| Bottom | | Front page |
Pesticides Research no. 94, 2004
Quantitative Structure-Activity Relationships (QSAR) and Pesticides
Contents
Foreword
Preface
Summary
Dansk sammendrag
1 Introduction
2 QSAR
3 Pesticides
4 Summary of conclusions
References
Appendix A
Foreword
The concept of similar structures having similar properties is not new. Already in the 1890's it was discovered, for example, that the anaesthetic potency of substances to aquatic organisms was related to
their oil/water solubility ratios, a relationship which led to the use of LogP octanol/water as an estimate of this effect. Today it is known that all chemicals will exhibit a minimum or “basal” narcotic effect
which is related to their absorption to cell membranes, and which is well predicted by their lipophilic profile.
The use of logP alone can thus explain about half of the toxicity (R²=0.5) of unrelated industrial chemicals to fish, and with closely related substances (such as linear alcohols or ketones) such simple models
are highly predictive. More reactive chemicals (“polar narcotics” such as phenols and amines) can also be modelled successfully in this manner. In all, approximately 70% of industrial chemicals fall into one
of these two general categories where aquatic toxicity estimates can be expected to be within an order of magnitude.
Other parameters such as molecular indices, quantum mechanical properties, shape, size, charge distributions, etc., can greatly improve estimates, particularly for substances which also act via highly reactive
toxic mechanisms (such as allylics, or acrylates).
The case is not quite as simple for substances with “specific” activities (pesticides or drugs). While simple narcosis will also be present for such chemicals, this may be of little interest compared with intense
activity induced by binding to a critical receptor site. This and other factors has resulted in considerable effort by, among others, the drug industry to develop tools which can better predict effects based on
structural information.
Today numerous computerised systems exist for predicting a large range of effects stretching from biodegradability to cancer. These include fragment based statistical systems such as TOPKAT and
MCASE, as well as three-dimensional modelling of ligand docking (COMFA). Some are well suited for screening of large numbers of chemicals, while others are very labour-intensive and best confined to
small closely related data sets.
Predictive ability will vary depending on both the method used, and the endpoint in question. In general, estimates of environmental effects have been more readily accepted than estimates of mammalian
effects. This may be changing rapidly. In general, predictive ability of sophisticated contemporary QSAR systems can often correctly predict the activity of about 80% of the chemicals examined, provided
that sufficient biological data exists to cover the domain of the structures. While this may not be good enough for some regulatory purposes, in others it may be sufficient. Even today a great number of
chemicals are never synthesised because the potential producer has already determined that they are likely to have harmful properties according to a QSAR estimate.
Jay Russel Niemela, Chemical Division.
Danish Environment Protection Agency.
Preface
This study considers the current available knowledge on the use of Quantitative Structure-Activity Relationship (QSAR) models relating to the use on pesticides.
The use is based on evaluation of the correlations between experimental values and QSAR estimates from the selected QSAR models. The experimental values are obtained from Pesticide Manual (Tomlin
1994, 1997), Linders et al. (1994), and from letters of approval for plant protection preparations containing data from studies submitted by manufacturers to the Danish Environmental Protection Agency
(Danish EPA) and evaluated in internal reports (Clausen 1998).
QSARs are quantitative models seeking to predict activity such as environmental toxicity derived from the molecular structure. Most often this is accomplished by first correlating properties such as
physico-chemical parameters with molecular structure and then correlating toxicity with these parameters. The central paradigm underlying such QSAR modelling is the structure-property similarity principle.
This paradigm states that analogous structures have generally similar properties. Since chemicals with similar properties tend to have similar biological activities, toxicity may be predicted from structure alone.
More than six million chemical substances are known and humans are exposed to 50,000 to 100,000 of them. As it is impossible to test each substance in a time and cost effective manner, this “guilt by
association” approach provides a powerful alternative to direct testing for predicting toxicity for untested substances. While QSARs in environmental toxicology were reported as early as 1869, modern
efforts have their roots in the classic turn of the century work of Meyer and Overton on narcotics.
Narcosis is the reversible state of arrested protoplasmic activity. It is a physical phenomenon, which is mostly independent of specific molecular structure. It is considered the most common mode of toxic
action, at least in short term exposure of aquatic organisms, and the mode of action for about 70% of the industrial organic chemicals. Narcosis is subdivided into several non-specific and specific
mechanisms (e.g. non-polar narcosis, polar narcosis etc.).
However, pesticides are developed specifically based on their specific mode of action mechanisms and this may affect the predictability of QSARs.
Based on the work already performed, the present report uses the most promising descriptors. As most of the preliminary work has been done on simpler molecules an evaluation at this stage may result in a
less promising result. However, it has been found reasonable to perform such an analysis to assess the current stage of the use of QSARs on pesticides.
Summary
In environmental risk assessment, information of environmental fate, behaviour and the toxicity of a chemical substance are of basic need. Quantitative Structure-Activity Relationships (QSAR) is a method to
derive certain effects or properties of chemical substances in the absence of experimental data.
For pesticides, the data requirements demanded for their authorisation normally means that sufficient data for a risk assessment exist. This is rarely the case for additives, impurities, degradation or
transformation products. Most QSAR models are developed from simple industrial chemicals and usually only a few pesticides are included. Especially, new pesticides consist of complicated molecular
structures acting with specific modes of action and their physico-chemical properties and ecotoxicological effect concentrations may not be estimated sufficiently close to the correct effect value by QSAR.
The current document presents the general framework in which QSARs can be used within the risk assessment procedure. Furthermore, it presents recommended QSARs for physico-chemical parameters
and ecotoxicological effect concentrations and performs an evaluation of their correlation with approximately 400 selected pesticides including salts and esters.
Of physico-chemical properties, the recommended QSARs to estimate the boiling point could not be evaluated due to lack of experimental values. Estimations of the melting point were inaccurate and mainly
overestimated by the presented QSAR. The solubility in water was reasonably well correlated with the recommended QSAR and a QSAR based on 322 pesticides was developed. The vapour pressure
QSAR based on suggested values from a computer model showed low correlation with the pesticides used. The Henry's Law constant was evaluated by comparing calculated values with QSAR predicted
values. A low correlation was observed. The octanol/water partition coefficient Kow derived by structure fragment analysis demonstrated acceptable agreement with the measured values. As the model was
a computerised version, a QSAR based on water solubility was suggested. Several QSARs have been developed to derive the adsorption coefficient Koc. Four recommended QSARs were selected for
comparison. The correlations between estimated and experimental Koc were acceptable for one of the QSARs. The QSAR for bioaccumulation was in reasonably good agreement with the experimental
values for pesticides.
For aquatic toxicity, QSARs have been developed for acute toxicity estimations for fish, daphnia and algae. The correlations between estimated and experimental EC50-values were low for fish, daphnia and
algae. New QSARs, based on experimental toxicity values and log Kow, were suggested following a grouping of pesticides into modes of action. It was observed that improvements of the predictability
could be obtained in some of the groups. However in other groups, the correlation coefficients were low and they could not be recommended except perhaps for screening procedures.
QSAR should not replace experimental values for pesticides. However, QSARs proved to have a reasonable predictive value and might be usable if no data were available.
Dansk sammendrag
Til risikovurdering er oplysninger om stoffernes opførsel og skæbne samt deres toksicitet nødvendige. Quantitative Structure-Activity Relationship (QSAR) er en metode til at skønne størrelsen af visse
fysisk/kemiske og toksiske værdier.
De dokumentationskrav, der forlanges ved godkendelse af bekæmpelsesmidler, betyder normalt, at tilstrækkelige data er til stede til en risikovurdering. Det samme er sjældent tilfældet for additiver,
urenheder, nedbrydnings- eller omdannelsesprodukter. De fleste QSAR modeller er udviklet ud fra simple industrikemikalier, og ofte er kun få pesticider medtaget. Da moderne pesticider ofte består af
komplicerede kemiske strukturer og er udviklet på basis af deres specifikke effekt, kan det medføre, at deres fysisk/kemiske egenskaber og toksiske effektkoncentrationer ikke kan skønnes tilstrækkeligt
præcist med QSAR modeller.
I projektet præsenteres områder for QSARs anvendelse, anbefalede QSAR modeller for fysisk/kemiske parametre og økotoksikologiske effekt-koncentrationer samt en vurdering af de anbefalede QSAR
modeller ved korrelationsanalyse med eksperimentelle værdier fra cirka 400 pesticider (inkl. salte og estere).
For fysisk/kemiske egenskaber blev det fundet, at den anbefalede QSAR model til skøn af kogepunktet ikke kunne vurderes på grund af manglende eksperimentelle data. Skøn af smeltepunktet var
upræcise og som regel over-estimerede med den anvendte QSAR. Opløseligheden i vand var til gengæld i god overensstemmelse med de målte data fra de anvendte pesticider. Damptrykket kunne ikke
estimeres særligt godt med den anvendte QSAR computer model. Henrys lovkonstant (H) vurderedes ved sammenligning med beregnede H fra eksperimentelle data og modelberegninger.
Overensstemmelsen var lille. Oktanol/vand koefficienten Kow beregnet ud fra en struktur analyse viste en rimelig overensstemmelse med de målte værdier. Da modellen kræver anvendelse af computer,
anbefales en QSAR model baseret på vandopløselighed. Der findes mange modeller til skøn af adsorptionskoefficienten Koc. Fire anbefalede modeller er anvendt i projektet, og den ene viste en acceptabel
korrelation til de eksperimentelle værdier. QSAR modeller for bioakkumulering i fisk viste god overensstemmelse med eksperimentelle data.
For toksisk effekt på vandlevende organismer er der udviklet QSAR modeller for fisk, dafnier og alger. Korrelationen mellem skønnede og eksperimentelle værdier var lav for både fisk, dafnier og alger.
Projektet har beregnet nye QSAR modeller baseret på pesticider opdelt efter deres virkningsmekanisme. Denne opdeling medførte væsentligt forbedrede korrelationer for nogle af de dannede grupper, mens
korrelationerne stadig var lave i andre. Der kan således ikke fremføres en generel anbefaling undtagen måske med forsigtighed i en “screening” fase. Studiet viste dog, at en opdeling efter stoffernes
specifikke virkning var en væsentlig forbedring fremfor anvendelsen af de mere generelle QSAR modeller.
QSAR modeller skal ikke erstatte eksperimentelle værdier for pesticider. QSAR modeller viste dog en rimelig skønnet niveau, hvis der ikke findes eksperimentelle data.
1 Introduction
The study on the relationships between molecular structure and physico-chemical and biological response, collectively known as Structure-Activity Relationships (SAR), is a rapidly growing field of research
in chemistry and biology. Some areas of the application of SAR include the design of more active and less toxic agricultural products (Martin 1978).
Basically, a SAR analysis consists of comparison between experimental values by mathematical variance analysis (e.g. regression analysis, discriminant analysis, factorial analysis and pattern recognition
techniques) and a selection of the best correlation values. The best-fitted correlations are then used to develop a mathematical expression to estimate end-values from known substances to unknown
substances.
When performing a SAR analysis, it is assumed that the chemical or biological response produced by a substance (usually an organic compound) is a direct function of its chemical structure, and that the
same substance will always produce the same response, under a given set of experimental conditions.
However, ”chemical structure” cannot be dealt with directly. Instead quantities, usually of a numerical nature, which are derived from and represent the chemical structures, are used. These quantities are
called molecular descriptors. The molecular descriptors are of various types:
- fragments (e.g. counts of atoms, bonds of various types, rings, ring atoms, molecular weight)
- topological (e.g. molecular connectivity, molecular symmetry)
- geometrical (e.g. molecular surface area and volume)
- physico-chemical (e.g. molar refraction, log Kow) or substructural (e.g. topological
- physico-chemical properties of substructures as embedded in the structure).
The more relevant to the chemical and to the observed responses the molecular descriptors become, the more exact the approximation will be and the more valid and useful the relationship will be.
Based on the work already performed on these initial analyses, this report uses the most promising descriptors. As most of the preliminary work has been done on simpler molecules, an evaluation at this
stage may result in a less promising result. However, it has been found reasonable to perform such an analysis to assess the current stage of the use of QSARs on pesticides.
The fast development in models (i.e. mathematical expressions) has resulted in a constant rewriting to include the most recent relationships during the processing of this report. The inclusion of QSAR in the
formal EU technical guidance document on risk assessment (TGD 1996) has made it imperative to present a report on QSARs and pesticides at this stage.
The statistical procedure used to derive QSAR models is linear regression analysis and it can be either single or multivariable depending on the number of structural descriptors used in a particular analysis.
The regression method affords transparent relations and simple mathematical equations and leads to quantitative correlations. However, for a successful and meaningful regression analysis, precise and
accurate input data are required (Karcher and Devilliers 1990).
It is important to keep in mind that the values used may be averages or
otherwise selected data and do not demonstrate the variation inherent in biological systems in contradiction to the precise estimates made from mathematical expressions. It is easy to become mesmerised by
the string of precise numbers being churned out by computers and to forget that the biological data going in are not anywhere near so precise (Dagani 1981).
It is important not to exaggerate the predictive accuracy of models, especially where the experimental data are either limited or controversial (Hart 1991). The weight in evaluation of QSAR results should be
placed on the level of magnitude and not the exact value which can only be established by experimental studies performed by internationally accepted guidelines. Different methods or guidelines for physical,
chemical and ecotoxicological tests can be used but priority to EU recommended methods is given in Commission Directive 92/69/EEC and 87/302/EEC (revision of Annex V in 67/548/EEC) or revised
versions e.g. OECD technical guidelines (OECD 1993),
Thus, QSARs can be used to assist data evaluation to contribute to the decision on whether further testing is necessary to clarify an endpoint of concern and to establish input parameters which are necessary
to conduct the exposure or effect assessment.
2 QSAR
2.1 QSAR method
2.2 QSAR modelling
2.1 QSAR method
Risk assessment
In environmental risk assessment, knowledge of the acute toxicity, chronic toxicity and environmental fate and behaviour of a chemical substance is a basic need. Factors affecting the environmental fate and
behaviour of a chemical comprise its water solubility, adsorption to soil and sediments, volatilisation, biotic and abiotic degradation, and bioaccumulation. Quantitative knowledge of these processes enables
one to model the concentrations of a certain chemical substance in the different environmental compartments (soil, air, water, and sediment). The knowledge of the toxicity of a chemical to aquatic organisms
is normally limited to simple effects as lethality, growth or reproduction inhibition. The effect concentration for acute toxicity is expressed as the LC50 (EC50), the aqueous concentration that produces 50%
lethality (and/or other effects). The effect concentrations for long-term or chronic effects is expressed as the NOEC, the highest test concentration with no observed effect on e.g. reproduction, population
growth or other kinds of sublethal toxicity. An approach towards the toxicity of a compound with regard to environmental risk assessment would be to determine a “safe level”, a concentration at or below
which, no organism or only a certain percentage of organisms in an ecosystem would be affected by the compound. Methods to predict the level of no-effect use the lowest acute or chronic values (TGD
1996) or interpolation of several values (e.g. Straalen & Denneman 1989, Wagner and Løkke 1991) and multiply with a relevant assessment factor.
Pesticides
For pesticides, the comprehensive data requirements demanded for authorisation normally mean that sufficient data for a risk assessment are present. This is not the case for the additives, impurities and
substances used in the formulation of the pesticide product and usually not the case for the degradation or transformation products from biocidal active substances. The research devoted to develop reliable
estimation procedures for the toxicity of environmental pollutants may therefore have a potential in estimating the needed data for the groups of substances. Today, the most promising technique for estimating
the toxicity of pollutants is QSAR. However, it should be noted that QSARs should be applied within its recognised limits of applicability, e.g. validity within a certain range of parameters (Kow-values, pH,
etc.), certain groups of chemicals (carbamates, phenylureas, triazines, etc.), or mode of action.
Structure-Activity Relationships
SAR is based on the knowledge that substances with a similar (analogous) chemical structure may have the same biological activity. SAR is a qualitative comparison of the structures of chemical compounds
and their effects in the biological system. From this evaluation of the influence of the chemical structure on the biological system, combined with experience in how changes in the chemical structure affect the
magnitude and type of biological effect, unknown toxic effects to the biological system of unknown compounds with related chemically structure are predicted.
Quantitative Structure-Activity Relationships
QSAR is a statistical data analytical procedure in which quantitative endpoints of compounds (e.g. toxicity) are correlated with one or more structural parameters of these compounds, normally through uni-
or multivariate linear regression (Chapman & Shorter 1978), non-linear regression (Könemann 1981), bilinear (Veith et al. 1983) or exponential regression. Commonly used structural parameters for
inclusion in QSAR correlations are for instance:
- octanol-water partition coefficients (log Kow)
- aqueous solubility (log S)
- Molar Refraction or Parachor (dispersion forces)
- dipole moment
- ionisation potentials
- molar volumes
- molar surface areas (Hermens 1989).
Several variables have been used in attempts to obtain the best-fitted parameter(s).
N-Octanol/water partition coefficient
The parameter n-octanol/water partition coefficient, Kow, is an experimental data describing the lipophilicity of the substance. It has been shown that a non-linear relation between biological activity and
lipophilicity exists. Substances of very low lipophilicity may be less able to pass lipidous membranes and substances with a high lipophilicity will accumulate in fat tissue and other lipophile phases and may
therefore not release a biological response.
Polarity
The polarity is an expression of the electronic distribution in the substance. The polarity is essential to the binding or release of the substance to an organism's membranes and/or macromolecules and thus
determines whether a biological response may take place or not.
Stereochemical structure
The stereochemical structure may influence the possibility of interaction with the macromolecules of an organism. Size and shape should be suitable to fit into the receptor or enzyme before biological action
may take place.
Scope
QSARs were originally mathematical models relating biological activity of chemicals to their structures and were developed and used mainly on the drug design area. Today, the scope has been broadened to
predict any kind of data related to both toxicity and exposure of chemicals i.e. the two categories of data that integrated together should permit the risk assessment of chemicals.
In ecotoxicology, QSAR models are used in the estimation of physico-chemical and effect related properties of chemicals in non-tested endpoints to assess if testing is needed or not.
QSARs are empirical models indicating that the results of evaluated studies are used in the further development of more precise models. The result of this iterative process is that QSARs change over time.
As an example of the scope of the structure-activity based modelling, the following parameters are considered:
- physico-chemical properties
- the partitioning of pesticides among environmental compartments
- bioaccumulation potential
- aquatic toxicity.
One of the main limits of applicability of QSAR is that relationships can only be established, and consequently used as a prediction tool, for compounds with a common mode of toxic action (e.g.
cholinesterase inhibitor or photosynthesis inhibitor). This is one of the major problems in the estimation of toxicity: What modes of action are recognised and how is a recognised mode of action assigned to a
certain compound, either inferred from measured data such as toxicity dose (concentration)-response (effect) curves, or predicted from structural parameters.
2.2 QSAR modelling
A QSAR model is a mathematical expression that relates the variation of the biological activity in a series of structurally similar compounds to the variation in their chemical structure. Thus, a QSAR model is
a mathematical equation describing the activity for a specific class of substances and derived from the quantified measured data belonging to these substances.
The strategy mainly rests on the concept that biological data measured for a few compounds selected may form the basis for a QSAR of a class. The developed QSAR models may permit the estimation of
the corresponding missing data for all the non-tested compounds belonging to the class, regardless of their number.
In order to validate the QSAR models, measured and predicted values are compared. The experimental values used in this report are mostly obtained from letters of approval or denial for sale or import
given according to the current statutory order from the Danish Ministry of the Environment where information from the applicants are evaluated by the Danish EPA's Pesticide Division. Other major sources
of information are the Pesticide Manual (Tomlin 1994, 1997) and Linders et al. (1994). The experimental values are compared by linear regression analyses with QSAR estimates derived from QSAR
models. The QSAR models used are the currently most preferred models.
The QSAR models or mathematical equations have been developed on the basis of experimental data on model substances. During the development of QSAR models, the calculations and testing were
performed by using a great number of substances, e.g. high production volume substances or other industrial substances. These industrial substances had mostly simple structures.
QSAR was previously used in the chemical industry in the development of new substances and only within the last decade the models have been refined to the use in assessment of chemical substances
effects, fate and behaviour in the environment.
The American Environmental Protection Agency (US-EPA) has developed a system of QSARs which are connected to a database (AQUIRE) and can therefore use the latest evaluated endpoint-values,
whether physico-chemical, effect or fate data. This should improve the models as the reliability of model estimations relies on the precision of the input data. The model system is called ASTER: Assessment
Tools for the Evaluation of Risk (Russom 1991, Pedersen et al. 1995).
The US-EPA has also developed a computer programme for estimating the ecotoxicity of industrial chemicals based on structure activity relationships: ECOSAR. The programme uses specific QSARs for
different chemical classes (US-EPA 1994). Because the programme was not complete at the time of this work, it was not used.
The value in using QSARs in the environmental assessment is that in the absence of experimental data employing QSAR may derive the missing variables. Besides, when several experimental studies on the
same chemical substance are giving information on single endpoints or parameters which are not complementary or in the same range, the decision on which results to use may be supported by QSAR (TGD
1996).
When applying QSAR, it should be taken into account that a QSAR is an estimation method and therefore, there is a certain probability that the estimate is poor even for well-evaluated models. QSAR
model estimates cannot be the only basis for preparing risk assessment. QSAR estimates should be seen as a complementary tool which, evaluated together with test results, can provide a more complete
understanding of the physico-chemical and ecotoxicological characteristics of the substance. This means that QSARs are no better than the data on which they are based. Furthermore, it should be noted that
QSAR models, generally, only exists for discrete organic substances and not for more complex substances or reaction mixtures.
Thus, QSARs can be used to assist data evaluation
- to contribute to the decision on whether further testing is necessary to clarify an endpoint of concern
- to establish input parameters which are necessary to conduct the exposure or effect assessment.
QSAR models should only be used in risk assessment if the models have been thoroughly evaluated and no experimental data or conflicting validated experimental data exist. As the work on QSAR model
development and evaluation is being performed in national and international programmes, the various models change currently.
Environmental risk assessment is based on a comparison of two variables:
- the concentration of the chemical in the environment (exposure)
- the concentration of the chemical at which no adverse effects on the environment are expected or estimated to occur.
Concentration in the environment
Measurements of the actual concentration in the environment are to be preferred. However, in many cases the concentration that can be expected after the release of the chemical in the environment
(exposure) is the most interesting issue. Fate modelling techniques may be applied to estimate these expected concentrations.
Fate models require an input of data for the various fate processes, e.g.:
- abiotic degradation (hydrolysis, photolysis, oxidation)
- biodegradation
- adsorption to soil, sediments, suspended particles
- volatilisation, evaporation
- leaching
- bioaccumulation
The rate or equilibrium constants of these fate processes can be measured in the laboratory and technical guidelines are developed to ensure comparable results (e.g. EU 1992, OECD 1993). To ensure a
comparable result that can be used in the risk assessment, the laboratory results are used instead of field data where factors affecting the results may be less controllable.
