| Front page | | Contents | | Previous | | Next |
Market information in life cycle assessment
6 Forecasting future processes
6.1 Procedure
6.1.1 Step 5: Consistency check
6.2 Determining the parts of the product systems for which forecasting is relevant
6.2.1 Time horizon of study
6.2.2 Position in the life cycle
6.3 Determining the necessary detail of forecasting
6.4 Choosing the relevant forecasting method
6.5 Forecasting by extrapolation
6.6 Forecasting by modelling
6.7 Participatory forecasting
6.8 Exploratory and normative forecasting
6.9 Scenario forecasting
6.10 Consistency check
6.11 Combining different forecasting methods
Forecasting is the activity of producing a forecast. A forecast is a statement about the future. Forecasting is done in almost all aspects of life and at a number of different levels. Weather forecasting, forecasting of sales curves and technology forecasting are commonly used.
In the previous chapters, reference was made to the time horizon of the studied change, and it was made clear that the processes to include in the studied product systems may change over time, depending on the future market situation. The topic of this chapter is the actual forecasting procedures to be applied.
The alternative to forecasting is the use of unjustified assumptions about the future or the use of data for the current situation as proxies for data for the future situation. While this may be adequate in some situations (and especially in the first iteration of a life cycle assessment) and for some parts of the product systems, the use of forecasting is often necessary to ensure adequate validity of the data used and the conclusions drawn. The purpose of this chapter is to show the relevance of forecasting and to recommend a procedure to improve the consistency and transparency of the forecasting.
Forecasting product systems includes both:
- The forecasting of the future market situations to be used in the procedures given in chapters 3 and 4, to allow the identification of the relevant processes to include in the product systems, i.e. forecasting of:
- Obligatory product properties
- Geographical and temporal market boundaries
- Market ties between specific suppliers and customers
- Market trends
- Production constraints
- Relative production costs etc., for each possible supplier/technology
- The forecasting of the technologies of the specific processes identified as relevant
- The forecasting of the exchanges of the specific processes identified as relevant
6.1 Procedure
The procedure consists of five steps (illustrated in the flowchart in figure 6.1):
- Determining the parts of the product systems to be forecast
- Determining the necessary detail of forecasting
- Choosing the relevant forecasting methods
- Forecasting
- Consistency check
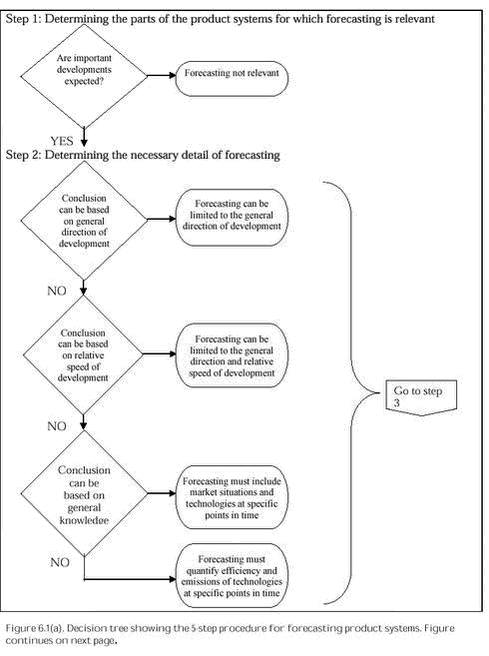 Click on the picture to see the html-version of: Figure 6.1(a)
6.1.1 Step 5: Consistency check
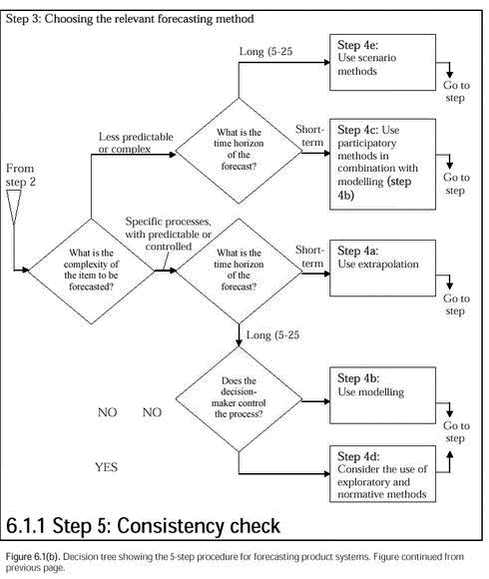
Click on the picture to see the html-version of: Figure 6.1(b)
6.2 Determining the parts of the product systems for which forecasting is relevant
It may not be equally important to forecast all parts of the product systems. There may even be entire life cycle assessments where forecasting is not necessary. The factors that need to be considered are:
- The general speed of development of the relevant markets, technologies and exchanges
- Expectations about radical or untypical developments
- The time horizon of the study relative to the expected development
- The position of the specific process in the life cycle of the product
Speed of development Markets generally develop more slowly as they mature. With time, the product becomes more well-defined (the obligatory product properties tending to become more encompassing), and the market boundaries and production constraints less volatile (tending to be determined more by natural geography, such as climate and natural transport barriers, than by administrative differences). Likewise, the production costs and technologies develop more slowly as the ultimate physical constraints of each material, process or technology is approached. There have been several attempts at classifying different industrial sectors according to their speed of development, but none of them are fully satisfactory. An example is given in table 6.1. For a given technology, the size of most process exchanges will decrease over time, following the general efficiency development in the corresponding technology, but for exchanges that are in focus because of their economic value or their known environmental impacts, the speed of development may be above average (example: the phasing out of CFC’s).
