data from within a desired population, B. less specific than - but including - the desired data, C. for a product/material, process type, area/location or time period not including the desired data, and which differs more or less from the desired.
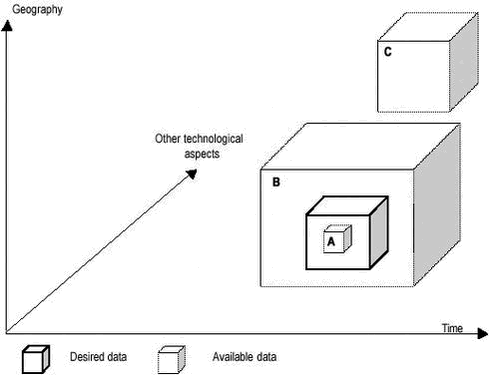 View the image in full size
Figure 1. Illustration of different kinds of "technological mismatch" between desired and available data.
The three dimensions of technology in figure 1 are those typically used to establish whether a specific data set is adequate to meet a specific data requirement in a life cycle study (Weidema & Wesnæs 1996, Weidema 1998): Temporal aspects: Differences depending on the period that the data is assumed to represent or for which data is collected, since technology changes over time. Geographical aspects: Differences depending on geographical location of the process. This may be caused by differences in natural conditions (as defined by climate, landscape, soil etc.) or administrative conditions (between country-groups, countries, states, counties). Other technological aspects of the data set, which may be further subdivided into: Structural aspects: Differences depending on the composition of the products from different processes within the same process class (as defined by CPA-code or more detailed classifications; see Annex A). These are often named ’structural’ differences, because they depend on the structure of the product composition within each process class. An example is aggregated data for steel production, which may consist of different amounts of recycled steel, steel that has passed through different amounts of finishing processes, and include a number of specialised steel types. The difference between data may simply be caused by differences in how much recycled steel, how much finishing, and how much of the different specialised steels are present in the different aggregated data. Differences among individual production plants at a given point in time and within a given geographical region: This is differences depending on e.g. capacity utilisation, age of installed technology at the given point in time, management factors including education, scale of plant, and effectiveness of (emission) control.
To some extent, a hierarchy between the different aspects can established (see Figure 2):
Some (but not all) of the variation at plant level may be explained by structural differences in product outputs, or by differences in administrative or natural conditions. Some (but not all) of the structural differences in product outputs may be explained by differences in administrative or natural conditions. Some (but not all) of the variation between administrative regions may be explained from differences in natural conditions. Furthermore, temporal variation (changes over time) may affect all of the other aspects, but is most important at the plant level and of least importance at the level of natural conditions.
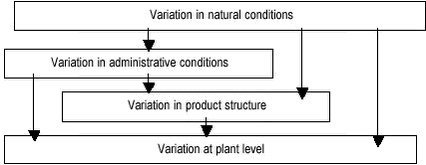
Figure 2. A hierarchy of causes of geographical and technological variation. Please note that variation at a lower level is not solely caused by the variation in the levels above.
This also implies that the total variation ast plant level may be divided according to the different causes as shown in table 1. In addition, temporal variation may play a role when the actual temporal position of the process is uncertain or when applying data from different periods.
Table 1 Classification of the causes of geographical and technological variation. Please note that the examples given under each heading are not exhaustive.
Variation between natural regions |
Climate Landscape Soil type Density of population Raw material quality and availability |
Variation between administrative regions |
Raw material price Labour costs Legislation/regulatory differences Available capital |
Variation in process or product structure (structural variation) |
Residual variation at plant level |
Capacity utilisation Age of installed technology Management factors, incl. Education Scale of plant Effectiveness of (emission) control |
Figure 3 illustrates some of the underlying causes of variation listed in table 1 and how they may be connected more or less to one or more of the 3 dimensions of figure 1.
If we can determine the contribution of each of these underlying causes to the variation in each of the 3 dimensions, we would be in a much better position to estimate the overall variation. If we could furthermore find some small parts of our 3-dimensional space, where an adequate number of measurement points actually is available, we could calibrate our estimates and see how large a residual is not explained by the identified causes (the causes listed in table 1 and figure 3 are not exhaustive).
In Annex A, a first attempt at obtaining such estimates is made on the basis of a theoretical-empirical analysis of underlying causes of uncertainty. The recommendations in the following text are based on the conclusions of this analysis.
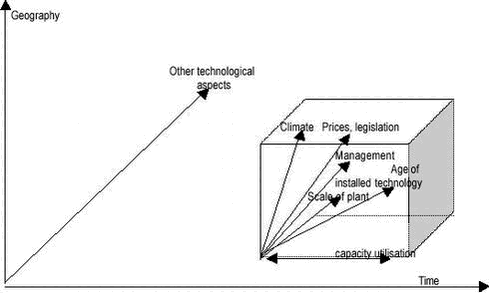 Figure 3. Some causes of variation and their relation to geographical, temporal and other technological aspects.
In general, among the causes of variation mentioned in table 1, the most important one is variation in process or product structure between related processes (structural variation).