The fate processes determine the extent to which the organisms are exposed to the substance, i.e. the extent to which the chemical is bioavailable.
Several models are developed to predict/estimate the environmental fate processes and based on the physico-chemical parameters. Physico-chemical properties are important data for the exposure analysis.
Especially, a few physico-chemical parameters and variables are observed to be important, i.e.:
- the size and structure of the chemical
- the water solubility
- vapour pressure
- octanol/water partition coefficient (lipophilicity)
- adsorption coefficient.
For a long time, the lipophilicity character has been shown to play a basic role in determining distribution phenomena as well as influencing the mechanisms of ecotoxicity of organic chemicals. The classic
procedure for measuring lipophilicity of organic chemicals is based on the partition between octanol and water.
Effect concentration
The concentration, at which a chemical exerts an effect, depends on:
- its toxicokinetic behaviour
- uptake
- biotransformation
- distribution
- excretion/elimination
- its toxicodynamic behaviour
- interaction with receptor target
As in the environment, a number of processes in an organism such as uptake, distribution, biotransformation will determine the concentration of a compound at the target site. In addition, differences in the
potency of chemicals to interact with the target will influence the effect concentration.
Conclusion
The development of QSAR is based on the assumption that chemical substances, which reach and interact with a target site by the same mechanism, perform likewise due to their similar chemical properties.
The analysis for QSAR is through regression method affording transparent relations and simple mathematical equations and leading to a quantitative correlation. However, for a meaningful regression analysis,
precise and accurate input data are required which tend to limit the number of samples in the testing set. It also means that the data used should be carefully evaluated and not taken from any available
handbook unless the data quality is known to be validated (Hart 1991).
3 Pesticides
3.1 Modes of action
3.2 QSAR and pesticides
3.2.1 SMILES notation
3.3 Physico-chemical properties
3.3.1 Boiling point
3.3.2 Melting point
3.3.3 Solubility in water
3.3.4 Vapour pressure
3.3.5 Henry's Law constant
3.3.6 Octanol/water partition coefficient (Kow)
3.3.7 Sorption
3.4 Bioaccumulation
3.4.1 Bioaccumulation factor for aquatic organisms
3.4.2 Bioaccumulation factor for terrestrial organisms
3.5 Aquatic toxicity
3.5.1 QSAR models on aquatic ecotoxicity
3.5.2 Correlations between experimental and estimated ecotoxicity
3.5.3 QSARs developed for specific pesticides
3.5.4 QSARs derived from pesticides in the report
3.5.5 Discussion on estimated ecotoxicity
Pesticides are chemicals that are especially chosen for their ability to affect “unwanted organisms” whether it may be animals, plants, fungi or microorganisms without affecting non-target organisms. Thus, pesticides are biological active substances or preparations containing one or more substances with a broad range of biocidal activity and often with a
specific mode of action.
3.1 Modes of action
It has been observed that chemicals of analogous structures have similar modes of action on the target organism. Dividing such chemicals into chemical classes therefore means that predictions of the mode of
action are possible. Pesticides may act by contact or systemic mode of action. A contact action means that the pesticide by contact with the target organisms affects the surface or penetrates the surface to
reach the target site. A systemic mode of action means that a pesticide is taken up by an organism and then acts after the uptake (e.g. insecticides taken up by eating or sucking insects from the plants the
insecticide has entered).
Below some of the major chemical classes used in pesticides are presented.
Aryloxyalkanoic acids
Aryloxyalkanoic acids (phenoxy acids) are selective systemic hormone type herbicides that are absorbed by the leaves and roots and translocated throughout the plant but concentrates in the meristematic
regions where it inhibits growth.
Azoles
Azoles are fungicides acting as steroid demethylation inhibitor.
Carbamates
Carbamates may be herbicides or insecticides. As herbicides, carbamates are systemic herbicides, which are absorbed by the roots, leaves and stem, and translocated to active growth sites (meristems)
where it inhibits elongation of roots and aerial parts. Some carbamates are insecticides with contact and stomach action and act as cholinesterase inhibitor.
Dinitroanilines
Dinitroanilines are selective soil herbicides, which are absorbed by the roots. Dinitroanilines affect seed germination and prevent plant growth by inhibition of root and shoot development.
Organophosphorous
Organophosphorous substances are usually insecticides with contact and stomach action and act as cholinesterase inhibitor affecting the nervous system. Organophosphorous herbicides do exist as selective
herbicides, which are absorbed through the roots.
Pyrethroids
Pyrethroids are usually non-systemic insecticides with contact action. They cause paralysis initially (“knock-down effect”) and may lead to death later. Usually, they are non-phytotoxic.
Sulfonylureas
Sulfonylureas are selective systemic herbicides, which are absorbed by the leaves and roots and translocated throughout the plant. Sulfonylureas inhibit the acetolactate synthesis. Plant growth is inhibited
followed by the development of chlorotic patches, which spread acropetally and then basipetally.
Triazines
Triazines are selective systemic herbicides, which are absorbed by the leaves and roots and then translocated acropetally in the xylem to accumulate in apical meristems. Triazines inhibit photosynthesis and
interfere with other enzymatic processes.
Triazoles
Triazoles are non-selective systemic herbicides, which are absorbed by the leaves and roots and then translocated in both the phloem and the xylem. Triazoles inhibit the chlorophyll formation and re-growth
from buds.
Ureas
Ureas are selective herbicides, which are absorbed by the roots and leaves. They inhibit photosynthesis.
3.2 QSAR and pesticides
Most QSARs are based on simple chemical structures and the use on more complex organic molecules which is often the case with pesticides, should be performed with this in mind. However, some studies
have been using the QSAR models on pesticides. Generally, most aspects of pesticides are very well documented compared with other chemicals and this should improve the input data quality. However,
data on metabolites and degradation products are usually missing and validated QSAR models may present estimates on whether a metabolite or degradation product should be considered for more study or
not.
Evaluation of the validity of the latest accepted QSARs is performed by comparing experimental values and the QSAR model estimates using specified models.
The experimental values are based on information from the manufacturers (Tomlin 1994, 1997, Linders et al. (1994) or letters of approval of plant protection preparations from the Danish-EPA) and a
Danish-EPA database (Clausen 1998). It means that data on ecotoxicological effects are based on evaluated and accepted studies.
The QSAR estimations on physico-chemical proportions are performed by programmes developed by Syracuse Research Corporation: MPBPVP, WSKOW, KOWWIN, HENRY, and PCKOCWIN.
The programmes are stand-alone programmes but can be run together using the Estimation Programs Interface (EPIWIN) as an interface. The programmes require that the molecular structure be presented
as a SMILES notation, cf. 3.2.1.
Fortunately, the programme includes a database where, by using CAS number, the programme itself presents the SMILES notation. If no SMILES notation exists it can relatively easy be performed.
The QSAR estimations on toxicity to aquatic organisms are performed by QSARs recommended in the EU technical guidance document (TGD 1996).
3.2.1 SMILES notation
Simplified Molecular Input Line Entry System (SMILES) is a simple chemical notation system to identify the molecule from its structure by a linear string of symbols. The system has been designed so that a
computer can use it. The chemical structure is described from basal symbols and a few rules:
- The SMILES notation can begin at any atom in the molecular structure.
- Hydrogen atoms are not presented.
- Normal atoms are named by capital letters (C, O, N, S, P...) unless they are included in aromatic ring structures.
- Aromatic atoms are named by small letters (c, o, n, s).
- Single bonds are not shown, double bonds as “=“ and triple bonds as “#”.
- Branches are presented in brackets. A branch cannot begin a SMILES notation but must follow immediately after the atom to which it connects. If an atom has more than one branch, the branches are
coded as consecutive pairs of parentheses.
- Ring structures are numbered to identify which atoms that are connected to present the ring structure, e.g. benzene: c1ccccc1, 1,3,5-triazin: c1ncncn1 (Lagersted 1987). More rings are introduced by
increasing number.
For a more comprehensive introduction to SMILES see to Weininger (1988).
Writing SMILES notation is relatively easy since a SMILES notation depicts a molecular structure as a two-dimensional picture. A single structure can be depicted correctly from different SMILES. This
means that there may be more than one correct SMILES notation result if the rules are followed.
More complicated structures, such as some pesticides, may be difficult and time consuming to construct. A few examples have been presented for illustration (table 1).
Table 1
Examples of SMILES notation.
Eksempler på SMILES notation.
Substance |
Structure |
SMILES |
Isobutyric acid |
 |
CC(C)C(=O)O or
C(C)(C)C(=O)O or
OC(=O)C(C)C or
O=C(O)C(C)C |
Benzene |
 |
c1ccccc1 |
Triallate |
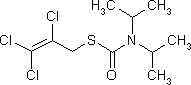 |
CC(C)N(C(C)C)C(=O)SCC(CL)=C(CL)CL |
Atrazine |
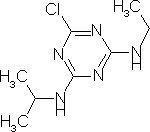 |
n(c(nc(n1)NC(C)C)NCC)c1CL |
Fenpropathrin |
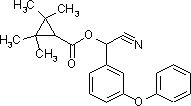 |
C1(C)(C)C(C)(C)C1C(=O)OC (C(#N))c2cccc(Oc3ccccc3)c2 |
Thifensulfuron-methyl |
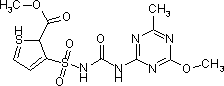 |
n1c(C)nc(OC)nc1NC(=O)NS(=O) (=O)c2ccsc2C(=O)OC |
3.3 Physico-chemical properties
3.3.1 Boiling point
The boiling point is defined as the temperature at which the vapour pressure of a liquid is equal to the pressure of the atmosphere on the liquid. For pure compounds, the normal boiling point is defined as the
boiling point at one standard atmosphere of pressure on the liquid. Besides being an indicator for the physical state (liquid vs. gas) of a chemical, the boiling point also provides an indication of the volatility.
The boiling point is estimated by using the Stein and Brown (1994) method of group contributions that calculates boiling point (BP) of a compound by adding group increment values according to the
relationship:
BP = 198.2 + Σnigi
Where gi is a group increment value and ni is the number of times the group occurs in the compound.
The resulting BP is then corrected by one of the following equations:
BP(corr) = BP - 94.84 + 0.5577 BP - 0.0007705 (BP)² |
[BP≤700°K] |
BP(corr) = BP + 282.7 - 0.5209 BP |
[BP>700°K] |
The Stein and Brown method was derived from a training set of 4426 organic compounds.
Other methods
Other methods are described in Lyman et al. (1982) but are either not validated or are using a reduced number of chemicals.
Pesticides
Boiling points for pesticides are usually not available. The available experimental values are either based on studies performed at non-comparable pressures or the substance decomposed before a boiling
point was reached. Apparently, most pesticides are estimated to have boiling points in the vicinity of 400°C according to the Stein and Brown method. The average estimated boiling point and standard
deviation was 406±102°C. Results of the estimations are presented in the appendix.
Conclusion
The validity of the estimations could not be evaluated due to the lack of experimental data on pesticide boiling points. Besides the Stein and Brown method, no other estimation method exists that has been
validated so extensively or accurately for diverse structures.
3.3.2 Melting point
The melting point is defined as the temperature at which crystals are in equilibrium with the liquid phase at atmospheric pressure. The melting point is an important parameter since it affects the solubility.
Solubility controls toxicity by affecting the bioavailability of the substance and the possibility of being transported to the active site within an organism. Primarily intermolecular forces and molecular symmetry
holding the molecules in a crystal lattice control the melting point of a compound. The melting point is determined by the strength of the crystal lattice since melting point is a measure of the energy required to
disrupt the crystal lattice. In the same way, the solubility can be regarded as a partitioning of the substance between its crystal lattice and the solvent. The melting point tends to increase with molecular size
simply because the molecular surface area available for contact with other molecules increases (Dearden 1991).
Meylan and Howard (1994) estimated the melting point by two different methods. The first is an adaptation of the Joback group contribution method for melting point and the second is a simple Gold and
Ogle method suggested by Lyman (1985).
The Joback adaptation is an extension of the original method to include the same groups as in the adapted Stein and Brown boiling point method. The Joback method overestimates MP for some structures.
The Gold and Ogle method simply relates melting point (MP) to boiling point (BP) as follows:
MP = 0.5839 BP (°K)
The computer programme MPBPVP by Meylan and Howard (1994) performs minor evaluations. If the values are close to the model averages, the two estimates are averaged, if not, the programme
performs and decides which estimate is more likely to be accurate and presents a “suggested” melting point. Although, the suggested MPBPVP estimates are usually adequate for screening purposes the
overall accuracy is not outstanding. The accuracy of the “suggested” value was tested on a 666 compound data set containing a diverse mix of simple, moderately complex compounds and many pesticides
and pharmaceutical compounds. MPBPVP estimates yielded a correlation coefficient (r²) of 0.73. However, even if the estimated melting points can only be used for screening purposes, it seems to be the
best method currently available (Meylan and Howard 1994).
Pesticides
Usually, the melting point of pesticides is known. Comparing the experimental melting points of the pesticides including salts and esters used in this study with the MPBPVP suggested melting points
performed the correlation presented in figure 1.
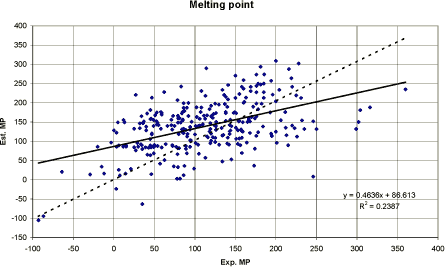
Figure 1
Experimental versus estimated melting points in °C. The dotted line represents the ideal correlation; i.e. the intercept is set to 0 and the slope to 1. The dark line represents the linear correlation.
Eksperimentelle overfor estimerede smeltepunkter i °C. Den stiplede linie repræsenterer ideal korrelation, dvs. skæringspunktet er sat til 0 og hældningen til 1. Den ubrudte linie repræsenterer
lineær korrelation.
In figure 1, the dotted trend line is placed with the intercept 0 and the slope 1, which represents the ideal situation of a perfect match. The position of the dotted line relative to the marking demonstrates that
most estimated values are apparently overestimated for the low experimental values and underestimated for the higher experimental values. The meanSD value for experimental and estimated melting points
was 112±69 and 140±69°C, respectively.
The correlation between the experimental values from 297 melting points and estimated values indicates that the estimations are inaccurate, as can be seen from the correlation coefficient (r² =0.24)
presented in the figure.
Conclusion
The correlation between experimental and estimated melting points was low.
The method generally overestimated the melting point values for substances with an experimental melting point up to 100°C. Furthermore it underestimated melting point values for substances with an
experimental melting point above 250°C. Thus, the currently best available method by Meylan and Howard (1994) cannot be used to estimate the melting point of pesticides with a sufficient accuracy in its
present form.
3.3.3 Solubility in water
The water solubility reflects the maximum amount of a chemical that will dissolve in pure water at a given temperature. The water solubility is one of the most important physico-chemical properties in
ecological hazard assessment and exposure assessment, including environmental fate. The spatial and temporal movement (mobility) of a substance within and between the environmental compartments of
soil, water and air depends largely on its solubility in water. The knowledge of the solubility in water is essential when estimating biological degradation, bioaccumulation, hydrolysis, adsorption and the
partition coefficient octanol/water. Highly water-soluble chemicals are potentially easier distributed by the hydrologic cycle, as they tend to have relatively low adsorption coefficients (i.e. low adsorption to
soil and sediment).
As the term “insoluble” is sometimes seen in handbooks it must be emphasised that no organic chemical is completely insoluble in water. All organic chemicals are soluble to some extent. The range observed
in pesticides is usually between µg/l to g/l. In a few instances, it may be even lower and some are infinitely soluble, i.e. total miscible with water.
Several approaches to estimate the water solubility have been developed (Lyman et al. 1982, Yalkowky and Banerjee 1992). Yalkowsky and Banerjee (1992) have reviewed most of the recent literature
where a variety of estimation methods are available. The methods involve the application of group activity coefficients, partition coefficients, chromatographic parameters, boiling point, molecular volume,
molecular surface area, molecular connectivity, parachor, solubility parameters, UNIFAC, linear solvation energy and multivariate statistical methods. After critical evaluation of the available methods in terms
of range of applicability, accuracy, ease of use and strength of underlying theory, Yalkowsky and Banerjee (1992) concluded that only two methods could be considered for universal application:
- group activity coefficient techniques which include group contribution fragment methods
- correlations based upon log Kow.
Group activity coefficient method
The group activity coefficient method is demonstrated with the group contribution approach of Wakita et al. (1986) that derived the following equations for:
Liquids: |
log S = -0.957 Σfi - 0.048 |
(n=307, r=0.982, sd=0.245) |
Solids: |
log S = -0.963 Σfi - 0.208 |
(n=112, r=0.986, sd=0.410) |
Where Σfi is the summation of all applicable fragment values. The fragment values are derived from experiments starting with small molecules and increasing the molecular structure with known atoms or
functional groups and calculate the contribution from each change in the molecular structure. The Wakita method was fairly accurate for its training set, which primarily consisted of hydrocarbons and simple
monofunctional compounds. When applied to a test set of pesticides and drugs, the accuracy was reduced: standard deviation (S.D.) = 1.05 (Yalkowsky and Banerjee 1992).
Correlations using log Kow
At present, the most practical method to estimate water solubility involves regression derived correlations using log Kow. Most of the highly water-soluble substances show low log Kow values. Several
correlations have been developed depending on the applied chemicals used in the calculations. Eighteen different regression equations that correlate water solubility to log Kow have been found in the
literature (Lyman et al. 1982, Isnard & Lambert 1989). Only the two equations, which include pesticides, are shown below:
Lyman et al. 1982
log S (mg/l) = |
-0.922 log Kow + 4.184 |
(n=90, r²=0.740) |
log S (µmol/l) = |
-1.49 log Kow + 7.46 |
(n=34, r²=0.970) |
Some equations include the melting point (MP) for solids. For liquids, the MP is set to 25°C which zeroes out the melting point parameter.
Isnard and Lambert (1989) performed a correlation on 300 substances (166 liquids and 134 solids) including some pesticides and obtained following correlations where log S is the logarithm to the water solubility and MP is the melting point:
Isnard and Lambert 1989
log S (mg/l) = 6.05 - 1.29 logKow |
(n=300, r=0.964, sd=0.631) |
log S (mg/l) = 5.90 - 1.18 logKow - 0.0048(MP-25) |
(n=300, sd=0.560) |
or |
|
log S (mol/m³) = 4.17 - 1.38 logKow |
(n=300, r=0.965, sd=0.665) |
log S (mol/m³) = 4.00 - 1.26 logKow - 0.0054(MP-25) |
(n=300, sd=0.582) |
The two latter equations were recommended for liquids and solids in OECD (1993b). The equations can be changed to give the result in mol/l:
log S (mol/l) = 1.17 - 1.38 log Kow
log S (mol/l) = 1.0 - 1.26 log Kow - 0.0054 (MP -25)
Meylan and Howard (1994) have developed a QSAR model on water solubility where the water solubility in mol/l is estimated based on log Kow with and without a melting point. The first equation was
developed based on a validation set of 85 substances with an experimental log Kow and water solubility values but with no available melting point. The second validation set included 817 compounds with
measured water solubility and melting points.
The Meylan and Howard equations are shown below:
Meylan and Howard 1994
log S (mol/l) = 0.796 - 0.854 logKow - 0.00728MW + cf |
(n=85, r²=0.865, sd=0.961) |
log S (mol/l) = 0.693 - 0.96 logKow - 0.0092(MP-25) - 0.00314MW + cf |
(n=817,r²=0.902, sd=0.615) |
Where MW is the molecular weight, MP is the melting point and “cf” the correction factor. Knowledge of the melting point reduces the standard deviation and improves the correlation coefficient and this
model should be used when a measured melting point is available. The melting point is only used for solids. The correction factor is applied to 15 structure types (e.g. alcohols, acids, selected phenols,
amines, amino acids, etc). The calculations of the Meylan and Howard QSAR model can be performed on computer (WS-KOW, Syracuse, Meylan and Howard 1995).
Pesticides
Performing a correlation between the experimental water solubility from 377 pesticides and the result of Meylan and Howard computerised version (WSKOW) is presented in figure 2 using log values. The
logarithmic values (log10) are used to reduce the range and to make the values comparable.
For a perfect match, the intercept should be 0 and the slope 1. If the estimated values were overestimated the values should be above the trend line with a slope of 1 and intercept 0. Such a trend line would
be close to the linear regression line presented in figure 2 that had a slope of 0.8.
Performing regression between the experimental water solubility values and the values estimated using the computerised WSKOW model with melting point resulted in a correlation coefficient r² of 0.65. It is
a poorer result than found by the training set used by Meylan and Howard (1994) but considering the wide diversity of the included pesticides the result cannot be considered unacceptable for a first
estimate.
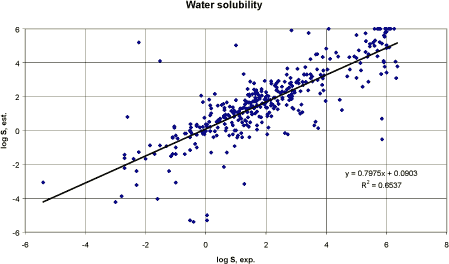
Figure 2
Correlation between the logarithm to the experimental water solubility and the logarithm to the estimated water solubility.
Korrelation mellem logaritmen til eksperimentel vandopløselighed og logaritmen til estimeret vandopløselighed.
Based on the measured water solubility and log Kow values from 322 pesticides, a linear regression analysis was performed. Figure 3 presents the result graphically.
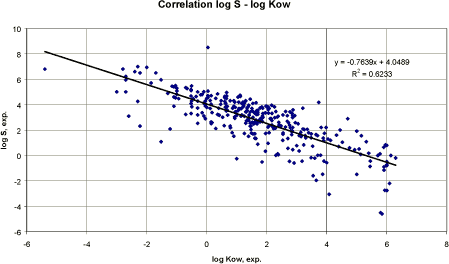
Figure 3
Correlation between the logarithm to the measured water solubility and log Kow.
Korrelation mellem logaritmen til målt vandopløselighed og log Kow.
The resulting correlations using mg/l (figure 3) or recalculating the solubility data to mmol/l were:
log S (mg/l) = -0.7639 log Kow + 4.0489 |
(n= 322, r²=0.6233) |
log S (mmol/l) = -0.844 log Kow + 1.5683 |
(n= 322, r²=0.6245) |
The result of the correlation seems relatively poor and no improvement was obtained by including the molecular weight. However, considering the variation in chemical classes etc. the result is not
unacceptable. It should also be considered that the experimental data are based on temperatures varying from 20 to 25°C whereas the estimated water solubility are estimated at 25°C. Figure 3 also
demonstrates the negative correlation between water solubility and octanol/water partition coefficient (lipophilicity).
Conclusion
The estimations of water solubility was reasonable acceptable using the selected computerised QSAR model although the correlation coefficient for pesticides was smaller than for the data used to develop
the model. A linear regression analysis based on log water solubility and log Kow of the pesticides used in this report resulted in a QSAR model with an almost identical correlation coefficient. Thus, both
methods may be used with the same degree of uncertainty.