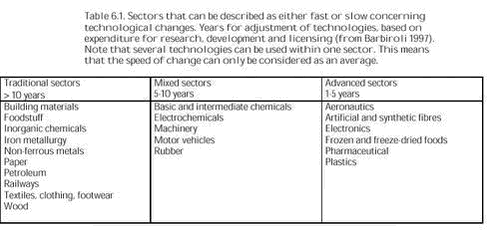 Click on the picture to see the html-version of: Table 6.1
Radical or untypical developments The general considerations in the preceding paragraph may be overruled by specific knowledge in specific situations. Even traditional sectors may be subject to sudden, radical changes determined by larger shifts in other sectors or in general technological developments or socio-economic conditions. For example, over the last decade development in the vehicle sector has been speeded up by pre-announced regulation on emissions. Another example is the introduction of genetic engineering, which may cause sudden, radical changes to the otherwise technologically mature food sector.
6.2.1 Time horizon of study
The general need for forecasting depends on the relation between the time horizon of the study and the general speed of development, taking into account also any untypical developments. The time horizon of the study is determined by the period for which the conclusions of the life cycle study should be valid plus the life-time of the affected capital investments. This period is typically considerably longer than the lifetime of the product. The period for which the conclusions should be valid is related to the application area of the study (cf. figure 1.1). Forecasting is typically relevant if the time horizon of the study is longer than 5 years. In addition, forecasting is relevant in sectors with rapid development or if radical or untypical developments can be expected.
6.2.2 Position in the life cycle
Even when the time horizon of the study is longer than 5 years, not all processes in the life cycle may be affected so far into the future that forecasting becomes relevant.
6.3 Determining the necessary detail of forecasting
As any other aspect of life cycle assessment, forecasting may be made in more or less detail. Covering the most important processes in the studied life cycles, a forecast may include (in order of increasing detail):
- the general direction of the development, in terms of technology and exchanges,
- the relative speed of development of the relevant processes,
- the situation at specific points in time, corresponding to the time horizon of the study,
- the specific technology and its exchanges at such specific points in time.
If the general direction of development confirms or enhances the current situation, this qualitative information may be adequate as an addition to a life cycle study based on current or historical data. For example, to conclude that an alternative energy source that is currently competitive versus fossil fuels will continue to be competitive, it is adequate to have the general knowledge that costs of fossil fuel resources will be slowly increasing on the long term (as reserves are depleted) and that costs of the alternative energy source will continue to fall (following an ordinary learning curve).
The relative speed of development of different processes must be taken into account if the direction of development does not in itself provide a clear indication, and if the speed of development is not uniform for all the involved processes. This information, which is still qualitative, may sometimes be adequate basis for a conclusion. For example, when the price of all fossil fuels are expected to increase in the long run, it is necessary to know the relative speed of price developments for coal, oil, and natural gas, in order to determine which fossil fuel will be the most competitive in the future.