Of the geographical causes, practically all sectors are affected by differences in:
- legislation and/or regulation,
- raw material quality and availability,
- availability of investment capital,
- culture, labour costs and educational level.
In addition to these causes, certain sectors (e.g. agriculture, building and transport) are especially susceptible to differences in:
- climate,
- landscape,
- geology, and
- population density.
Differences in legislation/regulation are of largest importance for emissions, while the other causes affect both energy and material consumption and emissions. Nevertheless, emissions are generally affected more than energy and material consumption.
Besides the structural and geographical differences, age of technology appears to be the most important cause for variation at plant level, with management and plant size as other important causes. Capacity utilisation is often of less importance (with transport as an exception). Management is of larger importance for emissions than for material and energy consumption. Emission control equipment and its efficiency is an important cause for variation in emissions.
Besides the above, it is not possible to draw general conclusions about the importance of the different causes, because of the large differences between sectors. For one sector, raw material quality may be a dominating cause, while availability of capital may be the most important cause for another. Even within sectors, such differences exist.
However, a general observation can be made concerning the reasons why sectors are different in respect to importance of causes of variation: Issues that are generally important for the activity will also be important as causes for variation. For example, some sectors are more regulated than others, and differences in regulation thus becomes a more important cause of variation. In the opposite end of the spectrum, agriculture and household processes can be mentioned as examples of processes where detailed regulation is difficult to apply and/or enforce. In a similar way, raw mate rials may play a minor role in some sectors (as shown by the ratio of the production value to the raw material costs), and variation in local raw material availability may thus play a minor role for such sectors. Similar arguments can be made for availability of capital, and labour costs. The obvious importance of natural geography for some sectors (and the consequent minor importance for the remaining sectors) has already been described above. The importance of age of technology depends on the speed of development of the process. For example, in the wood products industry, sawmills are generally slow in development due to lack of capital, while the wood panel industry develops more quickly. The result is a much lower variation in energy consumption (+/-10%5) in the latter industry compared to the former (+/-40%).
Thus, using table 1 as a general checklist and asking for each item: “How large a role does this item play for this activity in general?” will allow a quick identification of the most important causes of variation for a specific activity. Once the most important causes are identified, the further uncertainty analysis can focus on quantifying these causes, which will dominate the overall uncertainty of the activity.
If a desired average is not available, but data is available on a smaller part of the population of interest (situation A in figure 1), the desired average may be estimated from this smaller part. If only one single data value is available, and nothing else is known about the population, the best estimate is that the available data represents the mean value and that the uncertainty is of the same size as in other similar populations. Examples of the size of uncertainties of different populations show coefficients of variance ranging from 5% for large populations over 10-30% for specific energy data to the more extreme 60-150% typical for many emissions. Besides population size, the size of the uncertainties depends on the extent to which the variation is controllable (or controlled). The large coefficients of variance obviously reflect technological differences within the population. In a homogenous population, the desired average can be determined as the mean of the sample, but the uncertainty of the estimated average depends on the sample size. The larger the sample, the more likely it is that the sample is a good estimate of the population. If you have only few data, the standard deviation of the average is ½ the range of the sample, but if you have 10 data the standard deviation is 1/3 of the range of the sample. If you have 30 data, the sample is usually regarded as a good approximation of a full, homogenous population. However, the populations studied in life cycle assessments are seldom homogenous, and extrapolations from a sample to a larger population must therefore take into account all the issues described above.
If the desired data are not available, but you have average data for the larger population of which the investigated process or population is a part (situation B in figure 1), this average may be used to estimate the desired data. The variation on the average is an expression of the probability distribution for the desired data. The resulting smaller population will have a larger relative uncertainty than the large population of which it is a part. The typical uncertainty on specific processes and small populations can be deduced from the information given above and in section 2.3.
When the desired data are not available, but data are available for another geographical region, extrapolation may be relevant (situation C in figure 1, covering both geographical, temporal and other extrapolations). To judge the error that may be introduced, the following rules of thumb may be applied:
- Extrapolation from one geographical region to another will typically involve some additional variation because of subtle differences in culture, education levels or labour costs. To consider this, the coefficient of variance of the original data should, as a minimum, be increased with 10%.
- Special precautions must be taken when extrapolating:
- from regions with high availability of investment capital to regions with a low availability,
- from regions with different population densities,
- emission data from regions with different legislation/regulation,
- from regions with different geological conditions e.g. reflecting itself in differences in raw material quality and availability,
- agricultural data and data on buildings from regions with different climatic conditions,
- transport data from regions with different landscapes.
In these situations, the specific influence should be investigated in each individual case.
Averaging data over time should not exceed that which is necessary to even out seasonal fluctuations. When using older data to estimate the desired (newer) data, the attention should focus on:
- the possible influence of changes in product mix over time, which may not be obvious from the available data,
- shifts in technology that may cause the old data to be completely obsolete and misleading,
- the speed of development of the sector, which can be used to estimate the necessary correction factors used in the extrapolation.
When it is known that the basic technology remains the same over the period, extrapolation can be based on:
- expressed political targets, e.g. for reduction of specific emissions or reductions in energy use,
- knowledge on efficiency improvements over time.