3.3.4 Vapour pressure
The vapour pressure is defined as the pressure at which a solid is in equilibrium with its own vapour. The vapour pressure is a chemical specific property, which is important in evaluating the behaviour and
fate of a pesticide in the environment. Especially, when evaluating the distribution into the environmental compartments soil, air and water and its persistence in the compartments. Experimental or estimated
vapour pressures given in mmHg or atm. are recalculated to Pa in this report.
Numerous equations and correlations for estimating vapour pressure are presented in the literature. They normally require information on:
- the critical temperature
- the critical pressure
- the heat of vaporisation
- the vapour pressure at some reference temperatures
The vapour pressure is estimated by three methods:
- the Antoine method (Lyman et al 1990)
- the modified Grain method (Lyman 1985)
- the Mackay method (Lyman 1985).
All three methods use the normal boiling point to estimate the vapour pressure.
The Antoine method
The Antoine method was developed for liquids and gases. The method is described in detail in Lyman et al. (1990). The general equation is:
ln VP = [(ΔHvb (BP-C2)²) / (ΔZb R BP²)] * [1/(BP-C2) - 1/(T-C2)]
Where ΔHvb is the heat of vaporisation at the boiling point (cal/mol)
BP is the temperature of the normal boiling point in °K
C2 is a constant estimated to be = -18 + 0.19BP
T is the temperature in °K
ΔZb is assumed to have the value of 0.97.
R is the gas constant = 1.987 cal/mol × K
The modified Grain method
The modified Grain method is described in Lyman (1985). The method is a modification of the modified Watson method. It is applicable for solids, liquids and gasses. The method converts super-cooled
liquid vapour pressure to a solid phase vapour pressure. It is probably the best all round VP estimation currently available (Meylan and Howard 1994) and is used by US-EPA in the PC-CHEM
programme.
Mackay method
Mackay derived the following equation to estimate the vapour pressure:
ln VP = - (4.4 + ln BP)[1.803(BP/T-1) - 0.803 ln (BP/T)] - 6.8 (MP/T-1)
The equation includes the boiling point (BP), the melting point (MP) and the temperature (T) in °K. The melting point is ignored for liquids. Since the boiling point was usually unknown to pesticides, the
method was not applicable.
MPBPVP
The computer estimations made by MPBPVP (Meylan and Howard 1994) report all three methods and a “suggested” value. For solids, the suggested vapour pressure is the modified Grain estimate. For
liquids and gasses, the average of the Antoine and the modified Grain method is suggested. The Mackay method is not used, as it is limited to its derivation classes: hydrocarbons and halogenated
compounds (both aliphatic and aromatic). Using a data set of 805 compounds, a correlation coefficient (r²) of 0.941 and a standard deviation, S.D., of 0.717 were observed.
Pesticides
For the pesticides, a linear regression analysis has been performed using log transformation due to the large variation in the experimental vapour pressure values. The result is presented graphically in figure 4.
The experimental vapour pressure values and values estimated by the model MPBPVP and presented as suggested values (Meylan and Howard 1994) were not well correlated for pesticides. The
correlation coefficient r² was 0.46 for n = 308. The deviations seem to increase at decreasing vapour pressures. The reason for the low correlation coefficient may be the relatively few outliers. However,
removing the outliers did not significantly improve the result.
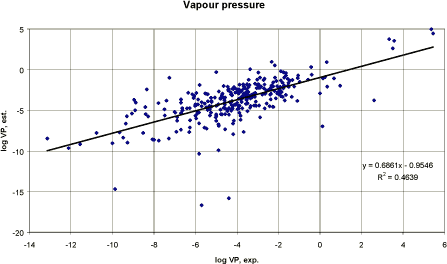
Figure 4
Correlation between the experimentally reported vapour pressure and the model-estimated vapour pressure (both logarithmic).
Korrelation mellem eksperimentelt rapporteret damptryk og model-estimeret damptryk (begge logaritmiske).
Conclusion
Generally, the presented QSAR model was not able to perform a sufficient close correlation of experimental and estimated vapour pressures from the included pesticide data set. However, the main part of
the substances is relatively well correlated and the estimation method may be considered sufficient for a first estimate.
3.3.5 Henry's Law constant
Henry's constant is the ratio of chemical concentration in air to the concentration in water, when those two phases are in contact and are at equilibrium. The partitioning between water and air is a physical
property that is described by the Henry's Law constant, H. The magnitude of H provides an indication of which of the two phases a chemical will tend to partition into at equilibrium. Henry's Law constant
can be estimated from:
H = Vapour pressure x Molecular weight / Water solubility [Pa m³/mol]
H can also be expressed as the ratio of the concentration in air and water, called the “dimensionless” H, H', where:
H' = concentration in air / concentration in water, i.e.
H' = kair-water = H / (gas constant (8.314) x temperature (°K))
Where kair-water is the air-water partition coefficient.
QSAR models
QSAR estimations of H are based on a combination of connectivity indices and calculated polarisability (Nirmalakhandan and Speece 1988). A narrow range of chemical types was used to develop the
method, which is not widely applicable.
QSARs based on group and bond contributions are developed from experimentally measured log Kair-water values, when available. The methods of Hine and Mokerjee (1975) have been further
developed and are now available in PC programme (HENRY in EPIWIN, Meylan and Howard 1992).
Compounds with large structures, which include many different types of bonds and groups, may have significant inaccuracies in their estimations.
Pesticides
For the pesticides in this project, no measured Henry's Law constants were available. It was decided to compare the computerised estimation with the calculated H values using:
H = VP (Pa) * MW (g/mol) / S (mg/l) [Pa m³/mol]
This is the preferred estimation method when reliable measured data on vapour pressure and water solubility are available (Meylan and Howard 1991). In the PC-model, the group estimation methods were
usually presenting the message “incomplete” in the estimation programme and therefore the bond contribution method was applied in the estimations (Meylan and Howard 1991).
The regression analysis between the calculated H and the H estimated by bond contribution method is shown graphically in Figure 6 and resulted in a correlation coefficient r² of 0.43 (n= 308). The results of
the bond contribution method are relatively underestimated compared with the calculated values.
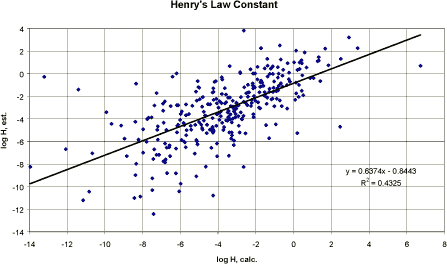
Figure 5
Correlations between Henry's Law constant calculated and estimated by structure analysis in HENRY PC-model (Meylan and Howard 1992).
Korrelation mellem Henrys Lov konstant beregnet og estimeret ud fra strukturanalysen i HENRY Pc-modellen (Meylan and Howard 1992).
No conclusion can be drawn from the used pesticide data set since values estimated by calculation and by computer model are not necessarily correct for the pesticide in question. It can be concluded that
the two estimation methods are presenting comparable results with estimated H being slightly above calculated H below log H -2 and slightly above the calculated H above log H -2.
3.3.6 Octanol/water partition coefficient (Kow)
Hydrophobicity is one of the key parameters in QSARs for environmental endpoints. The property is usually modelled by the n-octanol/water partition coefficient (Kow) which is an established laboratory
method to measure the hydrophobicity of a chemical. As such, Kow or log Kow is a key parameter in the assessment of environmental fate and the bioaccumulation potential. Kow has been found to be a
good predictor for relatively non-specific processes. For instance, many distribution processes are found to be related to Kow, e.g. sorption to soil and sediment, partitioning into air and bioconcentration,
and non-specific toxicity. This especially relates to non-polar organic chemicals. When more polar chemicals and more specific processes such as degradation, biodegradation and specific toxic interactions
are the subject, other kinds of interactions (stereo-electronic) become more relevant (EEC report 1995).
The octanol/water partition coefficient is the ratio of a chemical's concentration in the octanol phase to its concentration in the aqueous phase of a two-phase system at equilibrium. Thus, the two-phase
system consists of octanol as the non-polar solvent and water as the polar solvent. Since measured values range from below 10-4 to more than 108, the logarithm (log Kow) is normally used to characterise
its value.
The literature contains several methods for estimating log Kow. The most common method for the estimation of Kow is based on fragment constants. The fragmental approach is based on simple addition of
the lipophilicity of the individual molecular fragments of a given molecule, i.e. atoms or larger functional groups. The most widely used fragment constant method was proposed by Hansch and Leo (1979)
and initially computerised for use by Chou and Jurs in the CLOGP programme (Daylight Chemical Information Systems, New Orleans). The fragment constant method consists of determining the log Kow
values of a set of small molecules very accurately and then calculating “fundamental” chemical fragments from these values. The method uses single atom “fundamental” fragments consisting of isolated
carbons or a hydrogen or hetero-atom plus multiple atom fundamental fragments, e.g.-CN, and a large number of correction factors. Other methods have been developed but have, at present, not proven to
be acceptable as a general estimation method (Meylan and Howard (1995). Meylan and Howard (1995) have evaluated 10 different methods and concluded that CLOGP and the AFC methods (cf. below)
are the best comprehensive predictors currently available.
A major problem with most fragment constant approaches is their inability to estimate log Kow for a structure containing a fragment that has not been correlated.
Meylan and Howard (1995) have developed a new fragment constant approach, the atom/fragment contribution (AFC) method which was developed by multiple linear regressions of reliable experimental
log Kow values. The regressions were performed in two stages: The first regression correlated atom/fragment values with log Kow and the second correlated correction factors. The log Kow is then
estimated by summing up the values from a structure.
In general, each non-hydrogen atom, e.g. carbon, nitrogen, oxygen, sulphur, in a structure is a core for a fragment and the exact fragment is determined by what is connected to the atom. The result of the
atom and/or fragment regression was:
log Kow = (Σfini) + 0.229 |
(n=1120, r²=0.98, SD=0.22) |
Where (Σfini) is the summation of fi (the coefficient for each atom or fragment) times ni (the number of times the atom/fragment occurs in the structure).
The general equation for estimating log Kow of any organic compound is
log Kow = (Σfini) + (Σcjnj) + 0.229 |
(n=2351, r²=0.982, SD=0.216) |
Where (Σcjnj) is the summation of cj (the coefficient for each correction factor) times nj (the number of times the correction factor occurs or is applied in the structure).
Examples
Three calculation examples using pesticides are presented
Simazine
Simazine is a herbicide belonging to the triazines and has the molecular structure presented below:
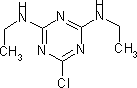
Table 2
Simazine, structure fragment analysis used to calculate log Kow.
Simazin, strukturfragmentanalyse anvendt til beregning af log Kow.
Fragment: |
Coefficient |
Frequency |
Value |
-CH3 |
0.5473 |
2 |
1.0946 |
-CH2 |
0.4911 |
2 |
0.9822 |
Aromatic carbon |
0.2940 |
3 |
0.8820 |
Aromatic nitrogen |
-0.7324 |
3 |
-2.1972 |
-N (one aromatic attachment) |
-0.9170 |
2 |
-1.8340 |
-Cl (aromatic attachment |
0.6445 |
1 |
0.6445 |
Correction factors: |
|
|
|
Symmetric triazine ring correction |
0.8856 |
1 |
0.8856 |
Amino type triazine |
0.8566 |
2 |
1.7132 |
Equation constant |
|
|
0.2290 |
|
log Kow = |
2.3999 |
Simazine has an experimental log Kow of 2.18. Based on structure fragment analysis the log Kow was estimated to be 2.40.
Thiodicarb
Thiodicarb is an insecticide belonging to the oxime carbamates and has the molecular structure:
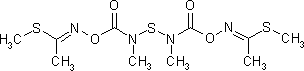
Table 3
Thiodicarb, structure fragment analysis used to calculate log Kow.
Thiodicarb, strukturfragmentanalyse anvendt til beregning af log Kow.
Fragment: |
Coefficient |
Frequency |
Value |
-CH3 |
0.5473 |
6 |
3.2838 |
C (no hydrogens) |
0.9723 |
2 |
1.9446 |
-N< (aliphatic attachment) |
-1.8323 |
2 |
-3.6646 |
-ON (nitrogen attachment) |
0.2352 |
2 |
0.4704 |
OC(=O)N (carbamate type carbonyl) |
0.1283 |
2 |
0.2566 |
-N=C (aliphatic attachment) |
-0.0010 |
2 |
-0.0020 |
-SC= (aliphatic C=) |
-0.1000 |
2 |
-0.2000 |
-S- (two nitrogen attachments) |
1.2000 |
1 |
1.2000 |
Correction factors: |
|
|
|
Di-N-aliphatic substituted carbamate |
0.1984 |
2 |
0.3968 |
>C=NOC(=O)- |
-1.0000 |
2 |
-2.0000 |
Equation constant |
0.2290 |
|
log Kow = |
1.9146 |
Thiodicarb has an experimental log Kow of 1.70. Based on structure fragment analysis the log Kow was estimated to be 1.91.
Thifensulfuron-methyl
Thifensulfuron-methyl is a herbicide belonging to the sulfonylureas. It has the molecular structure shown below.
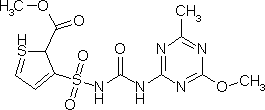
Table 4
Thifensulfuron-methyl, structure fragment analysis used to calculate log Kow.
Thifensulfuron-methyl, strukturfragmentanalyse anvendt til beregning af log Kow.
Fragment: |
Coefficient |
Frequency |
Value |
-CH3 |
0.5473 |
3 |
1.6419 |
-NH (aliphatic attachment) |
-1.4962 |
1 |
-1.4962 |
Aromatic carbon |
0.2940 |
7 |
2.0580 |
Aromatic nitrogen |
-0.7324 |
3 |
-2.1972 |
-N (one aromatic attachment) |
-0.9170 |
1 |
-0.9170 |
-O- (one aromatic attachment) |
-0.4664 |
1 |
-0.4664 |
Aromatic sulphur |
0.4082 |
1 |
0.4082 |
-C(=O)O (ester, aromatic attachment) |
-0.7121 |
1 |
-0.7121 |
-SO2N (aromatic attachment) |
-0.2079 |
1 |
-0.2079 |
-NC(=O)N- (urea type) |
1.0453 |
1 |
1.0453 |
Correction factors: |
|
|
|
Symmetric triazine ring correction |
0.8856 |
1 |
0.8856 |
Amino type triazine |
0.8566 |
1 |
0.8566 |
Alkyloxy ortho to two nitrogens |
0.8955 |
1 |
0.8955 |
-NC(=O)NS on triazine |
-0.7500 |
1 |
-0.7500 |
Equation constant |
0.2290 |
|
log Kow = |
1.2733 |
Thifensulfuron-methyl has an experimental Kow of 1.6 at pH 5 and a Kow of 0.02 at pH 7.
The deviation demonstrates that the method results are “ion-corrected” for compounds that have log Kow values that vary with pH. The log Kow correction for ionisation is calculated as:
log Kow corrected = log KowpH + log(1+10pH-pKa).
Thifensulfuron-methyl has a pKa value of 4.0. This would result in a corrected Kow at pH 7 = log (0.02) + log (1+107 - 4) = log 1.3.
Pesticides
Performing a correlation between 327 pesticides, an overestimation seems to be the case for pesticides of low experimental Kow values. In figure 6, the trend line with the intercept 0 and the slope 1 would
be almost identical to the linear regression line that had the slope 0.87. Thus, the correlation is close to represent the ideal correlation.
A linear regression analysis between the experimental log Kow and the estimated log Kow gave a correlation coefficient r² of 0.72 (n = 327) (figure 6) which is sufficient close for predictive purposes.
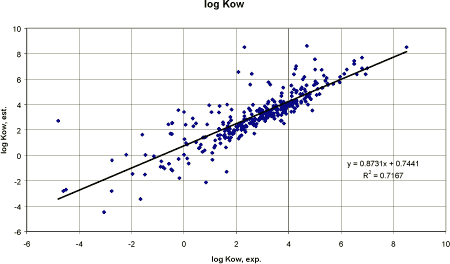
Figure 6
Correlation analysis between the experimental log Kow and the estimated log Kow.
Korrelationsanalyse mellem den eksperimentelle log Kow og den estimererede log Kow.
Some derivation may arise from imprecise or incorrect log Kow's or from “ionised” substances (cf. the thifensulfuron-methyl example above). However, the results are accurate enough to be used for the
estimation on related chemical compounds, e.g. pesticide degradation and/or transformation products. Concerning the pesticide active substance itself, there should always be an evaluated measured value
present and the value should consider different pH if the substance is depending on pH (Ribo 1988). This is especially important since the log Kow is an essential part of most predicting methods on
adsorption, toxicity etc.
Very often several log Kow values may be found even as experimental data obtained from laboratory methods performed according to accepted guidelines (reverse phase HPLC, “shake-flask”, “slow
stirring method” etc). In such cases, QSAR models may be used in evaluating which value to decide on.
The method is, however, not recommendable for manual calculations since 130 fragments and 235 correction factors are included. The PC versions of the methods AFC or CLOGP are recommended
instead.
Log Kow estimated from water solubility
Based on the selected pesticides in this report, a QSAR for manual calculation of an estimated log Kow based on water solubility can be suggested.
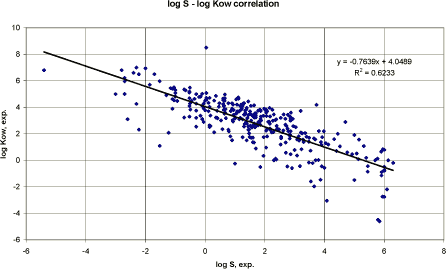
Figure 7
Correlation between experimental water solubility (mg/l) and log Kow.
Korrelation mellem eksperimentel vandopløselighed (mg/l) og log Kow.
Performing linear regression between the measured water solubility either as mg/l or mmol/l and log Kow resulted in the equations:
log Kow = - 0.7639 log S (mg/l) + 4.0489 |
(n=322, r² = 0.6233) |
log Kow = - 0.7322 log S (mmol/l) + 2.2024 |
(n=322, r² = 0.6229) |
The correlation coefficient was 0.62 which is reasonable acceptable for predictive purposes.
Conclusion
The octanol/water partition coefficient log Kow could be reasonably well estimated from the QSAR models based on structure fragment analysis. The method is complicated and recommended to be
performed by available computer models. However, an alternative QSAR model may be used based on water solubility. For the pesticides used in this report, it resulted in a lower correlation coefficient but
if the computerised model is not available the method should be sufficient in a screening procedure.
3.3.7 Sorption
The sorption (adsorption/desorption) to soil and sediments is a determining factor for the mobility of chemicals. This property also contributes to the distribution among soil, sediment and water phases,
volatilisation from soil surfaces, and bioavailability. The extent of soil and sediment sorption is governed by a variety of physico-chemical properties of both the soil/sediment and the chemical, e.g. organic
carbon content, clay content, humidity, pH value, cation exchange capacity, temperature, etc.
The sorption of non-polar substances may be regarded as a distribution process between the polar phase of the soil water and the organic phase of the soil component. The equilibrium constant of this
partitioning between solid and solution phase constitutes the adsorption coefficient for soil and sediments. The sorption coefficient is defined, at steady state, as:
Kd = Concentration of chemical sorbed to soil / Mean concentration in aqueous solution.
As the organic fraction is the principal interaction site for hydrophobic compounds, a partition coefficient normalised for the content of organic carbon (OC) is used to reduce the variance of sorption
coefficients:
Koc = (Kd/OC%) 100
The remaining variation may be due to other characteristics of soils (clay content, clay composition, surface area, cation exchange capacity, pH, etc.), the nature of the organic matter present and/or variation
in the test methods. Numerous studies of the correlation of adsorption coefficient with these variables found that the organic carbon content usually gave the most significant correlations (Stevenson 1976).
Soil adsorption coefficients may be reported on a soil-organic matter basis (Kom). Since the organic carbon content of a soil can be measured more directly, reporting values as Koc are preferred. The ratio
of organic matter to organic carbon varies from soil to soil but a value of 1.724 is often assumed when conversion is necessary, i.e. Koc =1.724 Kom (Lyman et al. 1990) based on an organic carbon
content of 58% in organic matter.
Since Koc is accepted as essential in the evaluation of pesticides, many different procedures for estimating Koc have been developed. One general method correlates Koc with Rf-values obtained from soil
thin-layer chromatography tests. Various authors have shown a reasonable good correlation between these properties but gave no regression equation (Briggs 1973, Hance 1967, Lambert 1968) or required
an additional parameter (pore fraction of the soil (Hamaker 1975)). Briggs (1969) described a correlation between Koc and the Hammet and Taft energy constants for phenylureas but without presenting a
regression equation.
Other factors affect the measured value of Koc under actual environmental conditions besides the differences in laboratory procedures (Lyman 1990):
- temperature
- pH of soil and water
- particle size distribution and surface area of solids
- concentration of dissolved organic matter in water
- non-equilibrium adsorption mechanisms or failure to reach equilibrium conditions
- solids to solution ratio
- loss of chemical due to volatilisation, degradation, adsorption to test flask walls etc.
- non-linear isotherm
- time factor
Temperature
Temperature may affect the measured values since adsorption is an exothermic process. Values of Koc usually decrease with increasing temperature.
pH of soil and water
Chemicals that tend to ionise are much affected by pH. Weak acids and weak bases show the greatest sensitivity to pH changes in the range normally met in soil and surface waters (pH 5 to pH 9).
Particle size distribution and surface area of solids
The fine silt and clay fraction of soil and sediments may have a great tendency to absorb chemicals. The different clay fractions have different adsorptive capacities.
Non-equilibrium adsorption
Non-equilibrium adsorption may occur when a chemical moves through an environmental compartment so rapidly that equilibrium conditions cannot be achieved.
Solids to solution ratio
Changes in the water content of a soil or sediment will change the fraction of chemical that is adsorbed. As the water content is lowered, the fraction adsorbed will increase as the concentration in solution
does.
Loss of chemical
The chemical may be lost during the test due to volatilisation, degradation, adsorption to test flask walls etc. if this is not considered.
Non-linear isotherm
If the adsorption isotherm is non-linear, the reported value of Koc will depend on the range of chemical concentrations used in the tests.
Time factor
The time for the chemicals to adsorb/desorb varies depending on conditions, substance properties etc.
Models
Several compilations of QSAR models for soil sorption are published in the literature. All of the available methods for estimating Koc involve empirical relationships with some other property of the chemical:
- water solubility
- octanol/water partition coefficient (Kow)
- bioconcentration factor etc.
Most models are based on Kow because hydrophobic interactions are the dominant type of interactions between non-polar substances and soil organic carbon. However, chemicals with more polar groups
may interact by other types of interaction. It is therefore obvious that not one single model accurately predicts soil sorption coefficients and that different models should be used depending on which class of
chemicals that the specific compound belongs to.