Combining knowledge on the direction and speed of development with more quantitative information allows forecasts of the market situation and the technologies involved at specific points in time. For example, information on the current costs of coal and wind power and the actual speed of cost developments for these two technologies (e.g. expressed in average percentage change in raw material costs and efficiency per year and/or as a coefficient of a learning curve) will allow to forecast whether wind power or coal power is the most competitive at a specific point in time.
If necessary, the relevant technologies may then be further quantified, also in terms of exchanges, by combining specific technical information with general forecasts on technical efficiency and emission control.
An example of a forecast combining knowledge on the direction and speed of development can be found in Prognos (1999).
6.4 Choosing the relevant forecasting method
Several methods can be used for forecasting, some of which are more commonly used for specific applications. The most extensive description of methods is by the UN millennium project (Glenn 1994a, 1999). Other reviews of methodology are made by Bell (1997), Donnelly (1997), Vanston (1995), and Martino (1972).
The number of described methods varies between the different reviews. Also, terminology is variable and overlaps occur. Strict definitions of the specific methods are generally lacking.
The taxonomy suggested by the UN millennium project (Gordon 1994a, 1999) distinguish the methods as either:
- normative or exploratory, and
- quantitative or qualitative.
However, the authors describe themselves the shortcomings of this taxonomy: The normative/exploratory dimension relates more to the application of the methods than to the methods themselves. Many methods are used for both normative and exploratory forecasting. As for the quantitative/qualitative distinction, it is argued that even quantitative methods use qualitative assumptions and a qualitative method can use numbers (Glenn 1994b).
Other taxonomies have been suggested by Vanston (1995), dividing according to different views of the future (extrapolators, pattern analysts, goal analysts, counter punchers and intuitors), and Michael Marien (cited in Glenn 1994b) using a division according to 7 P‘s (probable, possible, preferable, present, past, panoramic and participatory).
We have found it most useful to divide the methods into 6 groups:
- Extrapolation, - Modelling, - Exploratory, - Scenario, - Participatory, - Normative,
which we describe shortly below. The forecasting method to apply in a specific situation depends on the time horizon of the forecast and the predictability and complexity of the item to be forecast (see table 6.2). The choice of method does not depend on the required detail.
Extrapolation is based on extending historical and current trends into the future. It is based on a belief that the future represents a logical extension of the past and that information contained in historical data can be extracted, analysed, and reduced to one or more equations that can be used to predict future events. This may be adequate for short-to-medium term forecasts of specific processes, when no radical or untypical developments are expected. A forecast based on extrapolation may also be used as a surprise-free base-line forecast ("suppose things keep going as they have in the past ...") for the modifications of other methods. Trend analysis, time series, regression, econometrics, and simulation modelling belong in this group (Futures Group 1994a).
Modelling seeks to identify the determining mechanisms and to model how the combined effects of several mechanisms will influence the future. It is based on a belief that future events will be influenced by mechanisms analogous to those determining past events. Thus the best way to describe the future is by identifying the determining mechanisms and to model how these will influence the future. In this way, probabilities rather than possibilities are considered. Examples of methods for identifying determining mechanisms and probabilities are analogy analysis, technological sequence analysis, stakeholder analysis (Vanston 1995), and structural analysis. In trend impact analysis, surprise-free forecasts are adjusted to accommodate the expected impact of determining mechanisms (Gordon 1994b). Using cross-impact analysis (Gordon 1994c), probabilistic systems dynamics (Monte Carlo models), engineering-economic models and equilibrium models, the combined effects of several trends can be studied.
Participatory methods seek the insight and opinions of experts and stakeholders. They are based on the belief the future is shaped by complex mixture of trends, random events and actions of individuals and institutions. Therefore, to forecast the future, the insight and opinions of experts and stakeholders are seen as more useful than rational methods. The results of these methods are often more normative (what the future should be) than analytic (what the future is likely to be). However, analytical and modelling methods may provide input to guide participatory methods and the results from participatory methods may be used as inputs in modelling methods. Participatory methods are mainly relevant in complex situations, whereas in simple situations with a high degree of control, stakeholder involvement may be an unnecessary complication. Participatory methods range from the more structured Delphi technique (Gordon 1994d), scanning (Gordon & Glenn 1994), focus groups, charrette, Syncon, and future search conferences (Glenn 1994d) to the less structured methods relying more on subjective judgement, such as genius forecasting, intuition and visioning (Glenn 1994e).