For further recommendations on forecasting, see Weidema (2002b).
Extrapolation of data from related processes or products is only relevant for activities that are very closely related. This may be the case if the same product is produced with the same technology and under the same conditions at different plants. However, even processes that seem very closely related might in fact be quite different. Even between quite similar activities, extrapolation may still involve additional uncertainty, mainly due to:
- differences in size (as a default, the coefficient of variance should be increased by 10-20%),
- differences with respect to management (for emissions, the coefficient of variance should be increased by minimum 10%, while for energy consumption, the coefficient of variance should be increased by minimum 2%).
2.3 Uncertainty inherent to the available data
The major causes for uncertainty within a specific (available) dataset are similar to those causing uncertainty between different processes, as described in section 2.2, and the dominating cause of uncertainty can be identified in the same way, i.e. by using table 1 as a checklist.
If the available data is an average, its uncertainty can be expressed in terms of the variation of the population in question around its average value. A dataset covering a larger group of processes, a larger geographical area or a larger time span will obviously have a larger absolute uncertainty than a more specific dataset. However, the relative uncertainty will typically be lower, the larger the population. With increasing sample size, the variation increases, while the relative uncertainty decreases, since it is more likely that the sample is a good estimate of the population.
When individual data are not available from which the uncertainty can be calculated, a default coefficient of variance of 5% may be applied for national averages. Larger uncertainties should be assumed if the population is small, i.e. if the specific unit process occurs only in a small number within a given country, or if the population is inhomogeneous, i.e. if it includes processes that applies different technologies or have different product mixes.
The smallest uncertainties are generally found for raw material consumption and energy use, and the largest for emissions.
The uncertainty related to emissions tend to fall in four distinct groups:
- Emissions that occur as a result of substances present in fuels or raw materials, e.g. carbon (C) in fossil fuels, which is primarily emitted as CO2 in known and fixed proportion to the amount of fuel used. For this type of emission, the uncertainty is obviously of the same order as for the fuels and raw materials in which they occur, i.e. below 20% and in the order of 10%.
- Emissions that occur as a result of substances present in fuels or raw materials, but which can be reduced by cleaning of the exhaust gases e.g. sulphur (S) in fossil fuels. For this type of emission uncertainty can be significant depending on the type and efficiency of the cleaning technology used, especially if the data covers geographic areas where regulations regarding emissions are different. If not, the uncertainty will often be of the same order as above.
- Emissions that are created during the production process, and which vary significantly depending on the physical conditions during production, e.g. the
amount of CO and NOx created during combustion of fossil fuels,which depends on temperature, the amount of oxygen present etc. These emissions may vary with a factor of 5-10. The same is the case for emissions that occur from the use of specific chemicals during the production process. In this case, the emissions will usually be highly dependent on the specific production process, which generally leads to significant uncertainty.
- Emissions that occur as a result of substances present in fuels or raw materials, and which naturally vary significantly, e.g. cadmium, lead, mercury and other metals in coal and crude oil. In extreme cases, this type of emissions may vary with a factor of 1000 or more.
When using averages, both national averages, averages over industrial sectors, and averages over time, it should be remembered that although the uncertainty on the average is low, the underlying processes might still have a large uncertainty. This means that the average with its low uncertainty should only be applied as such, when this is actually the desired data. If used to estimate a smaller part of the population or other data, larger uncertainties will be involved as described in section 2.2.
If the available data is site specific, the inherent uncertainty of a specific data set is typically low. If no information on uncertainty is available, the following default coefficients of variance can be applied:
- for energy consumption: 1%
- for material consumption: 2%
- for emissions: 10%
These defaults reflect general measurement uncertainty. Energy consumption is typically measured continuously, while material consumption is typically registered by weight or volume and may be subject to errors in estimating stocks, concentration, water content etc. Continuous measurements of emissions are seldom, which is the reason for the much larger coefficient of variance suggested. Some emissions may be better monitored and this should be reflected in the applied coefficients. Other emissions (and even auxiliary materials) may be estimated or roughly calculated, which should result in larger coefficients of variance.
Besides measurement uncertainty, site specific data may be subject to uncertainty stemming from implicit or explicit allocation procedures. If the data represents an activity with several products, of which only one is of interest for the life cycle study in question, allocation procedures may be applied to arrive at the data for this product. Such allocation procedures may not always be explicitly reported, since they may be regarded as obvious or implicit, e.g. the allocation of a joint raw material over all produced items by relative weight, the allocation of a surface coating over the relative surface etc. Nevertheless, such procedures may cause considerable uncertainty, especially if the process in question has a variable product mix. When assigning default uncertainties, the possible additional contribution from implicit allocations should be considered specifically.
The above described default measurement uncertainties do not apply to data that are interpolated from average data or extrapolated from older data, related activities or geographical regions. Uncertainty on such data was dealt with in section 2.2.
5 In this text, we generally use the +/- to describe a range covering 3 times the standard deviation.
| Front page | | Contents | | Previous | | Next | | Top |
|