Some of the models/equations have been developed from training sets of insecticides, herbicides and fungicides or compounds of related structure.
The relationships are regression equations usually expressed in the log-log form:
log Koc = a log (S, Kow, or BCF) + b
Where “a” and “b” are constants.
Examples of QSARs developed for estimating log Koc from experimental data on water solubility, log Kow and bioaccumulation factor BCF are given in Lyman et al. (1982) and presented in table 5 for
different chemical classes.
The second equation in table 5 was derived from pesticides (Kenaga 1980). Individual results estimated for the pesticides selected for this report can be found in the appendix under the heading ”Koc,
Lyman, est”.
Table 5
Estimation of Koc (Lyman et al. 1982).
Estimering af Koc (Lyman et al. 1982).
Chemical classes |
Equation |
n |
r² |
Variable, mostly pesticides |
log Koc = -0.55 log S + 3.64 |
106 |
0.71 |
Variable, mostly pesticides |
log Koc = 0.544 log Kow + 1.377 |
45 |
0.74 |
Aromatics, polynuclear aromatics, triazines and dinitroanilin herbicides |
log Koc = 0.937 log Kow - 0.0006 |
19 |
0.95 |
s-triazines and dinitroaniline herbicides |
log Koc = 0.94 log Kow + 0.02 |
9 |
NA |
Variety of insecticides, herbicides and fungicides |
log Koc = 1.029 log Kow - 0.18 |
13 |
0.91 |
Substituted phenylureas and alkyl-N-phenylcarbamates |
log Koc = 0.524 log Kow + 0.855 |
30 |
0.84 |
Variable, mostly pesticides |
log Koc = 0.681 log BCF + 1.886 |
22 |
0.83 |
S: water solubility in mg/l. NA: not available. n: number. r²: correlation coefficient.
The EU Technical Guidance Document (TGD 1996) for risk assessment presents 19 equations to estimate log Koc in soil and sediment for different chemical classes. The 19 QSAR models were developed
by Sabljic et al. (1995). For values of log Kow, evaluated and recommended log Kow values (known as LOGPSTAR data) or reliable ClogP data were used. For log Koc, data from two literature
compilations of soil sorption coefficients were used and median values when several measured Koc values were available (Sabljic et al. 1995). The soil sorption data used in Sabljic et al. (1995) were
determined for non-ionic species of respective chemicals and thus, the QSAR models presented in the table 6 will be applicable only for non-ionised chemicals:
Table 6
List of derived QSAR models for soil sorption with their chemical domains (Sabljic et al. 1995).
Liste over udledte QSAR modeller til estimering af adsorption med deres kemiske områder (Sabljic et al. 1995).
Chemical class |
Regression equation |
n |
r² |
SE |
Predominantly hydrophobics |
log Koc = 0.81 log Kow + 0.10 |
81 |
0.89 |
0.45 |
Nonhydrophobics |
log Koc = 0.52 log Kow + 1.02 |
390 |
0.63 |
0.56 |
Phenols, anilines, benzonitriles, and nitrobenzenes |
log Koc = 0.63 log Kow + 0.90 |
54 |
0.75 |
0.40 |
Acetanilides, carbamates, esters, phenylureas, phosphates, triazines, triazoles, and uracils |
log Koc = 0.47 log Kow + 1.09 |
216 |
0.68 |
0.43 |
Alcohols and organic acids |
log Koc = 0.47 log Kow + 0.50 |
36 |
0.72 |
0.39 |
Acetanilides |
log Koc = 0.40 log Kow + 1.12 |
21 |
0.51 |
0.34 |
Alcohols |
log Koc = 0.39 log Kow + 0.50 |
13 |
0.77 |
0.40 |
Amides |
log Koc = 0.33 log Kow + 1.25 |
28 |
0.46 |
0.49 |
Anilines |
log Koc = 0.62 log Kow + 0.85 |
20 |
0.82 |
0.34 |
Carbamates |
log Koc = 0.365 log Kow + 1.14 |
43 |
0.58 |
0.41 |
Dinitroanilines |
log Koc = 0.38 log Kow + 1.92 |
20 |
0.83 |
0.24 |
Esters |
log Koc = 0.49 log Kow + 1.05 |
25 |
0.76 |
0.46 |
Nitrobenzenes |
log Koc = 0.77 log Kow + 0.55 |
10 |
0.70 |
0.58 |
Organic acids |
log Koc = 0.60 log Kow + 0.32 |
23 |
0.75 |
0.34 |
Phenols and benzonitriles |
log Koc = 0.57 log Kow + 1.08 |
24 |
0.75 |
0.37 |
Phenylureas |
log Koc = 0.49 log Kow + 1.05 |
52 |
0.62 |
0.34 |
Phosphates |
log Koc = 0.49 log Kow + 1.17 |
41 |
0.73 |
0.45 |
Triazines |
log Koc = 0.30 log Kow + 1.50 |
16 |
0.32 |
0.38 |
Triazoles |
log Koc = 0.47 log Kow + 1.405 |
15 |
0.66 |
0.48 |
n: Number of substances. r²: Correlation coefficient. SE: Standard error.
In table 6, predominantly hydrophobics were in this context defined as compounds that contain only carbon, hydrogen and halogen atoms (i.e. C, H, F, Cl, Br, I). Nonhydrophobics were are all the
chemicals not defined as predominantly hydrophobic. It means that the definition was based on molecular structure and does not imply anything about lipophilicity.
For agricultural chemicals (acetanilides, carbamates, esters, phenylurea, phosphates, triazines and uracils), the following equation was recommended (cf. table 6 above):
log Koc = 0.47 log Kow + 1.09 |
(n=216, r²=0.68, SE=0.43) |
Of other methods, the first order molecular connectivity index (1) has successfully been used to predict log Koc for hydrophobic organic compounds (Sabljic 1987, Bahnick and Douchette 1988). The
calculation has been computerised and Meylan et al. (1992) describe the estimation methodology. Briefly, the equation used is:
log Koc = 0.53 ¹χ + 0.62 + ΣPf
Where ¹χ is the first order molecular connectivity index and ΣPf is the summation product of all applicable correction factors (Meylan and Howard 1994). The calculations are performed by PCKOC part of
the EPIWIN (Meylan and Howard 1994).
Pesticides
Because pesticides are the subjects of this report, correlations have been performed using both the model calculation programmes PCKOC (Epiwin, Syracuse) and the TGD recommended QSAR model by
Sabljic et al. (1995).
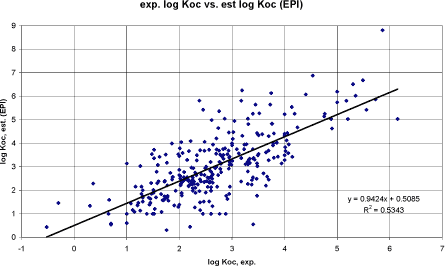
Figure 8
Correlation between experimental log Koc and log Koc estimated by PCKOC programme.
Korrelation mellem eksperimentel log Koc og log Koc vurderet efter PCKOC-program.
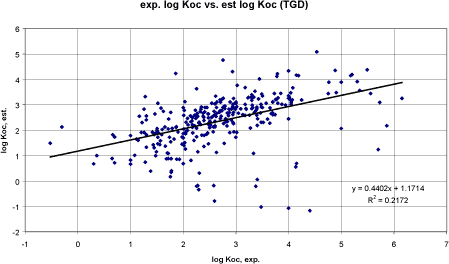
Figure 9
Correlation between experimental log Koc and log Koc estimated by TGD's QSAR model for pesticides (Table 6, 4th equation).
Korrelation mellem eksperimentel log Koc og log Koc vurderet efter TGDs QSAR-model for pesticider (Tabel 6, 4. ligning).
Performing a linear regression between the experimental Koc and the Koc estimated by the computer model EPIWIN (Meylan and Howard, Syracuse) resulted in a correlation coefficient r² of 0.53 (n=
306) (cf. figure 8). Performing the same regression using the QSAR model recommended in TGD for agricultural chemicals (cf. above) resulted in a correlation coefficient r² of 0.22 (n= 306) (cf. figure 9). It
would normally result in the EPIWIN model to be recommended. However, the poor result of the TGD model seem to be related to outliers whereas the main points are closer to the regression line than in
the EPIWIN estimates and the TGD QSAR models for Koc estimation developed by Sabljic et al. may be used with care.
Generally, all the correlation coefficients of the QSARs examined in this study were poor. The slope of the correlation equation for the EPI and the TGD recommended QSAR for hydrophobic substances
were the closer to 1 (n = 305. y = log Koc. x = log Kow. Individual values can be found in the appendix):
QSAR equation used: |
Correlation equation: |
r²: |
EPI structure analysis (figure 8) |
y=0.9424 x + 0.5085 |
0.5343 |
Lyman et al. 1982 (2nd eq. table 5) |
y=0.5006 x + 1.4658 |
0.2236 |
TGD, hydrophobics (1st eq., table 6) |
y=0.7454 x + 0.2322 |
0.2236 |
TGD, non-hydrophobics (2nd eq., table 6) |
y=0.4785 x + 1.1049 |
0.2236 |
TGD, pesticides (4th eq., table 6) |
y=0.4402 x + 1.3714 |
0.2172 |
Performing a linear regression analysis between the experimental log Kow and experimental log Koc demonstrates problems in estimating Koc from low log Kow values, cf. figure 10.
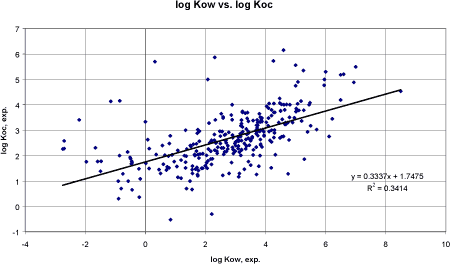
Figure 10
Correlation between experimental log Kow and experimental log Koc.
Korrelation mellem eksperimentel log Kow og eksperimentel log Koc.
Based on 300 pesticides, the correlation coefficient r² was 0.34 and unacceptably low but an improvement compared to the Lyman and TGD correlations. Apparently, the data are not linear. A polynomial
regression of the 2nd order was tried (figure 11) and resulted in the equation:
log Koc = 0.055 logKow² + 0.0764 logKow + 1.8476
The correlation coefficient r² was 0.41 using data from 300 pesticides. The graph demonstrated that a linear correlation might be most valid between log -1 to 5. The individual results are presented in the
appendix under the heading log Koc, OCH estimate.
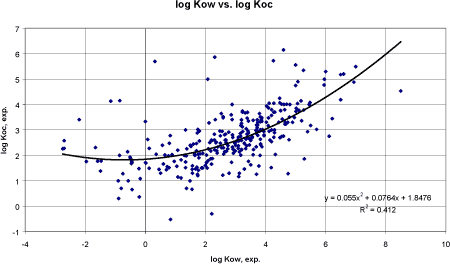
Figure 11
Correlation between experimental log Kow and experimental log Koc. Trend line adapted by polynomial regression (n=300).
Korrelation mellem eksperimentel log Kow og eksperimentel log Koc. Kurven er fastlagt ud fra en polynomial regression (n=300).
A new linear regression analysis was tried after leaving out the pesticides with the lowest log Kow values. The result is presented in figure 12.
Reducing the number of outliers by narrowing the log Kow range from 0 to 8.5 increased the correlation coefficient to 0.39. The resulting regression equation or derived QSAR model was:
log Koc = 0.4389 log Kow + 1.3752 |
(n= 273, r²=0.3851) |
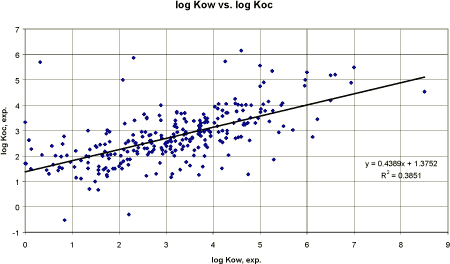
Figure 12
Correlation between experimental log Kow and experimental log Koc. Outliers below log Kow 0 was removed.
Korrelation mellem eksperiment log Kow og eksperimentel log Koc. Data med log Kow mindre end 0 er udeladt.
Using the water solubility to estimate the adsorption coefficient did improve the result (figure 13).
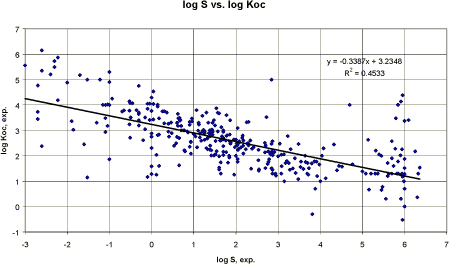
Figure 13
Correlation between experimental water solubility ( log S) and experimental log Koc.
Korrelation mellem eksperimentel vandopløselighed (log S) og eksperimentel log Koc.
The resulting correlation was:
log Koc = -0.3387 log S + 3.2348 |
(n= 338, r²=0.45) |
The result is close to the QSAR observed for pesticides by Kanazawa (1989), although for a fewer number:
log Koc = -0-356 log S + 3.01 |
(n=15, r=-0.887, p<0-001) |
Conclusion
The soil adsorption coefficient factor is very important in the evaluation of the mobility of pesticides and since the known methods for estimation are approximate at best, measured values should be
recommended.
If however, measured data for some reason are not present; the QSAR model to estimate Koc developed by Sabljic et al. (1995) and recommended in TGD (1996) or the calculation programme PCKOC
may be used. However, based on 338 pesticides in this project, QSAR models, based on log Kow or the water solubility, were derived which had correlation coefficients which were better than the model
by Sabljic et al (1995). These QSARs derived for pesticides could be considered when evaluating experimental results are either not present or conflicting.
3.4 Bioaccumulation
3.4.1 Bioaccumulation factor for aquatic organisms
The uptake of chemical substances into living organisms occurs mostly by direct adsorption but also along trophic web. The internal concentration, e.g. in fish, may increase by accumulation to a level causing
toxic effects, even if the internal concentration remains below the critical limit (OECD 1993b). The accumulated substance may then be passed on to other organisms higher up in the food web which were
not directly exposed themselves.
The bioaccumulation or bioconcentration factor (BCF) in aquatic organisms is defined as the ratio between the concentration of the chemical in biota and the concentration in water at equilibrium.
Procedures for estimating the bioconcentration potential have been reviewed by e.g. Lyman et al. (1982), Connell (1988), Nendza (1991b), and OECD (1993b). Comparison of non-ionic organic
chemicals exhibiting substantial bioconcentration revealed several common characteristics. The bioconcentration potential of a chemical was directly related to its lipophilicity and inversely related to its water
solubility, molecular charge and degree of ionisation (Lyman et al. 1982, Connell 1988). In fish, the lipid tissue is the principal site for bioaccumulation and since n-octanol is often a satisfactory surrogate for
lipids, linear correlations are usually observed between log BCF and log Kow. Most QSAR models on bioconcentration are based on log Kow. The simplest form of the relationships is based on the
partition process of the lipid phase of fish and water:
BCF = a × Kow (where a is the lipid fraction actually ranging 0.02 - 0.2)
It is generally agreed that a linear relationship exists for chemicals, which are not biotransformed with a log Kow < 6. Veith et al. (1979) developed a linear model based on fathead minnows (Pimephales
promelas) valid for log Kow < 6:
log BCF = 0.85 log Kow - 0.70 |
(n = 55, r² = 0.90) |
In the log Kow range above 6, the measured log BCF data tend to decrease with increasing log Kow.
Performing a regression analysis, using the Veith et al. (1979) developed QSAR on the pesticides used in this report, would result in the following correlation (figure 14):
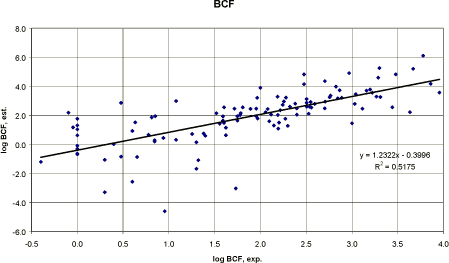
Figure 14
Correlation between the experimental log BCF and the estimated log BCF (Veith et al. 1979).
Korrelation mellem den eksperimentelle log BCF og den estimerede log BCF (Veith et al. 1979).
The correlation between the experimental values and the estimated values seems to be acceptable with a correlation coefficient, r², of 0.52 (figure 14). The result is less accurate than Veith et al. (1979) but
it is to be expected considering the diverse group of chemical compounds, different fish species and different laboratories although the amount of data (n=112) included was higher.
Developing a new QSAR, based on regression analysis between measured log Kow and experimental log BCF for 112 pesticides, would result in a similar correlation coefficient (figure 15):
log BCF = 0.3324 log Kow + 0.7545 |
(n = 112, r² = 0.502). |
The QSAR is still inaccurate but being at the same level as Veith et al. (1979) and may be used for screening purposes.
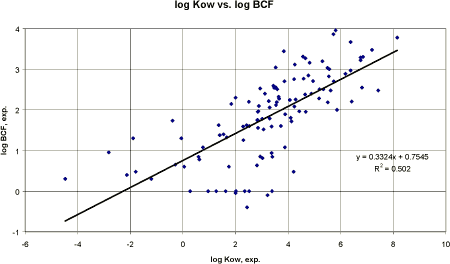
Figure 15
Correlation between experimental log Kow and experimental log BCF based on 112 pesticides.
Korrelation mellem eksperimentel log Kow og eksperimentel log BCF baseret på 112 pesticider.
3.4.2 Bioaccumulation factor for terrestrial organisms
In water, the bioconcentration factor are based on the concentration in the aquatic organism or part of the aquatic organism (e.g. whole fish or part of fish) divided by the concentration in water presumably
under equilibrium conditions.
The maximum amount of a chemical in water is governed by the water solubility of the chemical, which can be very low. In soil, the situation is different since no saturation limit is imposed by solubility. The
biology of the soil living organism determines whether the bioavailability is a question of the water solubility of the substance or not.
Distribution of pesticides in soil from use application is rarely uniform. If applied to soil surfaces, the upper few centimetres usually contain the majority of the substance and the measured concentration
depends on the depth of the soil sampled for analysis. For instance, if the application is 1 kg/ha and assumed uniformly distributed in soil 2.5 cm, 5 cm, 10 cm or 25 cm deep with the density 1500 kg/m³,
the estimated concentration in soil would be 2.67, 1.33, 0.66 or 0.26 mg/kg soil, respectively.
Thus, bioconcentration factors for terrestrial animals on treated soil do not have the same basis for a denominator as for aquatic animals in treated water (Kenaga 1980). Because of the difficulty in
determining and deciding on a uniform soil residue denominator for BCF, very little data comparable to aquatic organisms are available for terrestrial organisms.
Earthworms
For the assessment of secondary poisoning in the terrestrial food chain, the bioconcentration factor in worms is necessary. The bioconcentration factor is defined as:
BCFearthworm = Cearthworm / C pore water
As the concentration in soil and the concentration in pore water are related through the Koc where Koc = Csoil(oc) / Cpore water, the calculation could be performed using the concentration in soil and the
soil/water partition coefficient assuming steady state:
BCFearthworm = (Cearthworm Koc) / Csoil(oc)
QSAR
Finally, the QSAR models may be used. Van Gestel and Ma (1988) determined the BCF for two species of earthworms for five chlorophenols and found a relationship with log Kow. Connel and Markwell
(1990) increased the data amount from literature and developed a model based on log Kow. The model was generated on data on pesticides with a log Kow range of 1-6. The BCF-log Kow relationship
applies generally to neutral organic substances, which are not easily biotransformed. The relationship is not valid for ionised substances and organometals. The model needs further validation.
The QSAR for BCF in earthworms developed by Connel and Markwell (1990) is presented below:
log BCF = 1.0 log Kow - 0.6 |
(n=100, r²=0.91) |
Pesticides
Only a very few data exist on BCF in earthworms for pesticides that are precise enough to be used in a comparison. Therefore, the accuracy of the estimation from the QSAR by Connel and Markwell
(1990) could not be compared.
3.5 Aquatic toxicity
3.5.1 QSAR models on aquatic ecotoxicity
Within the aquatic ecotoxicology, QSAR models have been used to estimate biological effects of various chemical substances. For instance, frequently the octanol-water coefficient (log Kow) of a substance
has been used to estimate the ecotoxicity potential of the substance to organisms.
Most literature on developing QSARs for toxicity estimations has assumed that compounds from the same chemical class should behave in a toxicologically similar manner (cf. section 3.1). Recent literature
indicates that similarity in mode of toxic action is not necessarily related to typical chemical classification but to molecular structures. As a consequence, QSAR development and application have been
evolving from a chemical class perspective to one that is more consistent with assumptions regarding modes of toxic action (TGD 1996, Russom et al. 1997).
For instance, Russom et al. (1997) have performed a classification of chemicals into 8 modes of toxic action based on fathead minnow (Pimephales promelas) exposed in 96 hours flow-through studies to
617 chemicals.
The Russom et al.(1997) classification on modes of toxic action included:
- base-line narcosis or narcosis I
- polar narcosis or narcosis II
- ester narcosis or narcosis III
- oxidative phosphorylation uncoupling
- respiratory inhibition
- electrophile/proelectrophile reactivity
- acetylcholinesterase inhibition
- several mechanisms of CNS seizure responses
A few comments are given below on the most essential action mechanisms relating to pesticides.
Base-line narcosis or non-polar narcosis
Base-line toxicity or the “minimum toxicity” is related to the hydrophobicity of the substance and is also referred to as non-polar narcosis. In absence of specific toxic mechanisms, the internal effect
concentration is almost constant and a substance will then be as toxic as predicted by its hydrophobicity due to the relation with bioconcentration (McCarthy and MacKay 1993). Indications of non-polar
narcosis are the change of EC50 over time. The ratio EC50 (24 hours)/EC50 (96 hours) approximately 1.0 is considered indicative of non-polar narcosis. Excess toxicity values, calculated by dividing
predicted “narcosis I” EC50 values by the observed values, greater than 10 indicate that the substance does not act by non-polar narcosis (Russom et al. 1997). Examples of pesticides classified as having
the toxic mode of action of non-polar narcosis are alachlor, bromacil and diuron.
Polar narcosis
The polar narcosis class consists of more polar chemicals such as phenols, esters and anilines. The mode of action of these substances is not very specific but they are significantly more toxic than predicted
by non-polar narcosis.
Acetylcholinesterase inhibition
Several insecticides are acting by acetylcholinesterase inhibition, e.g. carbamates and organophosphorous pesticides. Examples of pesticides are azinphos-methyl, carbaryl, carbofuran, chlorpyriphos,
diazinon, malathion and methomyl.
CNS seizure responses
Central Nervous System (CNS) seizure/stimulant responses. In literature and handbooks, the mode of action is often called contact action or contact and stomach action. Examples of pesticides are
organochlorines and pyrethroids such as dicofol, fenvalerate and permethrin (Russom 1997).
QSARs for the acute and long term effects on fish, daphnia and algae are present for chemicals that act by non-specific mode of action (non-polar narcosis as well as polar narcosis).