Exploratory methods seek to structure all possible futures by combining analytic techniques, which give an exhaustive qualitative description of the field, with imaginative techniques aimed at filling all gaps in the analytical structure. In this way, possibilities rather than probabilities are considered. This may be useful in product development, for those processes upon which the decision-maker has a large potential influence. Morphological analysis, relevance trees, mind mapping and future wheel belong to this category (Futures Group 1994b, Glenn 1994c).
Normative (or goal-oriented) forecasting begins with stating the desired future and then moves backwards in time to identify the necessary steps for reaching this goal (Coates 1994). Besides this particularity, any of the above mentioned methods might be applied also in normative forecasting. Like exploratory methods, normative forecasting may be useful in product development, for those processes upon which the decision-maker has a large potential influence. Backcasting is an important member of this category.
Scenario methods include and combine aspects of the other methods, especially participatory, modelling and exploratory methods, with the aim of creating several distinct scenarios. They are based on the belief that the future is essentially unpredictable and largely random. Considering the uncertainties, modelling will not lead to one future, but rather to many different futures, each of which may be described in the form of a scenario (Futures Group 1994c).
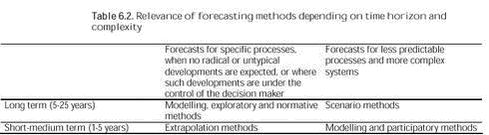
Click on the picture to see the html-version of: Table 6.2
The divisions in table 6.2 should be seen as guiding only. In practice, the distinction between the different situations and relevant methods is not sharp, and more than one method may be relevant in a specific situation. Often, different methods can be combined to give a more reliable forecast (see section 6.2).
6.5 Forecasting by extrapolation
Extrapolation is the simple (linear or non-linear) prolongation into the future of historical relations.
While all time series of data may be extrapolated, it is not all data that it is meaningful to extrapolate. To improve the reliability:
- The extrapolation should preferably be based on the determining factor for the expected development and the trend of this factor.
- Data for an extrapolation should at least go five years back, preferably 10 years. However, when radical changes have taken place, which have altered or radically influenced the determining factors, it does not make sense to include data from before such changes.
- Any constraints on the extrapolation should be taken into account (e.g. physical or political boundaries for the development of the extrapolated factor). When approaching a constraint, the extrapolation will no longer be a good approximation.
Some general conclusions from empirical observations may be applied:
- The introduction of a new technology tends to follow an S-curve, so that the initial penetration is slow but with a logarithmic increase, followed by a linear growth again followed by a logarithmic decrease in cumulative penetration until the market is saturated.
- Production costs tend to decrease with cumulative production capacity, following a so-called learning curve, a logarithmic curve typically described by a learning factor, which is the cost reduction achievable by doubling the cumulative production. The learning factor, which tends to be fairly stable for each specific technology, is typically between 0.9 and 0.75, meaning a cost reduction of 10-25% when doubling cumulative production. More innovative technologies tend to have the lowest learning factors (largest cost reductions) compared to more established technologies, which also implies that the learning factor does change when seen over very long time horizons. The learning curves for cost reductions mainly reflect savings in manpower, but physical efficiency improvements also play a role. Karvonen (2000) show a good correlation between gross emissions and cumulative investment in the Finnish pulp and paper industry, and Pento & Karvonen (2000) show that emission coefficients are even closer related to cumulative investments. Therefore, conservative learning factors (i.e. 0.85 - 0.95) may be used as proxies when estimating the physical flows in a life cycle study when the physical efficiency improvements in these flows are not known from other sources. Also in energy models, learning curves have recently gained more widespread use (Mattsson 1997, Mattsson & Wene 1997, IEA 2000a, Pehnt 2001)
Sources of time series may be:
- Technical literature and technical experts on the process in question.
- Statistics of industrial associations.
- General statistical publications.
Kakudate et al. (2000) provide an LCA-relevant example of extrapolation of copper contamination of steel based on steel production statistics, the lifetime of steel products, and national recycling, import, and export rates.
An extrapolation is not necessarily quantitative, but can be e.g. a text description of the consequences of extending the prevailing trends into the future.
Limitations of extrapolation in forecasting Since extrapolation is based on historical data alone, and does not include combined effects of several developments, it can only be used for medium or short-term forecasts for smaller, specific areas, where no radical or untypical developments are expected.