The latest evaluation of current models in ecotoxicity resulted in the QSAR models mentioned in the tables 7 and 8 (Verhaar et al. 1992, 1995).
Table 7
QSARs for non-polar narcosis (Verhaar et al. 1995, TGD 1996).
QSAR for ikke-polær narkotisk virkende stoffer (Verhaar et al. 1995, TGD 1996).
Species |
Regression equation (mol/l) |
Statistics |
n |
r² |
SE |
Fish Pimephales promelas |
log LC50 (96h) = -0.85 log Kow - 1.39 |
58 |
0.94 |
0.36 |
Daphnia: Daphnia magna |
log EC50 (48h) = -0.95 log Kow - 1.32 |
49 |
0.95 |
0.34 |
Daphnia magna (repro.) |
log NOEC (16d) = -1.05 log Kow - 1.85 |
10 |
0.97 |
0.39 |
Algae: Selenastrum capricornutum |
log EC50 (72-96h) = -1.0 log Kow - 1.23 |
10 |
0.93 |
0.17 |
The models are generated by linear regression analysis. The experimental data were generated according to OECD test guidelines or comparable methods.
QSAR models for chemicals, which act by polar narcosis (esters, phenols and anilines), are also available. The mode of action of these compounds is also not very specific but they are significantly more
toxic than predicted by non-polar narcosis.
Table 8
QSARs for polar narcosis (Verhaar et al 1995, TGD 1996).
QSAR for polær narkotisk virkende stoffer (Verhaar et al. 1995, TGD 1996).
Species |
Regression equation (mol/l) |
Statistics |
n |
r² |
SE |
Fish Pimephales promelas |
log LC50 (96h) = -0.73 log Kow - 2.16 |
86 |
0.90 |
0.33 |
Daphnia: Daphnia magna |
log EC50 (48h) = -0.56 log Kow - 2.79 |
37 |
0.73 |
0.37 |
The models were generated by linear regression analysis. The experimental data were generated according to OECD test guidelines or comparable methods.
Classification
For classification purposes, the Danish EPA has developed a programme to estimate the minimum acute aquatic toxicity: QTOXMIN. The programme QTOXMIN is available in Pedersen et al. (1995) and
in Pedersen and Falck (1997).
However, the use of QSAR data for classification is currently under discussion. At this stage it is proposed that the use of QSAR estimations for base-line acute aquatic toxicity should be limited and only
used if the prerequisites below are fulfilled (Pedersen et al. 1995):
- No measured data on acute aquatic toxicity are available even after the data collection procedure outlined in Pedersen et al. (1995) has been followed.
- Estimated QSAR-based effect concentrations on the three species fish, daphnia and/or algae are not above either the measured or estimated water solubility value.
- The estimated QSAR-based effect concentration on fish, daphnia or algae is below 1 mg/l.
The QSAR equations recommended are (results in mol/l):
Fish: |
log LC50 (96h) = - 0.85 log Kow - 1.41 |
n=68, r²= 0.94, SE=0.35 |
Daphnia: |
log EC50 (48h) = - 0.95 log Kow - 1.19 |
n=17, r²=0.99, SE=0.21 |
Algae: |
log EC50 (72h) = - 1.0 log Kow - 1.23 |
n=10, r²=0.93, SE=0.17 |
The equations are applicable for neutral, organic, non-reactive and non-ionisable compounds such as alcohols, ketones, ethers, alkyl and aryl halides, and can also be used for aromatic hydrocarbons,
halogenated aromatic and aliphatic hydrocarbons as well as sulphides and disulphides (Pedersen et al. 1995).
Generally, the differences in acute toxicity between non-polar and polar narcotic acting substances are only significant for substances with a log Kow value less than 2.7. Thus, for polar acting narcotic
substances with a log Kow value above 2.7, the presented QSAR equations can also be used as a reliable predictor of their acute aquatic toxicity. For polar narcotic acting substances with a log Kow value
less than 2.7, and especially for reactive substances and for substances with a specific mode of action, the presented QSAR equations will significantly underestimate the acute toxicity (Pedersen et al. 1995,
Pedersen and Falck 1997).
The QSAR equations recommended for classification are the same or almost the same as the equations recommended in TGD (1996), which are used in the estimations of aquatic toxicity of pesticides in this
report.
Pesticides
It is important to realise that most of the QSARs developed and the QSARs presented above are based on chemicals that are biased toward industrial organic chemicals, which are not overtly designed to
have biological activity. Pesticides are mostly reacting in a specific mode of action and no QSARs have been recommended for substances that act by more specific modes of action (TGD 1996).
3.5.2 Correlations between experimental and estimated ecotoxicity
Base-line toxicity correlations
In figure 16, the correlations on estimated acute toxicity in the aquatic organisms where estimated values are based on the QSARs for non-polar narcosis substances are presented. It is primarily performed
to illustrate the concept of “minimum” toxicity than estimated EC50 values as only few pesticides have been identified as non-polar narcosis and then on a level D confidence, e.g. diuron, bromacil and
alachlor (Rossum et al. 1997). For comparison, EC50-values have been analysed using both QSAR for polar as well as for non-polar substances. The estimated values in mol/l are recalculated to mg/l by
multiplying with molecular weight and a factor 1000.
Fish
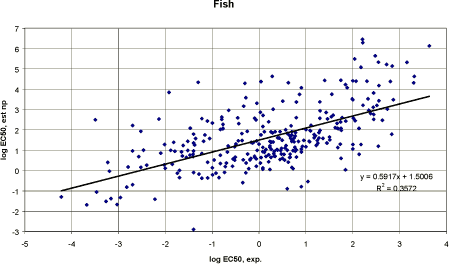
Figure 16
Correlation between experimental and estimated EC50-values (log) with non-polar narcosis type QSAR.
Korrelation mellem eksperimentelle og estimerede EC50-værdier (log) med ikke-polær narkosetype QSAR).
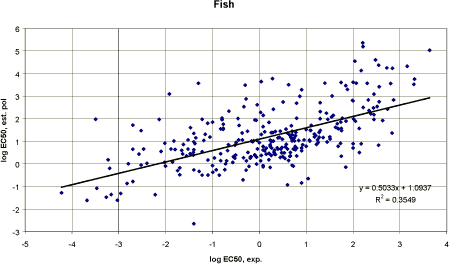
Figure 17
Fish: Correlation between experimental EC50 and estimated EC50 based on polar narcosis type QSAR.
Fisk: Korrelation mellem eksperimentel EC50 - og estimerede EC50 værdier baseret på QSAR for polært narkotisk virkende stoffer.
As expected, the estimated values on acute fish toxicity are overestimated i.e. above the trend line with intercept 0 and the slope 1. Thus, the true EC50-value is below the estimated value. For chemicals
with a specific mode of action, the effect concentration is generally between 10 and 10000 times lower than predicted by baseline toxicity QSAR equations (Verhaar et al. 1992). The same applies to
daphnia and algae (cf. below).
The linear regression analysis of the relationship between the experimental log EC50 and the estimated log EC50 for fish resulted in a correlation coefficient r² of 0.35 based on n = 297. The result was
approximately the same irrespective of whether the non-polar or the polar QSAR was used. It is far from satisfactory but the QSAR model may be used for screening purposes.
Daphnia
For comparison, linear regression analyses of the relationship between the experimental log EC50 and the estimated log EC50 for daphnia are performed for both non-polar and polar narcosis type QSARs
(figures 18 and 19). The correlation coefficient r² for non-polar narcosis type QSAR was 0.28 and the correlation coefficient r² for polar narcosis type QSAR was 0.27. The number of data sets was 255.
The result indicates that the available QSAR models for estimating acute toxicity to daphnia are not acceptable for pesticides in general at present.
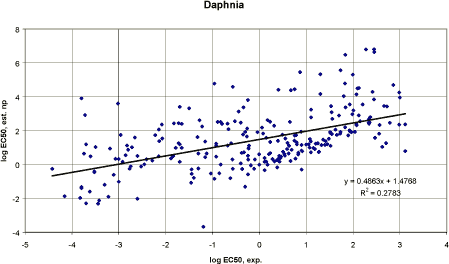
Figure 18
Daphnia: Correlation between experimental EC50 and estimated EC50 values based on non-polar narcosis type QSAR.
Dafnier: Korrelation mellem eksperimentelle EC50 og estimerede EC50 -værdier baseret på QSAR for ikke-polære narkotisk virkende stoffer.
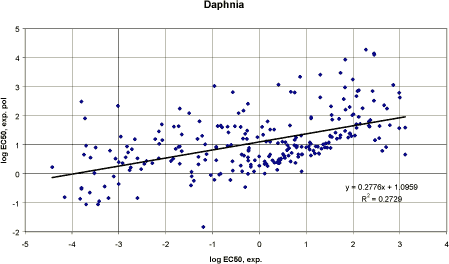
Figure 19
Daphnia: Correlation between experimental EC50 and estimated EC50 values based on polar narcosis type QSAR.
Dafnier: Korrelation mellem eksperimentelle EC50 og estimerede EC50 -værdier baseret på QSAR for polære narkotisk virkende stoffer.
Daphnia, chronic
Long-term effect studies (16 days) on Daphnia have also been used to develop a QSARs on daphnia reproduction.
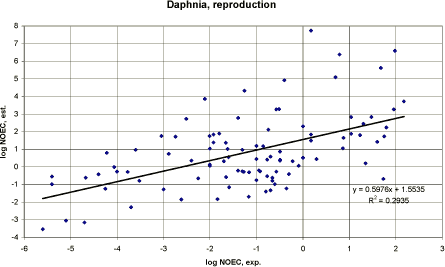
Figure 20
Correlation between experimental Daphnia NOEC (16-21 days) and NOEC values based on non-polar narcosis type QSAR.
Korrelation mellem eksperimentel dafnie NOEC (16-21 dage) og NOEC værdier baseret på QSAR for ikke-polære narkotisk virkende stoffer.
A linear regression analysis of the relationship between the log NOEC from experimental Daphnia reproduction tests and the currently recommended QSAR (table 7) resulted in a correlation coefficient r² of
0.29 based on n = 96 (figure 20). The correlation was lower than the recommended QSAR which was based on n=10. It should also be noted that the QSAR for estimating daphnia reproduction NOEC
was based on 16-day tests and the experimental results in this report are mainly from 21-day tests. This could reduce the comparability. The result indicates that the used QSAR model for estimating chronic
Daphnia NOEC values is not recommendable for pesticides in general.
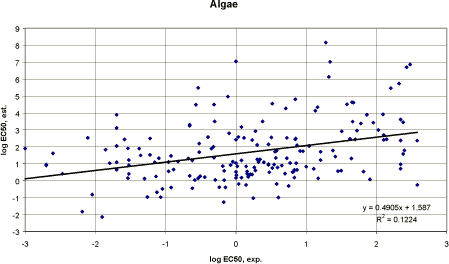
Figure 21
Correlations between the experimental log EC50 and estimated log EC50 for algae (non-polar narcosis).
Korrelation mellem eksperimentel EC50 og estimeret EC50 -værdier for alger (ikke-polær narkotisk virkende stoffer).
Algae
Linear regression analyses of the relationship between the experimental log EC50 and the estimated log EC50 for algae for non-polar type QSARs (figure 21) resulted in the correlation coefficient r² 0.12.
The number of data sets was 193. For algae, the correlation was so low that it must be concluded that the resulting estimations are unacceptably poor.
Discussion
The situation with the present data set is that it has been chosen as a mixture of different pesticides to evaluate whether the evolved QSARs could be used in general. The general conclusion is that the results
demonstrate that the available QSAR models should be used with great precaution, as they are not developed specifically for pesticides.
Individual results of the estimation can be found in the appendix. Generally, the results support the concept of minimum toxicity, i.e. if the substance is estimated to be toxic the experimental results may be
expected to confirm that the substance is more toxic than estimated.
If the pesticides were selected according to function, mode of action or chemical class another result might have appeared. The following two sections presents examples of QSARs developed for specific
pesticides (3.5.3) and the QSARs derived from the pesticides used in the report (3.5.4).
3.5.3 QSARs developed for specific pesticides
Two examples of developed QSAR models for pesticides from the same chemical class and assumed same mode of action are presented below.
Phenylureas
Phenylurea herbicides have been selected as model compounds representing a well-defined class of chemical structure mostly acting by a uniform mode of action by Nendza (1991) and Nendza et al.
(1991). Satisfactory estimates on the phenylureas fish toxicity were obtained from a log Kow dependent QSAR. The toxicity to algae and plants which were the most sensitive species corresponding to the
mode of action (interacts with the electron transport chain in the photolysis reactions) could be estimated by including the Hill reaction inhibition in the QSAR. Nendza et al. (1991) used the following
equations with satisfactory results:
Fish: log 1/LC50 (mmol/l) = 0.62 log Kow - 0.56 (n=118, r=0.91, s=30)
QSARs derived for non-reactive chemicals underestimate the effects of phenylureas on algae significantly. Regarding their mode of action, the inhibition of algae photosystem should be included. Nendza et
al. (1991) presents a QSAR modelling the interaction with the electron transport chain (pI50 Hill reaction) giving acceptable estimates of the phenylureas' algae toxicity by including the ionisation potential
(IP) besides log Kow as descriptors:
Algae: pI50 = 0.93 log Kow + 0.69 IP - 3.03 (n=12, r= 0.97, s= 0.37)
In Nendza (1991), the equation on algae toxicity is changed and instead tabulated electronic properties () is employed:
Algae (log 1/[mmol/l]):
log 1/C = 1.89 log Kow - 0.17 log Kow² - 0.65 - 0.66
The results from Nendza et al. (1991) are presented in table 9.
For algae, it was observed that the estimated EC50 values were always below the experimental values (cf. table 9).
Table 9
Phenylurea herbicides log Kow and experimental (exp.) and estimated (calculated.) toxicity (log 1/(mmol/l) (Nendza et al. 1991).
Phenylurea herbiciders log Kow, eksperimentel (exp.) og estimeret (calc.) toksicitet (log 1/(mmol/l) (Nendza et al. 1991).
Compound |
Log Kow |
Fish log LC50 |
Algae log EC50 |
Exp. |
Calc. |
Exp. |
Calc. |
Buturon |
1.6 |
2.57 |
0.43 |
3.13 |
1.94 |
Chlorbromuron |
3.05 |
2.82 |
1.33 |
5.17 |
3.50 |
Chloroxuron |
4.00 |
0.80 |
1.92 |
5.08 |
4.03 |
Chlorotoluron |
2.53 |
-0.06 |
1.01 |
|
2.86 |
Defenuron |
1.10 |
1.55 |
0.12 |
|
1.36 |
Diuron |
2.75 |
-0.57 |
1.15 |
5.33 |
3.23 |
Fenuron |
0.87 |
0.82 |
-0.02 |
|
1.17 |
Isoproturon |
2.44 |
1.36 |
0.95 |
|
2.60 |
Fluometuron |
2.28 |
1.19 |
0.85 |
3.82 |
2.91 |
Linuron |
3.18 |
0.85 |
1.41 |
|
3.69 |
Metobromuron |
2.46 |
1.06 |
1.06 |
3.51 |
2.81 |
Metoxuron |
1.68 |
0.46 |
0.48 |
|
2.02 |
Monolinuron |
2.31 |
0.42 |
0.87 |
3.24 |
2.71 |
Monuron |
1.88 |
|
0.61 |
3.94 |
2.27 |
Neburon |
4.09 |
0.22 |
1.98 |
5.06 |
4.51 |
Siduron |
3.65 |
|
1.70 |
|
3.74 |
Organophosphorous
QSARs have also been developed for the toxicity of organophosphorous pesticides to Daphnia (Vighi et al. 1991). The 22 tested chemicals were belonging to the subclasses: phosphates,
phosphorothionates, phosphorodithionates, and phosphonates. They all had a specific mode of action: cholinesterase inhibition. The QSARs were obtained by multilinear correlations with independent
variables: log Kow, first order valence molecular connectivity (1χv) and complementary information content (CIC) as independent variables for a number of chemical substances.
For Daphnia, the best equation obtained was:
log 1/EC50 = 0.55 log Kow - 0.085 (log Kow)² - 3.93IC -0.016 (1χvox)² + 0.13α3 - 0.0006(α3)² + 4.58
(n=22, r²=0.895, s=0.67)
where the molecular connectivity indices developed by Hall and Kier (1981) and information index of neighbourhood symmetry, IC (information content), were used as descriptor of topological information. Valence molecular connectivity indices encode information on volumetric and electronic characteristics of the bonds in the molecule and are also calculated for the oxygen metabolites, 1χvox. The variable 3 describes the electronegativity of the leaving group in the acetylenesterase inhibition reaction (Vighi et al. 1991). The approach was considered satisfactory in describing the toxicity of a relatively
heterogeneous set of organophosphorous compounds after introduction of variables able to describe the particular reactivity of these compounds characterised by a highly specific biological activity.
3.5.4 QSARs derived from pesticides in the report
Based on the approximately 400 pesticides including some salts and esters selected for this study (cf. appendix), it was decided to study whether dividing the pesticides into minor groups would improve the
predictability. Furthermore, it was decided to use the mode of action as basis. At first the pesticides were divided into five main groups and then further subdivided if sufficient data were present:
Fungicides |
|
Herbicides |
Growth inhibitors Photosynthesis inhibitors |
Insecticides |
Cholinesterase inhibitors Contact and stomach action (CSA) |
Plant growth regulators (PGR) |
|
Rodenticides |
|
Linear regression analyses were performed between the experimental EC50 values and the experimental log Kow. The analyses were performed using both mg/l and mmol/l on account of the general idea
that the molecular weight influenced the effect level. Graphic presentations of the correlations between experimental EC50-values and log Kow are presented below for fish, daphnia and algae.
Fish
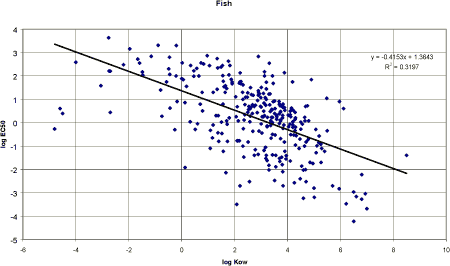
Figure 22
Correlation between experimental log Kow and experimental EC50 for fish (n=300) for all pesticides in the appendix.
Korrelation mellem eksperimentel log Kow og eksperimentel EC50 for fisk (n=300) for alle pesticider i appendiks.
Using all the selected pesticides and performing a linear regression analysis of the correlation between experimental log Kow and the acute toxicity (cf. figure 22), the resulting equation for fish was:
log EC50 (mg/l) = -0.4153 log Kow + 1.3643 |
(n=300, r² = 0.3197) |
The reduced correlation compared with the previous section may be the result of data from several fish species and a broader variety of pesticides included in the calculation. However, a trend is obvious and
the result may be sufficient for a screening procedure.
Removing the data with the lowest log Kow values (log Kow values 4) improved the correlation coefficient slightly (Figure 23). The resulting equation for acute fish toxicity was then:
log EC50 (mg/l) = -0.5076 log Kow + 1.6739 |
(n=296, r² = 0.3786) |
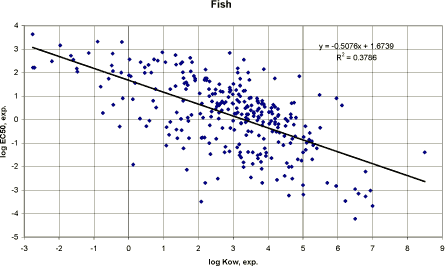
Figure 23
Correlation between experimental log Kow and experimental EC50 for fish (n=296).
Korrelation mellem eksperimentel log Kow og eksperimentel EC50 for fisk (n=296).
Many of the literature QSARs are presented using the mol/l instead of mg/l in the models estimating toxicity. It indicates that the molecular size or properties related to the molecular weight is important to the
estimated toxicity. As the argument seems reasonable a recalculation of the measured data to mmol/l was performed. The result is presented in figure 24.
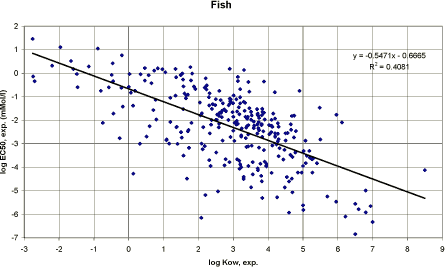
Figure 24
Correlation between log Kow and experimental EC50 for fish (n=296) in mmol/l.
Korrelation mellem log Kow og eksperimentel EC50 for fisk (n=296) mmol/l.
The resulting equation for acute fish toxicity was:
log EC50 (mmol/l) = 0.5471 log Kow - 0.6666 |
(n=296, r² = 0.4081) |
The correlation coefficient was slightly higher. The reason was probably that the variation of molecular weights for the used pesticides was relatively narrow (299±103 g/mol, n=409). Using mmol/l instead of
mg/l had minor effect on the correlation coefficient. Mainly results from mmol/l are shown in the figures and both equations are presented in the text.
Mode of action
The data on fish acute toxicity were divided into:
pH of soil and water
- herbicides with a growth inhibition mode of action
- herbicides with a photosynthesis mode of action
- insecticides
- insecticides with a cholinesterase inhibition mode of action
- insecticides with a contact and systemic action
- plant growth regulators
- rodenticides.
The axes in the following charts are kept constant for comparison.
For fungicides, 59 corresponding experimental log Kow and EC50 values were found. In figure 25, a trend is indicated but the dispersion is high. This could be expected since fungicides do not have a specific action mode towards fish.
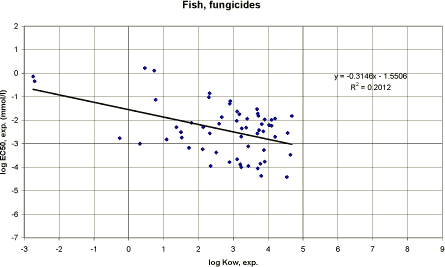
Figure 25
Correlation between log Kow and experimental EC50 for fish using data on fungicides (n=59).
Korrelation mellem log Kow og eksperimentel EC50 for fisk med data for fungicider (n=59).
The resulting equations for acute fish toxicity using fungicide data were:
log EC50 (mg/l) = -0.2963 log Kow + 0.8584 |
(n=59, r² = 0.1890) |
log EC50 (mmol/l) = -0.3146 log Kow - 1.5506 |
(n=59, r² = 0.2012) |
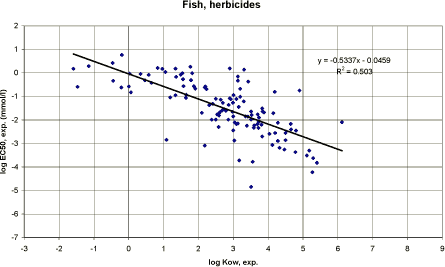
Figure 26
Correlation between log Kow and experimental EC50 for fish using data on herbicides (n=118).
Korrelation mellem log Kow og eksperimentel EC50 for fisk ved anvendelse af herbicid data (n=118).