In spite of its limitations, an extrapolation is a better forecast than an assumption of status-quo. Thus, even when there is no time or resources to involve technical experts, it may be justified that a non-expert makes a simple extrapolation as a first approximation.
6.6 Forecasting by modelling
Modelling is the analysis of the interactions of several cause-effect mechanisms over time, depending on their relative strengths and probabilities of occurrence.
Modelling is based on an identification of relevant mechanisms, their probabilities of occurrence, and their interactions. In this way, otherwise surprise-free forecasts are adjusted to accommodate the expected interactions of determining mechanisms.
Many of the publicly available forecasts for more complex systems (concerning e.g. electricity production, disposal, collection of waste etc.), as provided by governmental bodies or industry organisations, are based on modelling. Models can be divided in bottom-up engineering-economic models (such as European Commission 1995a, Mattsson 1997, Mattsson & Wene 1997, Stein & Wagner 1999, Kram et al. 2001, Gielen & Moriguchi 2001), and top-down macroeconomic and general equilibrium models such as those used by IEA (2000b). Jochem (1999) delivers a critique of engineering-economic models compared to top-down models and conclude: “Top-down [modelling] communities have far too little knowledge imbedded with regard to technological change, saturation in high income economies, and structural change. Engineering-economic modellers, on the other side, have little to say on the influence of rebound effects or income effects, which may be very important in specific target groups (e.g. private households),” and recommend a better integration of the communities of engineering-economic and top-down modellers. Walter-Jørgensen (1999) presents an interesting combination of technical analysis, farm-level economic analysis and use of a general equilibrium model in a study on phasing out of pesticide use.
Complex models as the ones mentioned above, cannot be explained in few words, and we therefore give only a few examples of more domain-specific models and applications:
Example: Modelling the energy use and emissions from transport The energy consumption for transport per kg good may change over time depending on changes in:
- modal choice (ship, rail, truck, aeroplane),
- transport distances,
- vehicle sizes,
- capacity utilisation,
- traffic conditions,
- combustion efficiency,
- education and maintenance.
Changes in emissions depend on all of the above plus changes in fuel composition and emission control. The interdependence of the different variables can be expressed in the form of equations, and a time series can be determined for the determining variables. This constitutes a model. Several such models of transport systems exist, e.g. as a result of the EU COST 319 action.
The following example shows a very simple, qualitative form of modelling, with only a few variables.
Example (from de Beer 1998):
Potential technologies for steel casting (identified through participatory methods, see section 6.1.6) are scored in a matrix according to their current stage of development and their degree of technical innovation compared to the currently applied technology.
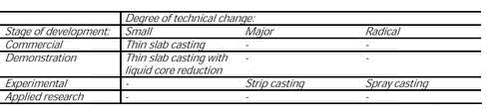
Click on the picture to see the html-version of: Degree of technical change
Supplemented by a consideration of costs and benefits (strip casting having the largest potential for energy savings), this modelling leads to placing the most probable future technologies (thin slab casting and strip casting) on a time series.
Limitations of modelling in forecasting Since modelling include the combined effects of several developments, and is not based on historical data alone, it can more readily be used for forecasts with a longer time horizon.
Still, depending on the number of variables and the degree of uncertainty included in the modelling, it may result in oversimplification of the future. Thus, in studies that deal with less predictable processes and more complex systems, where the driving forces can work in many directions, modelling should be supplemented by participatory methods (see section 6.1.6), and for forecasts with a longer time horizon, several scenarios should be applied (see section 6.1.8).
Modelling will typically require the involvement of technical experts both for the identification of relevant mechanisms, their interactions, and their probabilities of occurrence. It may thus be too sophisticated for more simple situations (medium or short-term forecasts for smaller, specific areas, where no radical or untypical developments are expected).
6.7 Participatory forecasting
Participatory forecasting methods use the insight and opinions of experts and stakeholders to derive statements on the possibility and/or probability of future events and mechanisms and their interaction.
The insight and opinions of experts and stakeholders are derived:
- from scanning of published information,
- by questionnaire polling,
- from one-to-one interviews,
- from panels, in which different opinions are confronted.
These sources may also be used in combination.