For herbicides, all available data on experimental log Kow and fish EC50 were used and presented in figure 26. The resulting equations for acute fish toxicity using herbicide data were:
log EC50 (mg/l) = -0.5193 log Kow + 2.3548 |
(n=118, r² = 0.4814) |
log EC50 (mmol/l) = -0.5337 log Kow - 0.0459 |
(n=118, r² = 0.5030) |
The data on herbicides was divided into herbicides with a growth inhibition mode of action and herbicides with a photosynthesis inhibition mode of action.
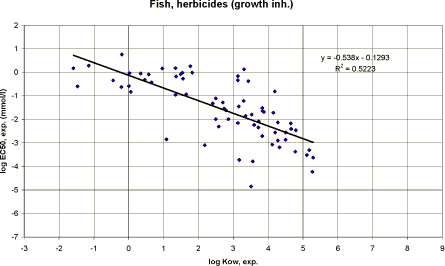
Figure 27
Correlation between log Kow and experimental EC50 for fish using data on herbicides with growth inhibition mode of action (n=69).
Korrelation mellem log Kow og eksperimentel EC50 for fisk ved anvendelse af herbicid data med væksthæmmende virkningsmekanisme (n=69).
The resulting equations for acute fish toxicity using data on herbicides with a growth inhibition mode of action (cf. figure 27) were:
log EC50 (mg/l) = -0.6344 log Kow + 2.3145 |
(n=69, r² = 0.5122) |
log EC50 (mmol/l) = -0.538 log Kow - 0.1293 |
(n=69, r² = 0.5223) |
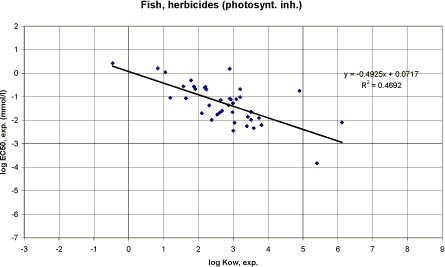
Figure 28
Correlation between log Kow and experimental EC50 for fish using data on herbicides with photosynthesis inhibition mode of action (n=40).
Korrelation mellem log Kow og eksperimentel EC50 for fisk ved anvendelse af data for herbicider med fotosyntesehæmmende (n=40). virkningsmekanisme.
The resulting equations for acute fish toxicity using data on herbicides with a photosynthesis inhibition mode of action (cf. figure 28) were:
log EC50 (mg/l) = -0.4394 log Kow + 2.3336 |
(n=40, r² = 0.3913) |
log EC50 (mmol/l) = -0.4925 log Kow + 0.0717 |
(n=40, r² = 0.4692) |
Compared to the result using fungicides, the estimations on fish acute toxicity using herbicide data were improved. The correlation coefficients for herbicides with a growth inhibition mode of action were
slightly higher than for herbicides characterised with a photosynthesis inhibition mode of action.
The two modes of action is based on action modes taking place in plants and would be expected to be meaningless to fish. However, some herbicides are toxic to fish and the reason may be a general toxic
action or a specific toxic action to enzymatic systems in fish. Whatever the reason, log Kow data on herbicides seems to an acceptable variable to estimate the general acute toxicity to fish.
For insecticides, all the data on experimental log Kow and acute toxicity are used and presented in figure 29. The data are then divided into insecticides with a cholinesterase inhibition mode of action and
insecticides with a contact and systemic mode of action.
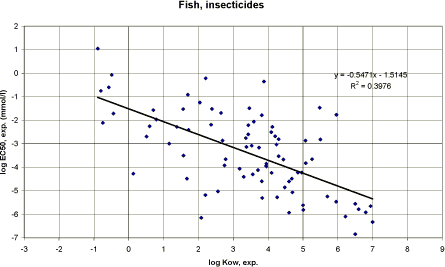
Figure 29
Correlation between log Kow and experimental EC50 for fish using data on insecticides (n=81).
Korrelation mellem log Kow og eksperimentel EC50 for fisk ved anvendelse af insekticiddata (n=81).
The equations for acute fish toxicity using insecticide data were:
log EC50 (mg/l) = -0.4998 log Kow + 0.8155 |
(n=81, r² = 0.3652) |
log EC50 (mmol/l) = -0.5471 log Kow - 1.5145 |
(n=81, r² = 0.3976) |
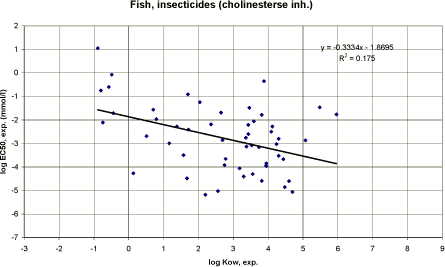
Figure 30
Correlation between log Kow and experimental EC50 for fish using data on insecticides with cholinesterase inhibition mode of action (n=53).
Korrelation mellem log Kow og eksperimentel EC50 for fisk ved anvendelse af insekticiddata for insekticider med cholinesterasehæmmende virkningsmekanisme (n=53).
The equations for acute fish toxicity using data on insecticides with a cholinesterase inhibition mode of action were:
log EC50 (mg/l) = -0.2903 log Kow + 0.4699 |
(n=53, r² = 0.1403) |
log EC50 (mmol/l) = -0.3334 log Kow - 1.8695 |
(n=53, r² = 0.1750) |
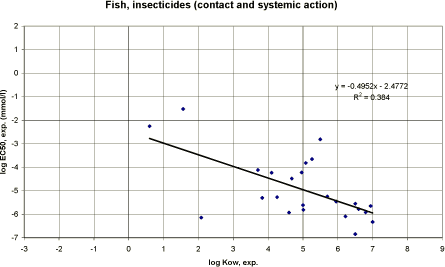
Figure 31
Correlation between log Kow and experimental EC50 for fish using data on insecticides with contact and systemic mode of action (n=24).
Korrelation mellem log Kow og eksperimentel EC50 for fisk ved anvendelse af data for insekticider med kontakt og systemisk virkningsmekanisme (n=24).
The equations for acute fish toxicity using data on insecticides with a contact and systemic mode of action were:
log EC50 (mg/l) = -0.4515 log Kow - 0.111 |
(n=24, r² = 0.3690) |
log EC50 (mmol/l) = -0.4952 log Kow - 2.4772 |
(n=24, r² = 0.3840) |
Comparing the figures on fungicides, herbicides and insecticides, it can be observed that the data points occupy a lower position in the charts that indicate a higher toxicity of insecticides to fish. This is not
surprising since for instance a cholinesterase inhibition mode of action should also affect fish.
The data points are widely scattered which result in a low correlation coefficient. However, it should be remembered that several fish species, laboratories etc. are included and that it is the lowest
experimental values found on acute toxicity that are used as effect data. These factors may result in the observed scattering of data points.
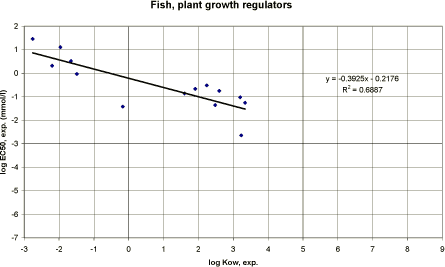
Figure 32
Correlation between log Kow and experimental EC50 for fish using data on plant growth regulators (n=14).
Korrelation mellem log Kow og eksperimentel EC50 for fisk ved anvendelse af data fra plantevækst regulatorer (n=14).
For plant growth regulators, only few data sets were available (cf. figure 32). The equations for acute fish toxicity using plant growth regulator data were:
log EC50 (mg/l) = -0.3391 log Kow + 2.054 |
(n=14, r² = 0.6495) |
log EC50 (mmol/l) = -0.3925 log Kow - 0.2176 |
(n=14, r² = 0.6887) |
The correlation coefficient was high and the estimations on toxicity based on log Kow were acceptable for plant regulators.
The data points are located in the upper end of the chart indicating lower toxicity than insecticides. It is not surprising since plant growth regulators usually affects meristematic tissues. The result could be
compared with the results on rodenticides (cf. figure 33) where a high correlation coefficient was also observed.
The equations for acute fish toxicity using rodenticide data were:
log EC50 (mg/l) = -0.5838 log Kow + 2.7154 |
(n=5, r² = 0.6390) |
log EC50 (mmol/l) = -0.617 log Kow + 0.2194 |
(n=5, r² = 0.6228) |
It should be noted that the regression equations on both plant regulators and rodenticides are based on few data sets and should be used with this in mind.
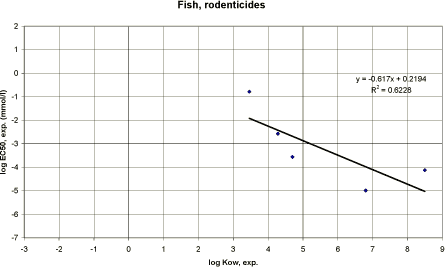
Figure 33
Correlation between log Kow and experimental EC50 for fish using rodenticides (n=5).
Korrelation mellem log Kow og eksperimentel EC50 for fisk med rottemidler (n=5).
Daphnia
For daphnia the same procedure as in fish was repeated using the same subdivision of pesticides.
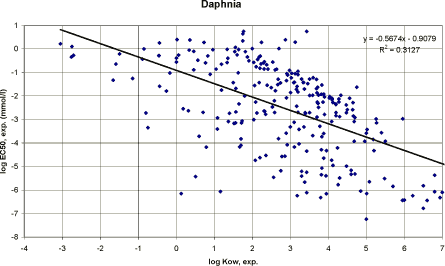
Figure 34
Correlation between log Kow and experimental EC50 values for Daphnia (n=255).
Korrelation mellem log Kow og eksperimentelle EC50-værdier for dafnier (n=255).
Using all the selected pesticides and performing a regression analysis of the correlation between the experimental log Kow and the acute toxicity, the resulting equations for estimating acute daphnia toxicity
were:
log EC50 (mg/l) = - 0.5166 log Kow + 1.39 |
(n=255, r² = 0.2743) |
log EC50 (mmol/l) = - 0.5674 log Kow - 0.9079 |
(n=255, r² = 0.3127) |
The reduced correlation compared with the Verhaar et al. QSARs may be the result of data from a broader variety of pesticides, data sources and/or study methodologies included in the applied values. The
low correlation coefficient of 0.31 does not indicate a predictive value to be sufficient even for a screening procedure.
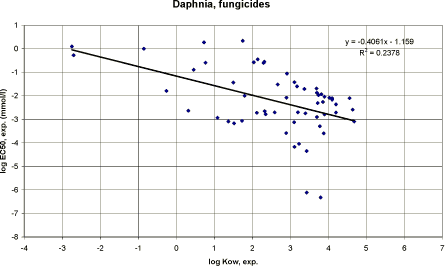
Figure 35
Correlation between log Kow and experimental EC50 for daphnia using data on fungicides (n=57).
Korrelation mellem log Kow og eksperimentel EC50 for dafnier med data for fungicider (n=57).
The resulting equations for acute daphnia toxicity using fungicide data were:
log EC50 (mg/l) = -0.3149 log Kow + 1.0021 |
(n=57, r² = 0.1518) |
log EC50 (mmol/l) = -0.4061 log Kow - 1.159 |
(n=57, r² = 0.2378) |
As with the fish data, the data points were widely dispersed and the correlation coefficient was low.
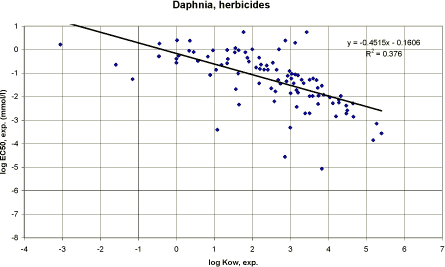
Figure 36
Correlation between log Kow and experimental EC50 for daphnia using data on herbicides (n=97).
Korrelation mellem log Kow og eksperimentel EC50 for dafnier ved anvendelse af herbicid data (n=97).
The resulting equations for acute daphnia toxicity using all available herbicide data (cf. figure 36) were:
log EC50 (mg/l) = -0.4388 log Kow + 2.2407 |
(n=97, r² = 0.3578) |
log EC50 (mmol/l) = -0.4515 log Kow - 0.1606 |
(n=97, r² = 0.3760) |
The correlation coefficients were low and with a low toxicity as indicated by the position on the chart (figure 36). The same comments on expected toxicity presented for fish above also applies to daphnia.
The herbicide data were divided into herbicides with a growth inhibition mode of action (cf. figure 37) and herbicides with a photosynthesis inhibition mode of action (cf. figure 38).
The resulting equations for acute daphnia toxicity using data on herbicides with a growth inhibition mode of action were:
log EC50 (mg/l) = -0.4263 log Kow + 2.1565 |
(n=58, r² = 0.3344) |
log EC50 (mmol/l) = -0.4344 log Kow - 0.2771 |
(n=58, r² = 0.3547) |
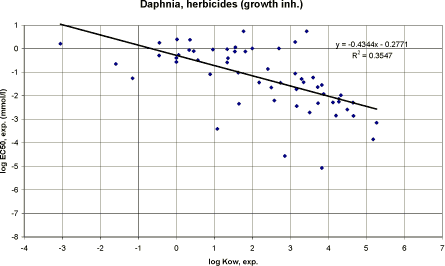
Figure 37
Correlation between log Kow and experimental EC50 for daphnia using data on herbicides with growth inhibition mode of action (n=58).
Korrelation mellem log Kow og eksperimentel EC50 for dafnier ved anvendelse af data fra herbicider med væksthæmmende virkningsmekanisme.
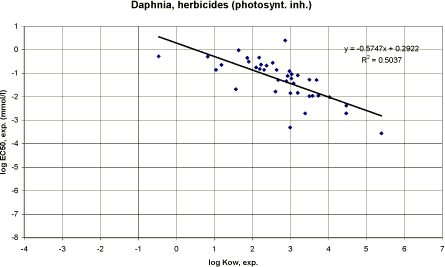
Figure 38
Correlation between log Kow and experimental EC50 for daphnia using data on herbicides with photosynthesis inhibition mode of action (n=39).
Korrelation mellem log Kow og eksperimentel EC50 for dafnier ved anvendelse af data fra herbicider med fotosyntesehæmmende virkningsmekanisme (n=39).
The resulting equations for acute daphnia toxicity using data on herbicides with a photosynthesis inhibition mode of action were:
log EC50 (mg/l) = -0.5284 log Kow + 2.569 |
(n=39, r² = 0.4788) |
log EC50 (mg/l) = -0.5747 log Kow + 2.922 |
(n=39, r² = 0.5037) |
The correlation coefficient for data on herbicides with a photosynthesis inhibition mode of action was considerably higher than for the other data sets on herbicides. No explanation can be given. The position
of the data points indicates relatively low toxicity of herbicides to daphnia that was not unexpected.
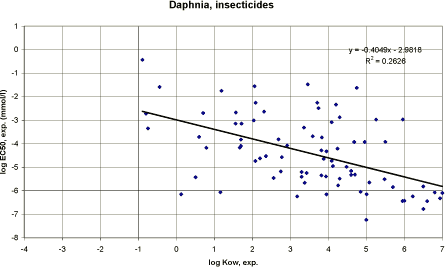
Figure 39
Correlation between log Kow and experimental EC50 for daphnia using data on insecticides (n=79).
Korrelation mellem log Kow og eksperimentel EC50 for dafnier ved anvendelse af insekticiddata (n=79).
The equations for acute daphnia toxicity using insecticide data were:
log EC50 (mg/l) = -0.3521 log Kow - 0.6848 (n=79, r² = 0.2203) |
log EC50 (mmol/l) = -0.4049521 log Kow - 2.9818 (n=79, r² = 0.2626) |
For insecticides, using all available data sets it could be observed by the position in the chart (figure 39) that insecticides were more toxic to daphnia than herbicides and fungicides as expected.
The equations for acute daphnia toxicity using data on insecticides with a cholinesterase inhibition mode of action (cf. figure 40) were:
log EC50 (mg/l) = -0.2711 log Kow - 1.1177 |
(n=43, r² = 0.1243) |
log EC50 (mmol/l) = -0.3156 log Kow - 3.4422 |
(n=43, r² = 0.1582) |
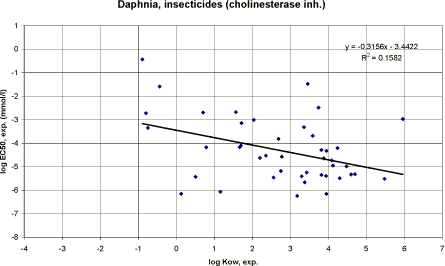
Figure 40
Correlation between log Kow and experimental EC50 for daphnia using data on insecticides with cholinesterase inhibition mode of action (n=43).
Korrelation mellem log Kow og eksperimentel EC50 for dafnier ved anvendelse af data for insekticider med cholinesterasehæmmende virkningsmekanisme (n=43).
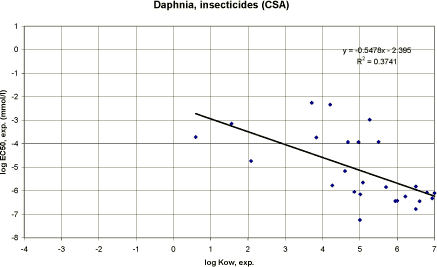
Figure 41
Correlation between log Kow and experimental EC50 for daphnia using data on insecticides with contact and systemic mode of action (n=26).
Korrelation mellem log Kow og eksperimentel EC50 for dafnier ved anvendelse af data for insekticider med kontakt og systemisk virkningsmekanisme (n=26).
The equations for acute fish toxicity using data on insecticides with a contact and systemic mode of action were:
log EC50 (mg/l) = -0.5026 log Kow - 0.043 |
(n=26, r² = 0.3465) |
log EC50 (mmol/l) = -0.5478 log Kow - 2.395 |
(n=26, r² = 0.3741) |
The data points from all insecticides were widely scattered. Dividing the data on insecticides into modes of action did not improve the correlation coefficients. Primarily data on Daphnia magna and a few
data on Daphnia pulex were included. No other crustacean species were used. Data were based on OECD standard 48-hour tests. However, test conditions, media etc. may differ and may be part of an
explanation.
For plant growth regulators, only a few data sets were available. As indicated by figure 42 they were less toxic than the insecticides which was not unexpected due to their specific mode of action on plant
meristematic tissues.
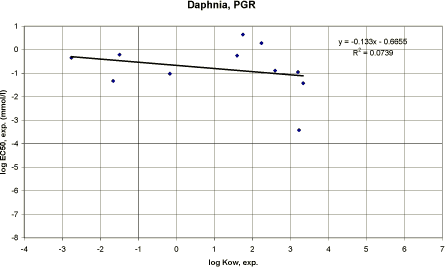
Figure 42
Correlation between log Kow and experimental EC50 for daphnia using data on plant growth regulators (n=11).
Korrelation mellem log Kow og eksperimentel EC50 for dafnier ved anvendelse af data fra plantevækst regulatorer (n=11).
The equations for acute daphnia toxicity using plant growth regulator data were:
log EC50 (mg/l) = -0.0887 log Kow + 1.6166 |
(n=11, r² = 0.0364) |
log EC50 (mmol/l) = -0.133 log Kow - 0.6655 |
(n=11, r² = 0.0739) |
The correlation was very low and the data points few. The data indicate a low toxicity of plant growth regulators to daphnia. Another parameter than Kow should be searched for as descriptor.
Daphnia reproduction
For chronic toxicity, experimental 21-day NOEC data on Daphnia magna reproduction were used. However, sufficient data were not available to perform a division further than to the main groups of
fungicides, herbicides and insecticides.
Using all available pesticide data sets on daphnia reproduction resulted in figure 43.
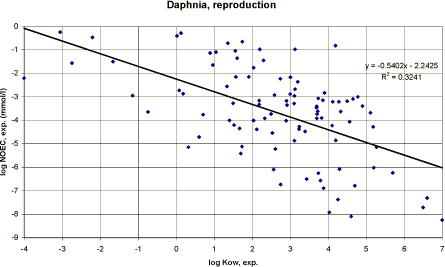
Figure 43
Correlation between experimental log Kow and experimental daphnia NOEC (21 days) values.
Korrelation mellem eksperimentel log Kow og eksperimentelle værdier for dafniers NOEC (21 dage).
Performing linear regression analyses between experimental log Kow and experimental NOEC values from daphnia reproduction tests resulted in the regression equations:
log NOEC (21d, mg/l) = -0.5052 log Kow + 0.1325 |
(n=96, r²=0.3002) |
log NOEC (21d, mmol/l) = -0.5402 log Kow - 2.2425 |
(n=96, r²=0.3241) |
The correlation coefficients were close to the correlation observed when the QSAR from Verhaar et al. (1995) was used (cf. figure 20). In other words, the above-derived QSAR model may also be used
for estimating the daphnia reproduction NOEC with the same precision as the recommended QSAR. The correlation coefficient was low. However, seeking for QSAR models to be used in general, low
correlation coefficients may be accepted and the results used with precaution for indicative purposes only.
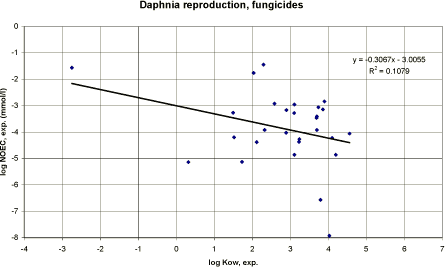
Figure 44
Correlation between experimental log Kow and experimental Daphnia NOEC (21 days) using data on fungicides.
Korrelation mellem data for eksperimentel log Kow og eksperimentel dafnie NOEC (21 dage) fra svampemidler.
The resulting equations for chronic daphnia toxicity using fungicide data were:
log NOEC (mg/l) = -0.3035 log Kow - 0.5229 |
(n=28, r² = 0.1106) |
log NOEC (mmol/l) = -0.3067 log Kow - 3.0055 |
(n=28, r² = 0.1079) |
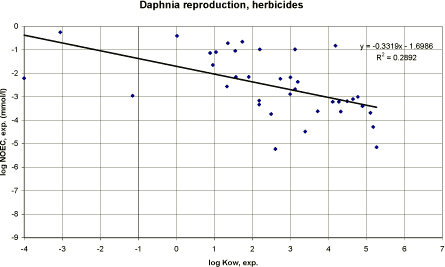
Figure 45
Correlation between experimental log Kow and experimental Daphnia NOEC (21 days) using data on herbicides.
Korrelation mellem data for eksperimentel log Kow og eksperimentel dafnie NOEC (21 dage) for herbicider.
The resulting equations for chronic daphnia toxicity using herbicide data were:
log NOEC (mg/l) = -0.3163 log Kow + 0.7216 |
(n=37, r² = 0.2647) |
log NOEC (mmol/l) = -0.3319 log Kow - 1.6986 |
(n=37, r² = 0.2892) |
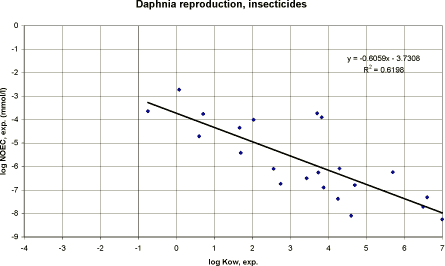
Figure 46
Correlation between experimental log Kow and experimental Daphnia NOEC (21 days) using data on insecticides.