Scanning of published information is the most neutral of the methods, but its scope is limited to the issues on which published information is available, and it does not allow interaction between the source and the inquirer. Questionnaire polling has the advantage of involving a larger and possibly representative group of people. One-to-one interviews provide more flexibility in soliciting arguments for the answers given, in searching for biases and contradictions, and in following unexpected lines of inquiry arising from the interview situation. Panel methods, in which the opinions of the participants are confronted, may be used both with an exploratory orientation, to stimulate creativity and divergence, and (more commonly) with a consensus-orientation, seeking to reach some degree of consensus among the panellists.
When selecting sources or participants for polling, interviews, or panels, more or less weight may be placed on involvement of:
- a representative group (typically the overall concern in questionnaire polling),
- different stakeholders (important when aiming at consensus),
- sources of interesting and extreme positions (important when the focus is more exploratory).
All participatory methods have an element of subjectivity, which may be countered in different ways:
- The selection of sources or participants may be biased, excluding certain stakeholders or extreme positions. This may be countered by letting the selection be done by one or more "neutral" third parties. It should be avoided that participants are excluded because of lack of resources or access to specific forms of communication (e.g. access to electronic mail or telephone services).
- Wording of questionnaires or the presentation of issues to interviewees or panellists can pre-determine the results. This may be countered by starting with more open questions, pre-testing the questions on a critical panel,and by including specific test-questions that address the same issue from a different angle. It should be avoided that participants are forced to answer questions that they do not feel qualified to answer.
- A human tendency to stay within traditional patterns of thought may be countered by specific mental techniques to stimulate new thoughts among participants.
- There is a tendency that participants answer questions in the way that they expect the interviewer to desire. Ensuring anonymity of participants may enhance their willingness to give controversial or extreme answers to questions. When working with panels, this may be further stimulated by group facilitation techniques such as simulations and games.
- Information on how others have answered the same questions, and possibly also their arguments for such answers, may stimulate revised answers or counter-arguments, especially when anonymity is ensured. Such repeated questioning with feedback and anonymity has become known as the Delphi-technique, which is very widely used e.g. for technological foresight programmes (Gupta & Clarke 1996, Georghiou 1996).
Forecasts resulting from participatory techniques are quite often available in published form.
Limitations of participatory methods Participatory methods are especially relevant when dealing with controversial or complex aspects of a life cycle study. Several opinions may be heard, including more extreme positions, which may be disregarded by more analytical methods such as modelling. When stakeholders are involved, participatory methods may furthermore increase the probability of acceptance of the results from a life cycle study and thus speed up the following implementation.
However, because of their subjective elements, participatory methods may still be seen as unacceptable both by those who feel unable to influence the result and by those who see the participatory process as endangering to their established power.
Also, participatory methods are quite time consuming and difficult to apply, and will therefore be too sophisticated for more simple situations (medium or short-term forecasts for smaller, specific areas, where no radical or untypical developments are expected).
6.8 Exploratory and normative forecasting
For processes upon which the decision-maker has a large degree of (potential) influence, and especially in the context of product development, it may be more interesting to examine how the future could be (using exploratory methods), or how it should be (using normative methods), than how it is likely to be (using analytical methods, such as modelling).
Exploratory methods concentrate on structuring possible futures, typically using qualitative descriptions. Exploratory methods combine analytic techniques that branches a broad topic or development into increasingly smaller subtopics or consequences, and imaginative techniques aimed at filling all gaps in the analytical structure. In this way, the full field of possibilities is identified and structured, providing a multitude of combinations and permutations as a starting point for e.g. product development. This large number of possibilities may afterwards be reduced according to economic, technical, and strategic criteria, summarised as e.g. breakthrough potential and importance to the decision-maker.
An example of an exploratory method for use in product development is TRIZ, a commercial method that combines analogy and morphological analysis (Kowalick 1997, Arciszewski & Zlotin 1998). TRIZ is based on systematic analysis of patents from which a number of principles of innovation and "laws of evolution of engineering systems" were derived (Altshuller 1984). Morphological analysis is used to identify the essential functions of the investigated product and the possible solutions for specific functions. These possible solutions are then combined with the innovation principles and the above statements or "laws" to point out the most relevant future solution. In TRIZ, functions and methods have been collected in a database. The TRIZ database is usually used in product development but it can also be used for technological forecasting by simulating the product development.
Normative (or goal-oriented) forecasting investigates how we want the future to be and how to obtain this goal. In contrast to e.g. modelling, which investigates possibilities and probabilities and generally moves forward into the future in terms of forces at play, normative forecasting states objectives that may be substantially discontinuous with the trends at play, then moves backwards to the present to identify the necessary steps for reaching the objectives. Besides this particularity, any of the other forecasting methods may be applied also in normative forecasting. Normative forecasting is at the heart of organisational planning. It allows an organisation to orchestrate and target its resources to achieve a goal. The statement of the goal itself must be realistic and take into account present and future resources and contexts. A crucial part of a normative forecast is the detailed analysis, which reveals the specific steps that must be taken at specific times.
Exploratory and normative forecasting require a detailed knowledge of the involved organisation and technical field. It must therefore be performed in close co-operation with knowledgeable people in the organisation. The involvement of the decision-makers is essential in the criteria-setting stage of exploratory forecasting and the goal-setting stage of normative forecasting.
Technology roadmaps are one result of exploratory normative forecasting. Examples are Eisenhauer et al. (1997), Semiconductor Industry Association (1999).
Limitations of exploratory and normative forecasting Exploratory and normative forecasting are only relevant methods for processes upon which the decision-maker has a large degree of (potential) influence, so that the necessary steps can be taken to reach the forecasted (selected) goal. It may be tempting to place unrealistic confidence in the potential influence of the decision-maker, and to place to little emphasis upon outside influences. Exploratory methods may yield an overabundance of possibilities, which makes it difficult to identify which of the possibilities are the most relevant.
6.9 Scenario forecasting
For long-term forecasts in complex situations where many interdependent forces are at play, it is unlikely that a specific forecast can be identified as the single “most likely” description of the future. Instead, scenario methods aim at presenting a broad range of plausible outcomes (scenarios), which can serve as a basis for robust conclusions that are viable over the wide range of possible futures.
The term “scenario” comes from the dramatic arts, where a scenario refers to an outline of the plot. In forecasting, a scenario is an integrated, coherent, and consistent narrative description of a plausible future situation, often including a description of the development from the present to the future to focus attention on causal processes and decision points.
Often scenarios are based on modelling, displaying the conditions of important variables over time, thereby giving a quantitative underpinning of the narrative description. The nature of evolutionary paths are especially relevant when scenarios are used directly in decision making, since decisions can deflect those paths. However, a scenario does not have to be based on a model, but can be a simple description of a situation.
One scenario usually represents a surprise-free continuation of the present forces at play. Other scenarios are typically based on extreme optimistic and/or extreme pessimistic developments in one or more of the particularly important cause-effect mechanisms (typically technological, political, economical or sociological mechanisms). In general, three to six scenarios are sufficient to capture the range of future possibilities.
Scenario methods are widespread and many good examples have been published (see e.g. UN 1990, Gallopin et al. 1997, WBCSD 1998, Glenn & Gordon 1998). General scenarios for use in life cycle assessments may be derived from such published sources, e.g. the model-based energy scenarios of the EU (European Commission 1996). A scenario methodology for use in product design, with both participatory and normative elements, is described by Manzini & Jégou (2000), see also Partidário & Vergragt (2000).
When there are no resources to produce case-specific scenarios, default scenarios may be applied instead. Three scenarios are described below, which represent three extreme perspectives. These three perspectives are commonly used for scenario building (see e.g. the FROG, GEOpolicy, and Jazz scenarios in WBCSD 1998). A theoretical foundation for the three perspectives is provided by three active archetypes of the socio-cultural viability theory (Thompson et al. 1990, Hofstetter 1998): the individualist, the hierarchist, and the egalitarian archetype.
For system delimitation, the important difference between the three perspectives concerns the degree of market regulation and the acceptability of environmentally induced change, see table 6.3:
- The individualist perspective calls for solutions based on free market economy, implying few regulations on competition and a general growth in production, which seeks to take environment into account through innovation and integration into the market mechanisms.
- The hierarchist perspective calls for solutions based on globally coordinated regulation and controlled growth that takes into account environmental externalities in the decision-making.
- The egalitarian perspective calls for solutions based on local regulation that radically change patterns of production and consumption to a sustainable level.
The consequence for system delimitation in LCA is summarised in table 6.3 and an example of how this influences the choice of electricity scenarios in Europe is provided below.