Korrelation mellem data for eksperimentel log Kow og eksperimentel dafnie NOEC (21 dage) for insekticider.
The resulting equations for chronic daphnia toxicity using insecticide data were:
log NOEC (mg/l) = -0.5516 log Kow - 1.425 |
(n=22, r² = 0.5682) |
log NOEC (mmol/l) = -0.6059 log Kow - 3.7308 |
(n=22, r² = 0.6198) |
Data on rodenticides were insufficient for analysis to be performed.
For estimating the chronic toxicity to daphnia using daphnia reproduction NOEC as end point and log Kow as descriptor, the resulting equations on fungicides and herbicides were meaningless. This may be
the result of the designated substances' mode of action in contrast to insecticides where a toxic action is expected. The only derived equations with high correlation coefficients were data on insecticides.
Although the data points were few a clear tendency was observed. However, more data should be included before a general use of the equation is recommendable.
Algae
The same procedure as for fish and daphnia is performed on acute algae tests. The data are from tests performed according to OECD test guidelines and the end point was inhibition EC50 values after 72 to
96 hours.
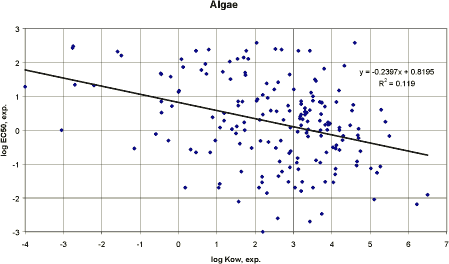
Figure 47
Correlation between log Kow and experimental EC50 values for algae.
Korrelation mellem log Kow og eksperimentelle EC50 værdier for alger.
Using all the selected pesticides and performing a regression analysis on the experimental log Kow and the acute toxicity data, the resulting equation for algae was:
log EC50 (mg/l) = - 0.2397 log Kow + 0.8195 |
(n=183, r² = 0.1190) |
The reduced correlation compared with the Van Leeuwen et al. QSARs may be the result of data from a broader variety of pesticides (Verhaar et al. n=10) and/or study methodologies included in the
applied values. The low correlation coefficient of 0.12 does not indicate a predictive value to be sufficient even for a screening procedure.
For algae, the QSAR from Verhaar et al. (1995) was expressed in mol/l where the results above were analysed using mg/l. Using mmol/l is presented in the figure below (figure 48).
Transforming the toxicity data to mmol/l, the resulting equation for algae was:
log EC50 (mmol/l) = - 0.2679 log Kow - 1.5452 |
(n=183, r² = 0.1451) |
Thus, only a minor improvement in the correlation coefficient is observed
The data points are still widely dispersed and only a weak tendency evolved from the change.
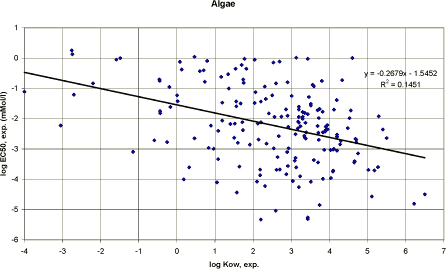
Figure 48
Correlation between log Kow and log experimental EC50 (mmol/l).
Korrelation mellem log Kow og log eksperimentel EC50 (mmol/l).
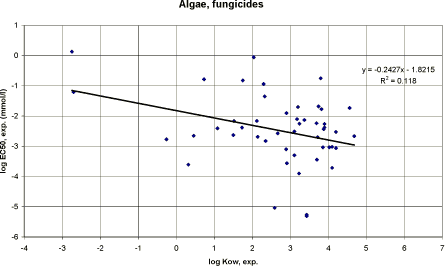
Figure 49
Correlation between log Kow and experimental EC50 for algae using data on fungicides (n=48).
Korrelation mellem log Kow og eksperimentel EC50 for alger med data for fungicider (n=48).
The resulting equations for acute algae toxicity using fungicide data were:
log EC50 (mg/l) = -0.2325 log Kow + 0.623 |
(n=48, r² = 0.1112) |
log EC50 (mmol/l) = -0.2427 log Kow - 1.8215 |
(n=48, r² = 0.1180) |
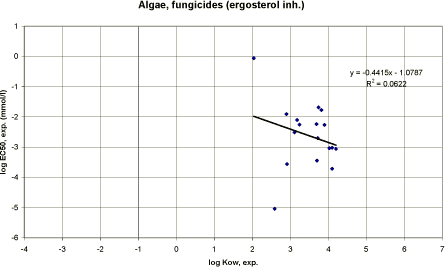
Figure 50
Correlation between log Kow and experimental EC50 for algae using data on fungicides with ergosterol inhibition mode of action (n=17).
Korrelation mellem log Kow og eksperimentel EC50 for alger med data for fungicider med ergosterolhæmmende virkningsmekanisme (n=17).
The resulting equations for acute algae toxicity using fungicide data on fungicides with ergosterol inhibition mode of action were:
log EC50 (mg/l) = -0.4549 log Kow + 1.4752 |
(n=17, r² = 0.0629) |
log EC50 (mmol/l) = -0.4415 log Kow - 1.0787 |
(n=17, r² = 0.0622) |
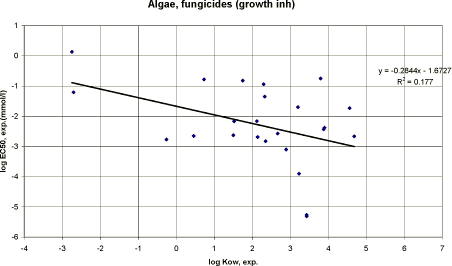
Figure 51
Correlation between log Kow and experimental EC50 for algae using data on fungicides with growth inhibition mode of action (n=24).
Korrelation mellem log Kow og eksperimentel EC50 for alger med data for fungicider med væksthæmmende virkningsmekanisme (n=24).
The resulting equations for acute algae toxicity using fungicide data on fungicides with a growth inhibition mode of action were:
log EC50 (mg/l) = -0.2669 log Kow + 0.7178 |
(n=24, r² = 0.1605) |
log EC50 (mmol/l) = -0.2844 log Kow - 1.6727 |
(n=24, r² = 0.1770) |
Based on fungicides, neither all available data nor a selection of data on fungicides with ergosterol inhibition mode of action resulted in any usable regression equations. The data points were scattered and no
tendency could be observed.
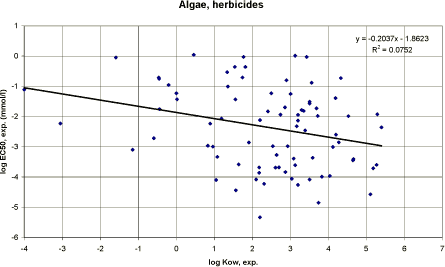
Figure 52
Correlation between log Kow and experimental EC50 for algae using data on herbicides (n=82).
Korrelation mellem log Kow og eksperimentel EC50 for alger ved anvendelse af herbiciddata (n=82).
The resulting equations for acute algae toxicity using all available herbicide data were:
log EC50 (mg/l) = -0.1898 log Kow +025345 |
(n=82, r² = 0.0636) |
log EC50 (mmol/l) = -0.2037 log Kow - 1.8623 |
(n=82, r² = 0.0752) |
It was surprising that using herbicide data sets did not show any tendency. Results from different algae species are included. Algae species are known to differ considerably in sensitivity to chemicals. Another
explanation could be that the general mode of action in herbicides does not apply to algae which normally is considered as aquatic micro-plants.
A subdivision of data on herbicides with growth inhibition (cf. figure 53) and photosynthesis inhibition mode of action (cf. figure 54) was performed.
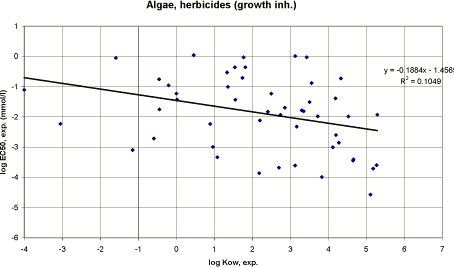
Figure 53
Correlation between log Kow and EC50 for algae using data on herbicides with growth inhibition mode of action (n=51).
Korrelation mellem log Kow og EC50 for alger ved anvendelse af data fra herbicider med væksthæmmende virkningsmekanisme (n=51).
The resulting equations for acute algae toxicity using data on herbicides with a growth inhibition mode of action were:
log EC50 (mg/l) = -0.1768 log Kow + 0.9666 |
(n=51, r² = 0.0934) |
log EC50 (mmol/l) = -0.1884 log Kow - 1.456 |
(n=51, r² = 0.1049) |
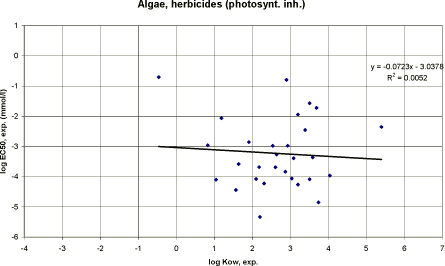
Figure 54
Correlation between log Kow and EC50 for algae using data on herbicides with photosynthesis inhibition mode of action (n=29).
Korrelation mellem log Kow og EC50 for alger ved anvendelse af data for herbicider med fotosyntesehæmmende virkningsmekanisme (n=29).
The resulting equations for acute algae toxicity using data on herbicides with a photosynthesis inhibition mode of action were:
log EC50 (mg/l) = -0.0294 log Kow - 0.7492 |
(n=29, r² = 0.0008) |
log EC50 (mmol/l) = -0.0723 log Kow - 3.0378 |
(n=29, r² = 0.0052) |
The subdivision of herbicide data sets did not improve the correlation coefficients. No acceptable regression equations could be deducted.
Regression analyses on experimental log Kow and insecticides (cf. figure 55) and selected insecticides with cholinesterase inhibition mode of action (cf. figure 56) were performed.
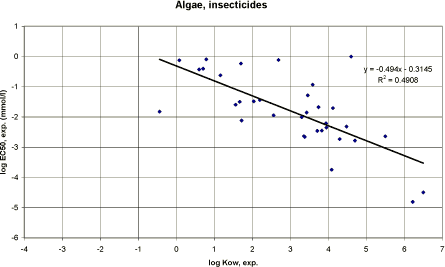
Figure 55
Correlation between log Kow and experimental EC50 for algae using data on insecticides (n=34).
Korrelation mellem log Kow og eksperimentel EC50 for alger ved anvendelse af insekticiddata (n=34).
The equations for acute algae toxicity using insecticide data were:
log EC50 (mg/l) = -0.4461 log Kow + 1.9898 |
(n=34, r² = 0.4395) |
log EC50 (mmol/l) = -0.494 log Kow - 0.3145 |
(n=34, r² = 0.4908) |
A tendency was observed and although the correlation coefficient was low the resulting regression equations may be used for indicative purposes. However, the number of data sets is small and more data
should be included before further recommendation can be considered.
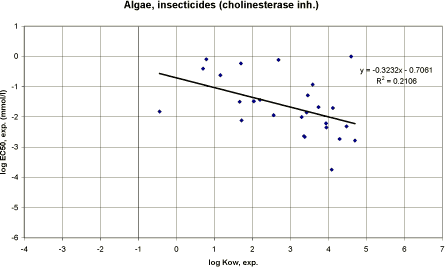
Figure 56
Correlation between log Kow and experimental EC50 for algae using data on insecticides with cholinesterase inhibition mode of action (n=26).
Korrelation mellem log Kow og eksperimentel EC50 for alger ved anvendelse af data for insekticider med cholinesterasehæmmende virkningsmekanisme (n=26).
The equations for acute algae toxicity using data on insecticides with a cholinesterase inhibition mode of action were:
log EC50 (mg/l) = -0.2843 log Kow + 1.6348 |
(n=26, r² = 0.1692) |
log EC50 (mmol/l) = -0.3232 log Kow - 0.7061 |
(n=26, r² = 0.2106) |
No result was gained by selecting data sets on insecticides with a cholinesterase inhibiting mode of action. It is not unexpected since the mode of action is irrelevant for algae.
Using data on plant growth regulators, a positive result would be expected. The equations for acute algae toxicity using plant growth regulator data were:
log EC50 (mg/l) = -0.2735 log Kow + 1.1986 |
(n=8, r² = 0.4655) |
log EC50 (mmol/l) = -0.3192 log Kow - 1.0879 |
(n=8, r² = 0.5149) |
A tendency was observed but the data too few to be conclusive. More data is needed before any recommendations can be made.
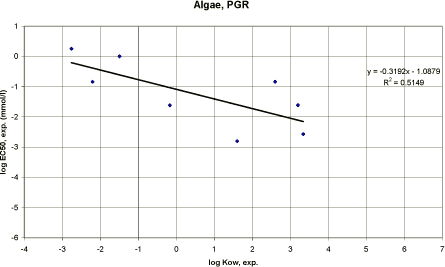
Figure 57
Correlation between log Kow and experimental EC50 for algae using data on plant growth regulators (n=8).
Korrelation mellem log Kow og eksperimentel EC50 for alger ved anvendelse af data for plantevækst regulatorer (n=8).
3.5.5 Discussion on estimated ecotoxicity
Based on the results using the available recommended QSARs it could be observed that the estimated acute toxicity was generally underestimated. Using the experimental results from pesticides, improved
results could be obtained for some organisms whereas for others (e.g. algae) no correlation could be observed using log Kow as descriptor. Dividing the data sets into primary functional areas (fungicides,
herbicides, insecticides, plant growth regulators and rodenticides) according to the assumption that the manufacturers recommended the substance for the use areas where the largest effects would be
expected, generally low correlations were observed. A further subdivision into the pesticides' mode of action resulted in improvements for some of the correlations and the opposite for others (cf. table 10).
It is generally agreed that when measuring the effect of a chemical it is at the molecular level that the chemical has an impact. Therefore the QSARs should be developed based on effect concentrations
expressed on a molar basis. Including the molecular weight in the calculations improved the correlations slightly (cf. table 10). However, for the pesticides where the variation in the molecular weight was
relatively small (mean molecular weight of the studied pesticides and standard deviation was 299 and 103, respectively) the improvements by using mmol/l instead of mg/l were low.
Table 10
Correlations between log Kow and experimental effect concentrations in mg/l or mmol/l
Korrelationer mellem log Kow og effektkoncentrationer i mg/l eller mmol/l
Test |
Group |
Mode of action |
Equation |
No. and correlation |
Fish acute |
Pesticides |
|
log EC50 (mg/l) = -0.4153 log Kow + 1.3643 |
(n=300, r² = 0.3197) |
log EC50 (mg/l) = -0.5076 log Kow + 1.6739 |
(n=296, r² = 0.3786) |
log EC50 (mmol/l) = 0.5471 log Kow - 0.6666 |
(n=296, r² = 0.4081) |
Fungicides |
|
log EC50 (mg/l) = -0.2963 log Kow + 0.8584 |
(n=59, r² = 0.1890) |
log EC50 (mmol/l) = -0.3146 log Kow - 1.5506 |
(n=59, r² = 0.2012) |
Herbicides |
All |
log EC50 (mg/l) = -0.5193 log Kow + 2.3548 |
(n=118, r² = 0.4814) |
log EC50 (mmol/l) = -0.5337 log Kow - 0.0459 |
(n=118, r² = 0.5030) |
Growth. inh |
log EC50 (mg/l) = -0.6344 log Kow + 2.3145 |
(n=69, r² = 0.5122) |
log EC50 (mmol/l) = -0.538 log Kow - 0.1293 |
(n=69, r² = 0.5223) |
Photosyn. inh. |
log EC50 (mg/l) = -0.4394 log Kow + 2.3336 |
(n=40, r² = 0.3913) |
log EC50 (mmol/l) = -0.4925 log Kow + 0.0717 |
(n=40, r² = 0.4692) |
Insecticides |
All |
log EC50 (mg/l) = -0.4998 log Kow + 0.8155 |
(n=81, r² = 0.3652) |
log EC50 (mmol/l) = -0.5471 log Kow - 1.5145 |
(n=81, r² = 0.3976) |
Cholinest. inh. |
log EC50 (mg/l) = -0.2903 log Kow + 0.4699 |
(n=53, r² = 0.1403) |
log EC50 (mmol/l) = -0.3334 log Kow - 1.8695 |
(n=53, r² = 0.1750) |
CSA |
log EC50 (mg/l) = -0.4515 log Kow - 0.111 |
(n=24, r² = 0.3690) |
log EC50 (mmol/l) = -0.4952 log Kow - 2.4772 |
(n=24, r² = 0.3840) |
PGR |
|
log EC50 (mg/l) = -0.3391 log Kow + 2.054 |
(n=14, r² = 0.6495) |
log EC50 (mmol/l) = -0.3925 log Kow - 0.2176 |
(n=14, r² = 0.6887) |
Rodenticides |
|
log EC50 (mg/l) = -0.5838 log Kow + 2.7154 |
(n=5, r² = 0.6390) |
log EC50 (mmol/l) = -0.617 log Kow + 0.2194 |
(n=5, r² = 0.6228) |
Daphnia Acute |
Pesticides |
|
log EC50 (mg/l) = - 0.5166 log Kow + 1.39 |
(n=255, r² = 0.2743) |
log EC50 (mmol/l) = - 0.5674 log Kow - 0.9079 |
(n=255, r² = 0.3127) |
Fungicides |
|
log EC50 (mg/l) = -0.3149 log Kow + 1.0021 |
(n=57, r² = 0.1518) |
log EC50 (mmol/l) = -0.4061 log Kow - 1.159 |
(n=57, r² = 0.2378) |
Herbicides |
|
log EC50 (mg/l) = -0.4388 log Kow + 2.2407 |
(n=97, r² = 0.3578) |
log EC50 (mmol/l) = -0.4515 log Kow - 0.1606 |
(n=97, r² = 0.3760) |
Growth inh. |
log EC50 (mg/l) = -0.4263 log Kow + 2.1565 |
(n=58, r² = 0.3344) |
log EC50 (mmol/l) = -0.4344 log Kow - 0.2771 |
(n=58, r² = 0.3547) |
Photosynt. inh. |
log EC50 (mg/l) = -0.5284 log Kow + 2.569 |
(n=39, r² = 0.4788) |
log EC50 (mg/l) = -0.5747 log Kow + 2.922 |
(n=39, r² = 0.5037) |
Insecticides |
|
log EC50 (mg/l) = -0.3521 log Kow - 0.6848 |
(n=79, r² = 0.2203) |
log EC50 (mmol/l) = -0.4049521 log Kow - 2.9818 |
(n=79, r² = 0.2626) |
Cholinest. inh. |
log EC50 (mg/l) = -0.2711 log Kow - 1.1177 |
(n=43, r² = 0.1243) |
log EC50 (mmol/l) = -0.3156 log Kow - 3.4422 |
(n=43, r² = 0.1582) |
CSA |
log EC50 (mg/l) = -0.5026 log Kow - 0.043 |
(n=26, r² = 0.3465) |
log EC50 (mmol/l) = -0.5478 log Kow - 2.395 |
(n=26, r² = 0.3741) |
PGR |
|
log EC50 (mg/l) = -0.0887 log Kow + 1.6166 |
(n=11, r² = 0.0364) |
log EC50 (mmol/l) = -0.133 log Kow - 0.6655 |
(n=11, r² = 0.0739) |
Daphnia Reprod. |
Pesticides |
|
log NOEC (mg/l) = -0.5052 log Kow + 0.1325 |
(n=96, r²= 0.3002) |
log NOEC (mmol/l) = -0.5402 log Kow - 2.2425 |
(n=96, r²= 0.3241) |
Fungicides |
|
log NOEC (mg/l) = -0.3035 log Kow - 0.5229 |
(n=28, r² = 0.1106) |
log NOEC (mmol/l) = -0.3067 log Kow - 3.0055 |
(n=28, r² = 0.1079) |
Herbicides |
|
log NOEC (mg/l) = -0.3163 log Kow + 0.7216 |
(n=37, r² = 0.2647) |
log NOEC (mmol/l) = -0.3319 log Kow - 1.6986 |
(n=37, r² = 0.2892) |
Insecticides |
|
log EC50 (mg/l) = -0.5516 log Kow - 1.425 |
(n=22, r² = 0.5682) |
log EC50 (mmol/l) = -0.6059 log Kow - 3.7308 |
(n=22, r² = 0.6198) |
Algae acute |
Pesticides |
|
log EC50 (mg/l) = - 0.2397 log Kow + 0.8195 |
(n=183, r² = 0.1190) |
log EC50 (mmol/l) = - 0.2679 log Kow - 1.5452 |
(n=183, r² = 0.1451) |
Fungicides |
All |
log EC50 (mg/l) = -0.2325 log Kow + 0.623 |
(n=48, r² = 0.1112) |
log EC50 (mmol/l) = -0.2427 log Kow - 1.8215 |
(n=48, r² = 0.1180) |
Ergosterol inh. |
log EC50 (mg/l) = -0.4549 log Kow + 1.4752 |
(n=17, r² = 0.0629) |
log EC50 (mmol/l) = -0.4415 log Kow - 1.0787 |
(n=17, r² = 0.0622) |
Growth inh. |
log EC50 (mg/l) = -0.2669 log Kow + 0.7178 |
(n=24, r² = 0.1605) |
log EC50 (mmol/l) = -0.2844 log Kow - 1.6727 |
(n=24, r² = 0.1770) |
Herbicides |
All |
log EC50 (mg/l) = -0.1898 log Kow +025345 |
(n=82, r² = 0.0636) |
log EC50 (mmol/l) = -0.2037 log Kow - 1.8623 |
(n=82, r² = 0.0752) |
Growth inh. |
log EC50 (mg/l) = -0.1768 log Kow + 0.9666 |
(n=51, r² = 0.0934) |
log EC50 (mmol/l) = -0.1884 log Kow - 1.456 |
(n=51, r² = 0.1049) |
Photosyn. inh. |
log EC50 (mg/l) = -0.0294 log Kow - 0.7492 |
(n=29, r² = 0.0008) |
log EC50 (mmol/l) = -0.0723 log Kow - 3.0378 |
(n=29, r² = 0.0052) |
Insecticides |
All |
log EC50 (mg/l) = -0.4461 log Kow + 1.9898 |
(n=34, r² = 0.4395) |
log EC50 (mmol/l) = -0.494 log Kow - 0.3145 |
(n=34, r² = 0.4908) |
Cholinest. inh. |
log EC50 (mg/l) = -0.2843 log Kow + 1.6348 |
(n=26, r² = 0.1692) |
log EC50 (mmol/l) = -0.3232 log Kow - 0.7061 |
(n=26, r² = 0.2106) |
PGR |
|
log EC50 (mg/l) = -0.2735 log Kow + 1.1986 |
(n=8, r² = 0.4655) |
log EC50 (mmol/l) = -0.3192 log Kow - 1.0879 |
(n=8, r² = 0.5149) |
PGR: Plant growth regulators. CSA: Contact and stomach action. Inh: Inhibitor
The problems in reaching an acceptable correlation may be multiple. The toxicity values are from several species of fish, but primarily the lowest value has been used unless Pimephales promelas or
Oncorhynchus my-kiss was present. Then they were preferred. The experimental Kow value may be incorrect if they are temperature or pH dependent. Generally, there is a large agreement with the
experimental and estimated log Kow. However, discrepancies did occur and especially for ionisable substances the deviation could be large. The result may be to perform several QSARs according to more
specific organism types, or according to chemical group e.g. from traditional chemical classification according to functional group. There is a high correlation between chemical functional groups and toxic
mode of action. Another explanation could be that there is no direct relationship between log Kow and toxicity for specific acting substances. The exposure time is also determining for the effect of
substances with a high log Kow.
Data reliability
To evaluate the predictive potential of QSARs, reliable biological and ecotoxicological databases must be assembled and computerised. These databases should be validated or reviewed as to data quality.
Currently, such works are performed e.g. by the US-EPA in the database AQUIRE and the chemical industry ECETOC (ECETOC 1993).
A procedure for data collection and interpretation for substances to be evaluated for classification as dangerous to the environment has been presented in Pedersen et al. (1995).
Biological results may be numerically imprecise and are generally not generated for use in QSAR prediction. For instance, results from limit tests or results above water solubility or just designated “more
than” cannot be used in QSARs. However, such data may be useful for demonstrating trends. But it must be kept in mind that individual results may not be quantitatively exact (Rispin 1981).
Isomers
Pesticides with one or more centres of asymmetry in the molecule represent a special problem in estimation of toxicity. For geometric isomers, the physico-chemical properties usually differ but optical
isomers have identical physico-chemical properties, at least in solution. Such isomers may nevertheless differ greatly in their biological activity (Ariëns 1984).
This makes ecotoxicity results based on racemic mixtures without know-ledge of the isomer ratio disputable, taking into account the fact that the individual isomers may differ essentially in their action.
4 Summary of conclusions
Traditional QSAR models are based on a few key physico-chemical data (typically melting point, octanol/water partition coefficient) which are then used to calculate other physico-chemical parameters if
they are missing. The calculation equations should be based on experimental laboratory studies performed by accepted guidelines and the results based on regression analyses.
The conclusions of this project comparing experimental values with QSAR model estimations are summarised below. The main object was to study whether the currently recommended general QSARs
could be used to perform acceptable estimates on pesticides physico-chemical properties and aquatic toxicity. The study was expanded by examining whether general or specific regression equations could
be derived for log Kow and pesticides after subdivision into fungicides, herbicides, insecticides, plant growth regulators and rodenticides. No attempt has been made to improve on regression equations by
removing outliers.
Melting point
The correlation between experimental and estimated melting points was low. The used method generally overestimated the melting point values. Thus, the currently best available method by Meylan and
Howard (1994) is not recommendable for pesticides in its present form.
Water solubility
The estimations of water solubility were acceptable using the selected computerised QSAR model although the correlation coefficient for pesticides was smaller than for the data used to develop the model.
A linear regression analysis based on log water solubility and log Kow of the pesticides used in this report resulted in a QSAR model with a slightly reduced correlation coefficient.
Vapour pressure
The presented QSAR model on vapour pressure was not able to perform a sufficient close correlation of experimental and estimated vapour pressures from the included pesticide data set. However, it
should be noted that the experimental values were based on test results varying between 20°C and 25°C whereas the vapour pressures were estimated at 25°C.
Henry's Law constant
For Henry's Law constant, no conclusion can be drawn from the used pesticide data set since values estimated by calculation and by computer model may not be correct for the pesticide in question.
However, it can be concluded that the two estimation methods are presenting comparable results.
Log Kow
The octanol/water partition coefficient log Kow could be reasonably well estimated from the QSAR models based on structure fragment analysis. The method is complicated and recommended to be
performed by available computer models. However, an alternative QSAR model may be used based on water solubility. For the pesticides used in this report, it resulted in a lower correlation coefficient but
both methods should be sufficient in a screening procedure.
Adsorption
The soil adsorption coefficient factor Koc is very important in the evaluation of the mobility of pesticides. Since the known methods for estimation are approximate at best, measured values should be used if
they are available. If however, measured data on Koc for some reason are not present, the PCKOC programme (Meylan and Howard 1994) or the QSAR model to estimate Koc developed by Sabljic et
al. (1995) and recommended in TGD (1996) can be used. Based on 338 pesticides in this project, a QSAR model was derived which had a correlation coefficient close to the model by Sabljic et al.
(1995). For comparison of the outcome of each QSAR estimation on individual pesticides the results are presented in the appendix.
Ecotoxicity
It is important to realise that most of the QSARs developed and the QSARs presented above are based on chemicals that are biased toward industrial organic chemicals that are not overtly designed to have
biological activity. Pesticides are mostly reacting in a specific mode of action and no QSARs have been recommended for substances that act by more specific modes of action (TGD 1996).
The situation with the present data set is that it has been chosen as a mixture of different pesticides to evaluate whether the evolved QSARs could be used in general. If another choice had been made, e.g.
the used pesticides were selected according to function, mode of action or chemical class, another result may have appeared. However, the chosen pesticides included in this report represent too many
chemical classes to collect a sufficient number in each class for analysis. On the other hand, the results demonstrate that the available QSAR models should be used with great precaution, as they are not
developed specifically for pesticides.
QSARs have been developed with acceptable results for most industrial chemicals. The recommended QSARs on ecotoxicity were not recommendable for pesticides in general. However, the results cannot
be used without further development for chemicals like pesticides with specific biological mode of action.
The study demonstrates that, by dividing the pesticides according to their mode of action, improved correlations could be found for some organisms and some modes of action. The problems in reaching an
acceptable QSAR for estimating ecotoxicity may be multiple (cf. discussion in section 3.5.5).
Another explanation for the result could be that there is no direct relationship between log Kow and toxicity for specific acting substances.
The conclusion is that no QSARs of a sufficient and reliable quality are currently available for estimating ecotoxicity of pesticides. The values can only be acquired from regular studies.
The general idea is not that QSARs shall replace experimentally derived data for pesticides. It must be kept in the mind that the purpose of using QSAR models is to give a qualified estimate of an endpoint in
replacement of a missing experimental data.
However, using the QSAR models with precaution and keeping in mind their background presents reasonable good estimates to perform preliminary assessments on physico-chemical properties and in very
precautions way of degradation products and /or metabolites. As demonstrated by the studies by e.g. Nendza et al. (1991) and Vighi et al. (1991), positive results can be obtained but they must await a
thorough evaluation before they can be recommended for pesticides in general or for specified groups.
Relating to degradation products and transformation products QSARs may be useful in the priority of toxicity studies and which endpoint should be studied.
QSARs have been found to have great predictive values for chemicals of less complicated structures or other modes of reaction than pesticides and the use is recommended in the TGD (1996) as a useful
part of the risk assessment procedure. The area of developing QSAR models for more complicated structures and chemicals with specific modes of action is therefore currently an area of high priority.
References
Ariëns EJ (1984): Domestication of chemistry by design of safer chemicals: Structure-activity relationships.
AQUIRE: Aquatic Toxicity Information Retrieval. US-EPA Environmental Protection Laboratory Duluth, Minnesota and Montana State University, Bozemann, Montana
Bahnick DA and Douchette (1988): Use of molecular connectivity indices to estimate soil sorption coefficients for organic chemicals. Chemosphere 17: 1703-1715.
Briggs GG (1969): Molecular structure of herbicides and their sorption by soils. Nature 223: 1288.
Briggs GG (1973): A simple relationship between soil adsorption of organic chemicals and their octanol/water partition coefficients. Proc. 7th British Insecticide and Fungicide Conf. Boots Co, Nottingham
UK.
Chapman NB, Shorter J (eds.) (1978): Correlation analysis in chemistry: Recent advances. Plenum Press, New York.
Clausen H (1998): Excel-database Tox-sum². Database elaborated during the preparation of technical report 223, 1998 at the Danish Environmental Research Institute (DMU).
Commission Directive 87/302/EEC adapting to technical progress for the ninth time Council Directive 67/548/EEC on the approximation of laws, regulations and administrative provisions relating to the
classification, packaging and labelling of dangerous substances. O. J. L 133, 30.5.1988.
Commission Directive 92/69/EEC adapting to technical progress for the seventeenth time Council Directive 67/548/EEC on the approximation of laws, regulations and administrative provisions relating to the
classification, packaging and labelling of dangerous substances. O. J. L 383, 29.12.1992.
Connell DW (1988): Bioaccumulation behaviour of persistent organic chemicals with aquatic organisms. Rev. Environ. Contam. Toxicol. 101: 117-154.
Connell DW, Markwell RD (1990): Bioaccumulation in the soil to earthworm system. Chemosphere 20: 91-100.
Dagani R (1981): Toxicologists seek structure-activity links. Chemical & Engineering News march 9, 1981: 26-29
Dearden JC (1991): Physico-chemical descriptors. In: Karcher and Devillers (eds) Practical application of quantitative structure-activity relationships (QSAR) in environmental chemistry and toxicology.
Chemical and Environmental Science Series Vol.1. Kluwer Acad. Publ. Dordrecht, the Netherlands.
ECDIN (1993): Environmental Chemicals Data and Information Network. Commission of the EC, JRC, Environmental Institute. Ispra, Italy.
ECETOC (1993): Aquatic toxicity data evaluation. Technical Report No. 56. (Appendix C: The database. In a separate document). European Centre for Ecotoxicology and Toxicology of Chemicals.
Brussels, Belgium.
EU (1992): Annex to Commission Directive 92/69/EEC of 31 July 1992 adapting to technical progress for the seventeenth time Council Directive 67/548/EEC on the approximation of laws, regulations and
administrative provisions relating to the classification, packaging and labelling of dangerous substances. Official Journal of the European Communities L 383 A Vol. 35, 29 Dec. 1992.
Hall LH, Kier LB (1981): The relation of molecular connectivity to molecular volume and biological activity. Eur. J. Med. Chem. 16: 399-407.
Hamaker JW (1975): Interpretation of soil leaching experiments. In: Haque R and Freed VH (eds.): Environmental dynamics of pesticides. Plenum Press NY.
Hance RJ (1967): Relationship between partition data and the adsorption of some herbicides by soils. Nature 214: 630-31.
Hansch C, Leo AJ (1979): Substituent constants for correlation analysis in chemistry and biology. Wiley, New York.
Hart LW (1991): The use of data estimation methods by regulatory authorities. Sci. Total Environ. 109/110: 629-633.
Hermens JLM (1989): Quantitative structure-activity relationships of environmental pollutants. In: Hutzinger O (ed.): Handbook of Environmental Chemistry, p 111-162. Springer Verlag, Berlin.
Hine J, Mookerjee PK (1975): The intrinsic hydrophilic character of organic compounds. Correlations in terms of structural contributions. J. Org. Chem. 40: 292-298.
Isnard P, Lambert S (1989): Aqueous solubility and n-octanol/water partition coefficient correlations. Chemosphere 18(9/10): 1837-1853.
Kanazawa J (1989): Relationship between the soil sorption constants for pesticides and their physicochemical properties. Environ. Toxicol. Chem. 8: 477-484.
Karcher and Devillers (eds) (1990): Practical application of quantitative structure-activity relationships (QSAR) in environmental chemistry and toxicology. Chemical and Environmental Science Series Vol.1.
Kluwer Acad. Publ. Dordrecht, the Netherlands.
Karcher W and Devillers J (1990): SAR and QSAR in environmental chemistry and toxicology: Scientific tool or wishful thinking. In: Karcher and Devillers (eds) Practical application of quantitative
structure-activity relationships (QSAR) in environmental chemistry and toxicology. Chemical and Environmental Science Series Vol.1. Kluwer Acad. Publ. Dordrecht, the Netherlands.
Kenaga EE (1980): Correlation of bioconcentration factors of chemicals in aquatic and terrestrial organisms with their physical and chemical properties. Environ. Sci. Technol. 14(5):553-556.
Könemann WH (1981): Quantitative structure-activity relationships in fish toxicity studies. Part 1: Relationships for 50 industrial pollutants. Toxicology 19: 209-221.
Lagersted A (1987): QSAR og toksikologi - en ny strategi i kemikalievurderingen. QSAR and toxicology - a New Strategy in the Evaluation of Chemicals. Environmental Project no 82. Danish EPA 1987.
Lambert SM (1968): Omega (), a useful index of soil sorption equilibria. J. Agric. Food Chem. 16: 340-343.
Linders JBHJ, Jansma JW, Mensink BJWE, Otermann K, (1994): Pesticides: Benefaction or Pandoras box? A synopsis of the environmental aspects of 243 pesticides. Report No 679101014. National
Institute of Public Health and Environmental Protection, Bilthoven, The Netherlands.
Lyman WJ (1985): Environmental exposure from chemicals. In: Neely WB, Blau GE (eds): Environmental exposure from chemicals. Vol.1. CRC Press, Boca Raton.
Lyman WJ, Reehl WF, Rosenblatt DH (1982, 1990) Handbook of chemical property estimation methods. American Chemical Society, Washington DC (1982 ed. reprinted in 1990).
McCarthy LS, MacKay D (1993): Enhancing ecotoxicological modelling and assessment. Environ. Sci. Technol. 27: 1719-1728.
Meylan WM, Howard PH (1991): Bond contribution method for estimating Henry's Law constants. Environ. Toxicol. Chem. 10: 1283-1293.
Meylan WM, Howard PH, Boethling (1992): Molecular topology/fragment contribution method for predicting soil sorption coefficients. Environ. Sci. Technol. 26: 1560-1567.
Meylan WM, Howard P (1994): EPIWIN - Estimation Program Interface for Microsoft Windows 3.1. Syracuse Research Corporation, Syracuse.
Meylan WM, Howard PH (1995): Atom/fragment contribution method for estimating octanol-water partition coefficients. J. Pharm. Sci. 84: 83-92.
Nendza M (1991): Predictive QSAR models estimating ecotoxic hazard of phenylureas: Aquatic toxicity. Chemosphere 23: 497-506.
Nendza M (1991b): QSARs of bioconcentration: validity assessment of log Pow/log BCF correlations. In: Nagel R, Loskill R (eds.): Bioaccumulation in aquatic systems. VCH Weinheim.
Nendza M, Dittrich B, Wenzel A, Klein W (1991): Predictive QSAR models for estimating ecotoxic hazard of plant protecting agents: target and non-target. Sci. Total Environ. 109/110: 527-535.
Nirmalakhandan NN, Speece RE (1988): Prediction of aqueous solubility of organic chemicals based on molecular structure. Environ. Sci. Technol. 22: 328-338.
OECD (1993): OECD guidelines for the testing of chemicals. Vol. I-II. The Organisation for Economic Co-operation and Development, Paris.
OECD (1993b): Application of structure-activity relationships to the estimation of properties important in exposure assessment. OECD Environment Monographs No. 67. The Organisation for Economic
Co-operation and Development, Paris.
Pedersen F, Falck J (1997): Environmental hazard classification: Classification of selected substances as dangerous for the environment (II). TemaNord 1997:549, Nordic Council of Ministers,
Copenhagen..
Pedersen F, Tyle H, Niemelä JR, Guttmann B, Lander L, Wedebrand A (1995): Environmental hazard classification - data collection and interpretation guide (2nd edition). TemaNord 1995: 581. Nordic
Council of Ministers, Copenhagen.
Ribo JM (1988): The octanol/water partition coefficient of the herbicide chlorsulfuron as a function of pH. Chemosphere 17: 709-715.
Rispin A (1981): Introduction to symposium on the development and use of reliable data bases for quantitative structure-activity relationships. J. Chem. Inf. Comput. Sci 21: 1-2.
Russom CL (1991): ASTER. Assessment tools for the evaluation of risk. A user guide. US Environmental Protection Agency. Environmental Research Laboratory. Duluth, Minnesota.
Russom CL, Bradbury SP, Broderius SJ, Hammermeister DE, Drummond A (1997): Predicting modes of toxic action from chemical structure: Acute toxicity in the Fathead minnow (Pimephales
promelas). Environ. Toxicol. Chem. 16(5): 948-967.
Sabljic A (1987): On the prediction of soil sorption coefficients of organic pollutants by molecular structure: Application of molecular topo-logy model. Environ. Sci. Technol. 21: 358-366.
Sabljic A, Güsten H, Verhaar H, Hermens J (1995): QSAR modelling of soil sorption. Improvements and systematics of log Koc vs. log Kow correlations. Chemosphere 31(11/12): 4489-4515.
Stein SE, Brown RL (1994): Estimation of normal boiling points from group contributions. J. Chem. Inf. Comput. Sci. 34: 581-587.
Stevenson FM (1976): Organic matter reactions involving pesticides in soil. In: Kaufman DD, Still GG, Paulson GD, Blandal SK (eds.): Bound and conjugated pesticide residues. ACS Symposium. Ser. 29,
American Chemical Society, Washington.
TGD (1996): Technical guidance document in support of Commission Directive 93/67/EEC on risk assessment for new notified substances and Commission Regulation (EC) No. 1488/94 on risk
assessment for existing substances. Part III. European Commission, Brussels.
Tomlin C (Ed.) (1994): The Pesticide Manual. Incorporating The Agrochemical Handbook. Tenth Ed. The British Crop Protection Council and The Royal Society of Chemistry. United Kingdom.
Tomlin CDS (Ed.) (1997): The Pesticide Manual.. Eleventh Ed. The British Crop Protection Council. United Kingdom.
US-EPA (1994): ECOSAR ver 1.0. A computer program for estimating the ecotoxicity of industrial chemicals based on structure activity relationships. US Environmental Protection Agency, Office of
Pollution Prevention and Toxics. Report No. 748-R-93-002.
Van Gestel CAM, Ma WA (1988): Toxicity and bioaccumulation of chlorophenols in earthworms in relation to bioavailability in soil. Ecotox. Environ. Safety 15: 289-297.
van Straalen NM, Denneman CAJ (1989): Ecotoxicological evaluation of soil quality criteria. Ecotoxicol. Environ. Safety 18: 241-251
Veith GD, Defoe DL, Bergstedt BV (1979): Measuring and estimating the bioconcentration factor of chemicals in fish. J. Fish. Board Can. 36: 1040-1048.
Veith GD, Call DJ, Brooke LT (1983): Structure-toxicity relationships for the fathead minnow, Pimephales promelas: narcotic industrial chemicals. Can. J. Fish. Aquat. Sci. 40: 15-29.
Verhaar HJM, Van Leeuwen CJ, Hermens JLM (1992): Classifying environmental pollutants. I: Structure-activity relationships for prediction of aquatic toxicity. Chemosphere 25: 471-491.
Verhaar HJM, Mulder W, Hermens JLM (1995): QSARs for ecotoxicity. In: Hermens JLM (ed.): Overview of structure-activity relationships for environmental endpoints, Part 1: General outline and
procedure. Report prepared within the framework of the project “QSAR for prediction of fate and effects of chemicals in the Environment”, an international pro-ject of the Environmental Technologies RTD
Programme (DGXII/D-1) of the European Commission under contract no. EV5V-CT92-0211.
Vighi M, Garlanda MM, Calamari D (1991): QSARs for toxicity of organophosphorous pesticides to Daphnia and honeybees. (Sci. Total Environ. 109/110: 605-622.
Wagner C, Løkke H (1991): Estimations of ecotoxicological protection levels from NOEC toxicity data. Water Res. 24: 1237-1242.
Wakita K, Yoshimoto M, Miyamoto S, Watanabe H (1986): A method for calculation of the aqueous solubility of organic compounds by using new fragment solubility constants. Chem. Pharm. Bull. 34:
4663-81.
Weininger D (1988): SMILES, a chemical language and information system. 1. Introduction to methodology and encoding rules. J. Chem. Inf. Comput. Sci. 28: 31-36
Yalkowsky SH, Banerjee S (1992); Aqueous solubility methods of estimation for organic compounds. Marcel Dekker, Inc. NY.
Appendix A
Appendix A
A list of the abbreviations used in the appendix is located after the tables.
Table 1
Pestcide ISO name, chemical class, use, mode of action, CAS No., molecular formula and molecular weight (MW).
Pesticid ISO navn, kemisk klasse, anvendelse, virkningsmekanisme, CAS nr., molekylformel og molekylvægt (MW).
Click here to see Table 1.
Table 2
Melting point (MP), boiling point (BP), water solubility (S), vapour pressure (VP) and Henry's Law constant.
Smeltepunkt (MP), kogepunkt (BP), vandopløselighed (S), damptryk (VP) og Henrys Lov Konstant.
Click here to see Table 2.
Table 3
Kow, log Kow, adsorption coefficient (Kd and Koc ranges and the log Koc used in the report) and Koc estimated by Lyman, TGD (non-hydrophobis and hydrophobics) and this study (OCH).
Kow, log Kow, adsorption koefficienter (kd og Koc variationsbredder samt log Koc anvendt i rapporten) samt log Koc estimeret efter QSARs opstillet af Lyman, TGD og denne rapport (OCH).
Click here to see Table 3.
Table 4
Bioaccumulation factor (BCF) and acute effect concentrations for fish, daphnia and algae. The experimental acute effect levels are the lowest observed in the accepted literature.
Bioakkumuleringsfaktor (BCF) og akutte effektkoncentrationer for fisk, dafnier og alger. Den eksperimentelle effektkoncentration angivet er den laveste, der blev fundet i den anvendte
litteratur.
Click here to see Table 4.
List of abbreviations used in the tables 1 to 4.
A |
Acaricide |
B |
Bactericide |
CA |
Contact action |
Cell div. inh |
Cell division inhibitor |
CSA |
Contact and stomach action |
CSRA |
Contact, stomach and respiration action |
dev. |
Development |
Exp. |
Experimental value |
Est. |
Estimated value |
F |
Fungicide |
H |
Herbicide |
hf |
QSAR on hydrophobic substances |
I |
Insecticide |
inh. |
inhibitor |
I rep |
Insect repellent |
Lyman |
cf. reference Lyman et al. 1982 |
MW |
Molecular weight |
N |
Nematicide |
nhf |
QSAR on non-hydrophobic substances |
np |
non-polar |
PGR |
Plant growth regulator |
pol |
polar |
Prot.synt.inh |
Protein syntesis inhibitor |
R |
Rodenticide |
SA |
Systemic action |
Synt. |
Synthesis |
Syst. |
Systemic |
TGD |
cf. reference TGD 1996 |
Vit. K inh. |
Vitamin K inhibitor (anticoagulant) |
| Top | | Front page |
Version 1.0 November 2004, © Danish Environmental Protection Agency
|