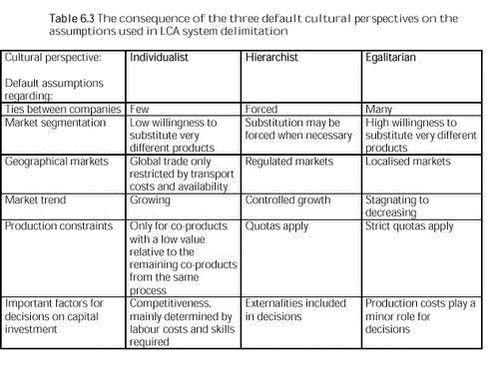 Click on the picture to see the html-version of: Table 6.3
Example: Supply of additional European electricity in the three default cultural perspectives In the individualist scenario, an additional demand for electricity will be supplied from the free market, which will be a growing, deregulated European market (only restricted by the physical limits for transmission), where the transmission capacity has been expanded to allow all producers to compete on equal terms. In this scenario, the highly competitive fossil fuels will continue to be the main source of additional power. Emission quotas do not play any significant role in restricting the use of coal, but the high capital requirements of coal-based technology may allow gas-based technology to gain a considerable market share. Innovation will mainly be driven by an interest in decreasing production costs through more efficient combustion (e.g. in fuel cells). In the hierarchist scenario, the market is regulated to include environmental externalities in the decision-making, which strives for an optimal balance between societal costs and benefits. This implies the use of tradable emission permits or emission taxes. An additional demand for electricity will be supplied by that power plant which in the given situation has the lowest production costs, taking into account the environmental externalities as translated through taxes and permits. This will place wind power very favourably, as long as acceptable solutions can be found to its localisation. The resulting electricity scenario is a mix of wind power with local biomass and regional natural gas as stabilising technologies. In the egalitarian scenario, the electricity demand will be stagnating due to a mix of increased efficiency and savings in consumption. Transmission capacity will be limited, as each region relies on its own production capacity. Nuclear power and fossil fuels has been phased out, leaving the electricity to be supplied by combustion of biomass and waste, hydro-, wind and solar sources. A certain loss of supply stability will be accepted. Since production costs play only a minor role for decision-making, a change in demand for electricity will affect the local supplier with the least environmentally benign technology, which in most cases will be the plants based on biomass combustion, although hydro-power facilities may also be affected in mountain regions.
Limitations of scenario methods Scenario forecasting may be unnecessarily sophisticated for medium or short-term forecasts and more specific, uncomplicated situations.
6.10 Consistency check
Within the same life cycle study, different forecasting methods may be appropriate for different parts of the product systems. This is not in itself an inconsistency, as long as the choice of method is justified and the specific assumptions used in the different methods are not inconsistent.
Even when the same method is applied throughout a study, it should be checked that assumptions and results are used in a consistent manner.
6.11 Combining different forecasting methods
Applying more than one forecasting method can be a way of validating the assumptions and/or outcome of each individually applied method. In particular:
- For an extrapolation, the outcome of modelling may be used to validate the relationship between different trends used, and the identification of which trends are determining and directly related to the time axis.
- An extrapolation or model may be validated by participatory methods (e.g. asking experts), which may also provide reasons that the extrapolation or model should be adjusted, e.g. due to expected legislation, economical changes or other initiatives that might affect the extrapolated trend or the modelled relations.
- Results of participatory methods are often more normative (what the future should be) than analytic (what the future is likely to be). Modelling may give a more analytical perspective on a result from participatory methods.
The outcome of one forecasting method may be used as input in other methods. In particular:
- As an input to modelling, an extrapolation may be used as one of more basic mechanisms or equations in a model, and participatory methods may provide information on causal relations and probabilities of events and mechanisms, and interaction between mechanisms.
- In extrapolation and modelling, the insights from exploratory methods may be used to ensure that all important aspects have been taken into account.
- In participatory and scenario methods, an extrapolation can be used as a surprise-free base-line forecast ("suppose things keep going as they have in the past ...") to be modified.
- In participatory methods, the results of practically all other forecasting methods may be used when designing topics and questions, or directly for the participants as background information for questions or as a common input to which they can relate.
- Normative forecasting may apply both the methods and results of any of the other forecasting methods. Especially modelling techniques may be useful during the "backtracking" step, but even extrapolations can be applied “in reverse”.
- In scenario methods, results from modelling, participatory and normative methods may be used as (part of) one or more scenarios.
| Front page | | Contents | | Previous | | Next | | Top |
|